Computer-aided experimental identification of new solvents for CO2 absorption
SSRN Electronic Journal(2022)
摘要
Molecular simulations and chemoinformatics are used quite extensively to identify new adsorbents for CO2. The use of those digital tools is far less common in the search for new solvents (absorption) to capture CO2. This is primarily related to the complexity of the absorption process, mainly due to its reactive nature. This paper highlights and builds on several recent significant advances in computer-aided experimental developments in the field of absorption. First, we focus on physical solvents. We previously developed and experimentally validated a machine-learning tool to predict the absorption capacity of CO2 as well as its selectivity with respect to other gases (H2, N2, CO, H2, and H2S). In this paper we investigate whether multi-task learning (MTL), a technique that aims to improve machine learning efficacy by simultaneously modelling several properties, can improve the model performances. The accuracy of both models, with and without MTL, is, however, comparable. Second, we study the impact of the addition of a physical co-solvent to an aqueous amine solvent on the CO2 absorption rate. Physical co-solvents with a specific value lower than water are expected to reduce the solvent regeneration energy. A recently developed and validated molecular simulations-based tool to predict CO2 absorption rates in aqueous tertiary amines is applied to solvents of aqueous tertiary amines with physical co-solvents. A comparison with new experimental data is made. Both the model and the experimental data predict a lower CO2 absorption rate in the presence of most physical co-solvents studied.
更多查看译文
AI 理解论文
溯源树
样例
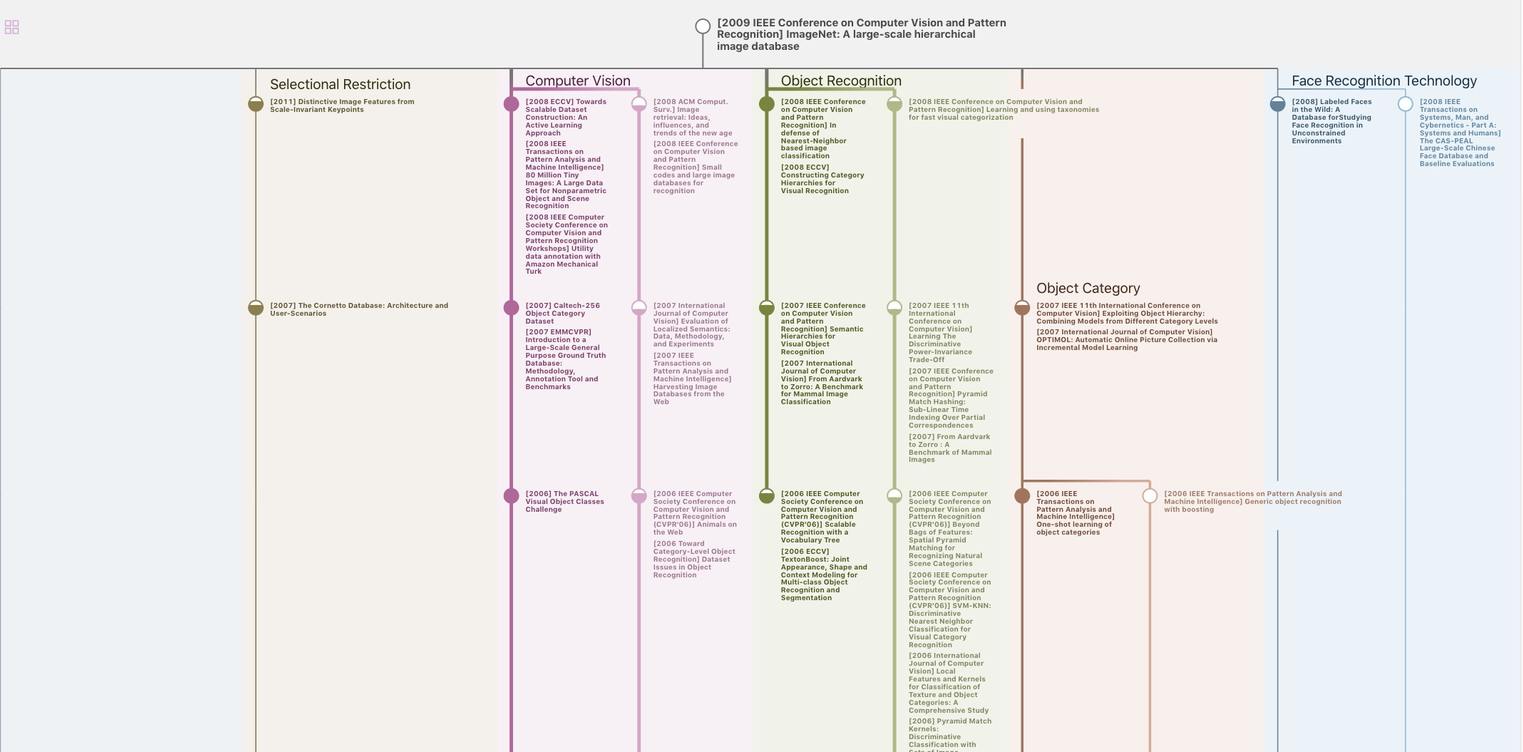
生成溯源树,研究论文发展脉络
Chat Paper
正在生成论文摘要