Comparison of machine learning algorithms for predicting hospital readmissions and worsening heart failure events in patients with heart failure with reduced ejection fraction: A modeling study (Preprint)
JMIR formative research(2022)
摘要
BACKGROUND Heart failure (HF) is highly prevalent in the United States. Approximately one-third to one-half of HF cases are categorized as heart failure with reduced ejection fraction (HFrEF). Patients with HFrEF are at risk of worsening heart failure, have a high risk of adverse outcomes, and experience higher healthcare utilization and costs. Therefore, it is crucial to identify patients who are at high risk of subsequent events following HF hospitalization among patients with HFrEF. OBJECTIVE Machine learning (ML) has been previously applied to predict HF-related outcomes. The objective of this study was to compare different ML prediction models and feature construction methods to predict 30-, 90-, and 365-day hospital readmissions and worsening heart failure events (WHFEs). METHODS The study utilized the Veradigm PINNACLE outpatient registry® linked to Symphony Health’s Integrated Dataverse data from July 1, 2013 to September 30, 2017. Adults with a confirmed diagnosis of HFrEF and a HF-related hospitalization were included. WHFEs were defined as HF-related hospitalizations or outpatient intravenous diuretic use within one year following the first HF hospitalization. We utilized different approaches to construct ML features from clinical codes: (1) Frequencies of Clinical Classification Software (CCS) categories; (2) Bidirectional Encoder Representations from Transformers (BERT) trained with CCS sequences (BERT + CCS); (3) BERT trained on raw clinical codes (BERT + raw); and (4) pre-specified features based on clinical knowledge. Multilayer perceptron neural network (MLP NN), eXtreme Gradient Boosting (XGBoost), random forest, and logistic regression prediction models were applied and compared. RESULTS A total of 30,687 adult patients with HFrEF were included in the analysis; 11.4% of adults experienced a hospital readmission within 30 days of their first HF hospitalization and nearly half (42.8%) of patients experienced at least one WHFE within one year following HF hospitalization. The prediction models and feature combinations with the best area under the receiver operating characteristic curve (AUC) for each outcome were: XGBoost with CCS frequency (0.595) for 30-day readmission; random forest with CCS frequency (0.630) for 90-day readmission; XGBoost with CCS frequency (0.649) for 365-day readmission; and XGBoost with CCS frequency (0.640) for WHFEs. Our ML models could discriminate readmission and WHFE among HFrEF patients. Our model performance was mediocre, especially for the 30-day readmission events, most likely due to limitations of the data including an imbalance between positive and negative cases and high missing rates of many clinical variables and outcome definitions. CONCLUSIONS We predicted readmissions and WHFEs following HF hospitalizations in patients with HFrEF. Features identified by data-driven approaches may be comparable to those identified by clinical domain knowledge. Future work may be warranted to validate and further improve the models using more longitudinal electronic health records which are more complete, comprehensive, and have a longer follow-up time. CLINICALTRIAL
更多查看译文
关键词
hospital readmissions,worsening heart failure events,heart failure,machine learning algorithms
AI 理解论文
溯源树
样例
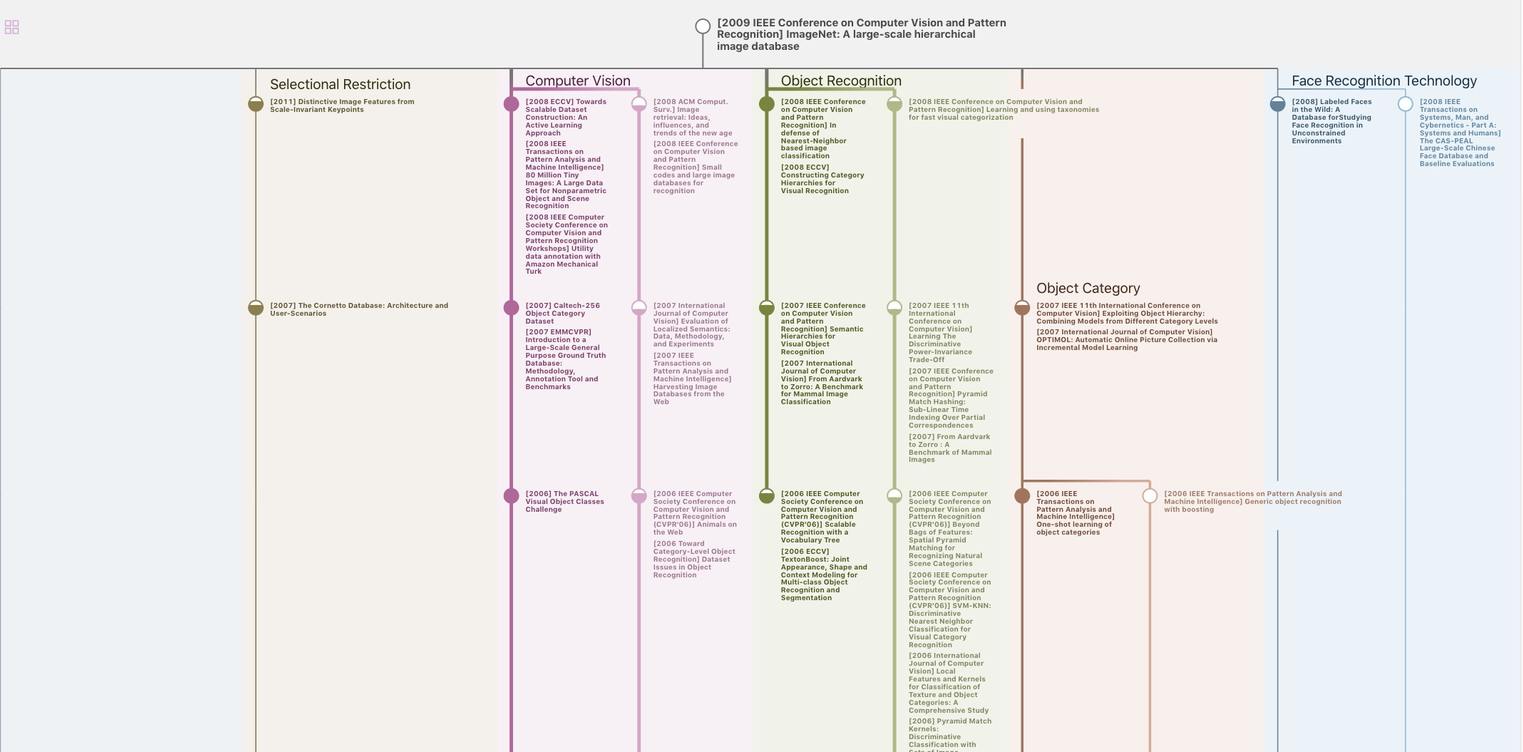
生成溯源树,研究论文发展脉络
Chat Paper
正在生成论文摘要