An Encoder-Decoder Model with Interpretable Spatio-Temporal Component for Soil Temperature Prediction
Authorea (Authorea)(2023)
摘要
Soil temperature (ST) is a crucial land-surface parameter and accurate, interpretable ST predictions are essential for Earth system science applications. While deep learning methods have shown excellent performance in ST prediction, they are often referred to as “black box optimizers”, making it difficult to extract physical knowledge and gain interpretability. To address this issue, we developed the Encoder-Decoder Model with Interpretable Spatial-Temporal Component (ISDNM) to improve predictive accuracy and provide spatial-temporal interpretation of ST. The ISDNM model combines a CNN-encoder-decoder and LSTM-encoder-decoder to enhance the representation of spatial-temporal features and applies linear regression and UMAP to provide interpretable spatial-temporal insights into ST. The ISDNM outperforms traditional deep learning models such as Convolutional Neural Network, Long Short-term Memory, and Convolutional LSTM, making it a valuable tool to improve our understanding of ST’s spatiotemporal characteristics.
更多查看译文
关键词
soil temperature,encoder-decoder,spatio-temporal
AI 理解论文
溯源树
样例
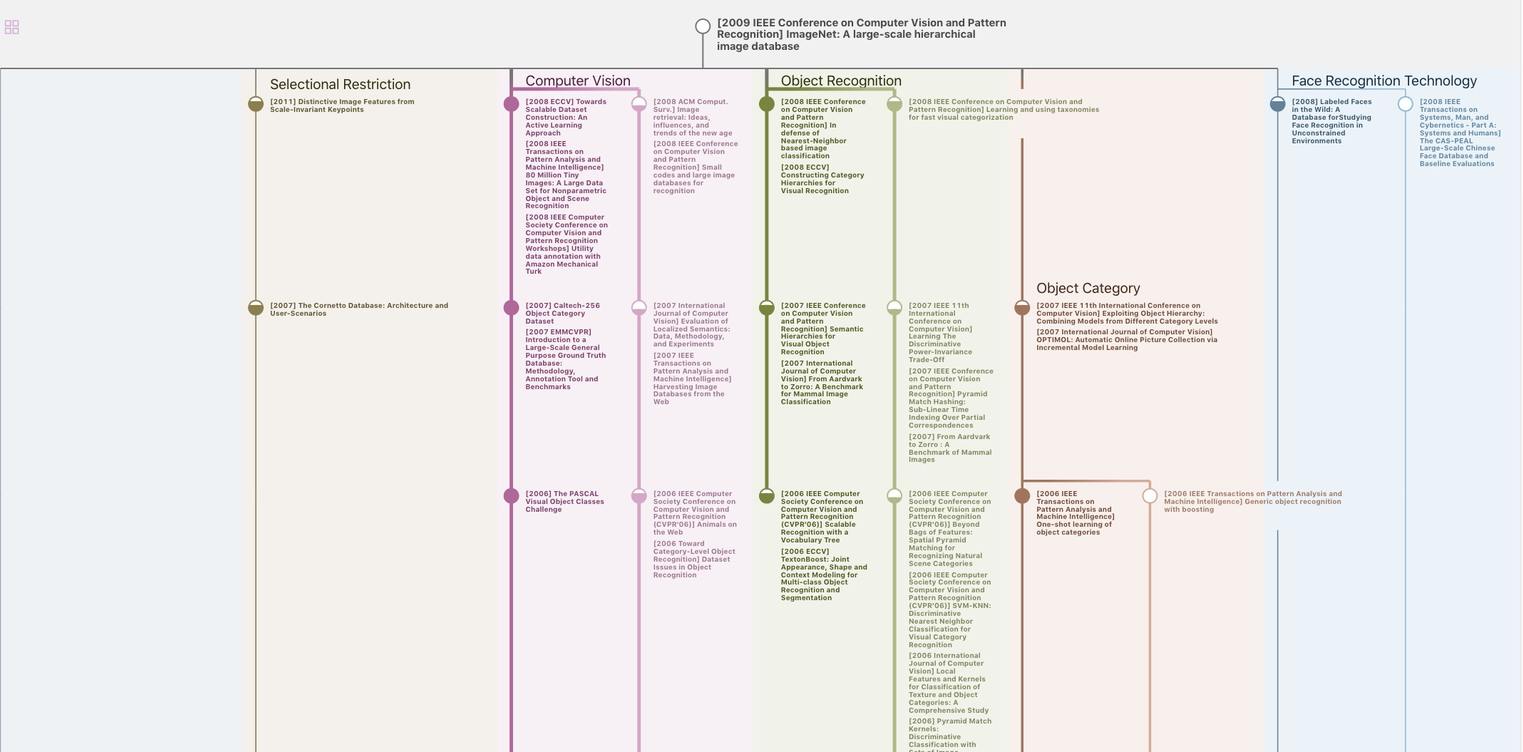
生成溯源树,研究论文发展脉络
Chat Paper
正在生成论文摘要