Dual attention-based multi-step ahead prediction enhancement for monitoring systems in industrial processes
SSRN Electronic Journal(2023)
摘要
In industrial processes, the ability to predict future steps is essential as it offers long-term insights, benefiting strategic decision-making. However, traditional sequence-to-sequence models designed to predict dynamic behaviors suffer from accumulating errors during recurrent predictions which use previous outputs as inputs for the next time step. In this article, we propose a dual attention-based encoder–decoder framework, specifically designed to enhance multi-step ahead predictions in industrial processes. The dual attention model strategically minimizes the error accumulation of output sequence by leveraging a temporal attention mechanism, which focuses on relevant time-steps in the input sequence, and a supervised attention mechanism that assigns different weights to output sequence errors during training. The supervised attention method, in particular, provides a significant improvement by focusing on minimizing the error of earlier steps during backpropagation using predefined attention weights, resulting in enhanced overall multistep prediction performance. Experiments on real-world industrial datasets demonstrate that our approach outperforms baseline models, specifically simple sequence-to-sequence and single attention-based sequence-to-sequence models. In fact, our dual attention framework consistently surpasses single attention models, currently regarded as state-of-the-art, at all prediction stages. The suggested approach has potential applications in the field of process monitoring and model predictive control.
更多查看译文
关键词
Multi-step ahead prediction,Sequence-to-sequence learning,Dual attention mechanism,Cumulative error minimization,Real-world industrial process
AI 理解论文
溯源树
样例
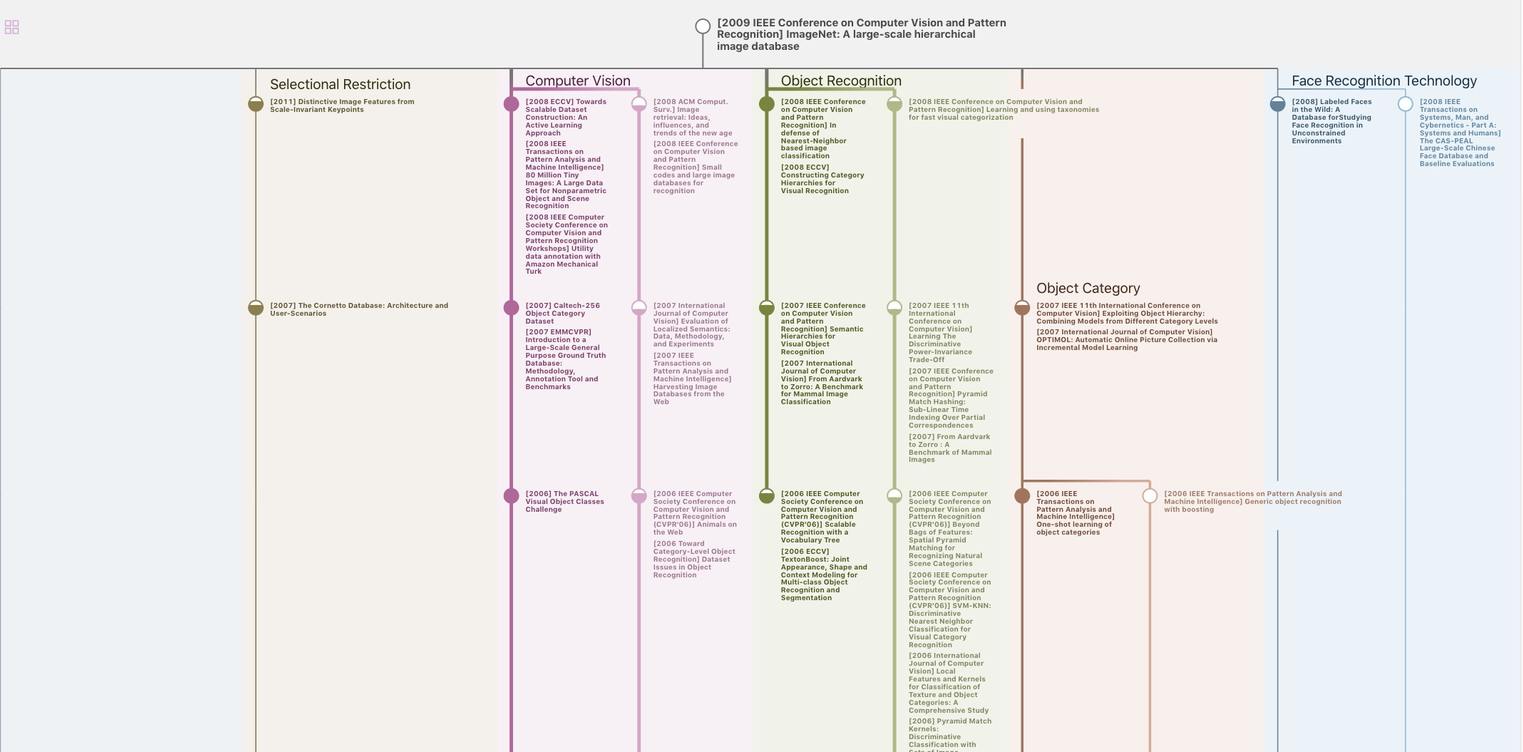
生成溯源树,研究论文发展脉络
Chat Paper
正在生成论文摘要