Towards a new surrogate model for predicting short-term NOx-O3 effects from aviation using Gaussian processes
crossref(2023)
摘要
<p>While efforts have been made to curb CO<sub>2</sub> emissions from aviation, the more uncertain non-CO<sub>2</sub> effects that contribute about two-thirds to the warming in terms of radiative forcing (RF), still require attention. The most important non-CO<sub>2</sub> effects include persistent line-shaped contrails, contrail-induced cirrus clouds and nitrogen oxide (NO<sub>x</sub>) emissions that alter the ozone (O<sub>3</sub>) and methane (CH<sub>4</sub>) concentrations, both of which are greenhouse gases, and the emission of water vapour (H<sub>2</sub>O). The climate impact of these non-CO<sub>2</sub> effects depends on emission location and prevailing weather situation; thus, it can potentially be reduced by advantageous re-routing of flights using Climate Change Functions (CCFs), which are a measure for the climate effect of a locally confined aviation emission. CCFs are calculated using a modelling chain starting from the instantaneous RF (iRF) measured at the tropopause that results from aviation emissions. However, the iRF is a product of computationally intensive chemistry-climate model (EMAC) simulations and is currently restricted to a limited number of days and only to the North Atlantic Flight Corridor. This makes it impossible to run EMAC on an operational basis for global flight planning. A step in this direction lead to a surrogate model called algorithmic Climate Change Functions (aCCFs, [1]), derived by regressing CCFs (training data) against 2 or 3 local atmospheric variables at the time of emission (features) with simple regression techniques and are applicable only in parts of the Northern hemisphere. It was found that in the specific case of O<sub>3</sub> aCCFs, which provide a reasonable first estimate for the short-term impact of aviation NO<sub>x</sub> on O<sub>3</sub> warming using temperature and geopotential as features, can be vastly improved. There is aleatoric uncertainty in the full-order model (EMAC), stemming from unknown sources (missing features) and randomness in the known features, which can introduce heteroscedasticity in the data. Deterministic surrogates (e.g. aCCFs) only predict point estimates of the conditional average, thereby providing an incomplete picture of the stochastic response. Thus, the goal of this research is to build a new surrogate model for iRF, which is achieved by :</p><p>1. Expanding the geographical coverage of iRF (training data) by running EMAC simulations in more regions (North & South America, Eurasia, Africa and Australasia) at multiple cruise flight altitudes,</p><p>2. Following an objective approach to selecting atmospheric variables (feature selection) and considering the importance of local as well as non-local effects,</p><p>3. Regressing the iRF against selected atmospheric variables using supervised machine learning techniques such as homoscedastic and heteroscedastic Gaussian process regression.</p><p>We present a new surrogate model that predicts iRF of aviation NO<sub>x</sub>-O<sub>3</sub> effects on a regular basis with confidence levels, which not only improves our scientific understanding of NO<sub>x</sub>-O<sub>3</sub> effects, but also increases the potential of global climate-optimised flight planning.</p><p><strong>References</strong></p><p>[1] van Manen, J.; Grewe, V. Algorithmic climate change functions for the use in eco-efficient flight planning. <em>Transp. Res. Part D Transp. Environ.</em> <strong>2019</strong>, <em>67</em>, 388–405.</p>
更多查看译文
AI 理解论文
溯源树
样例
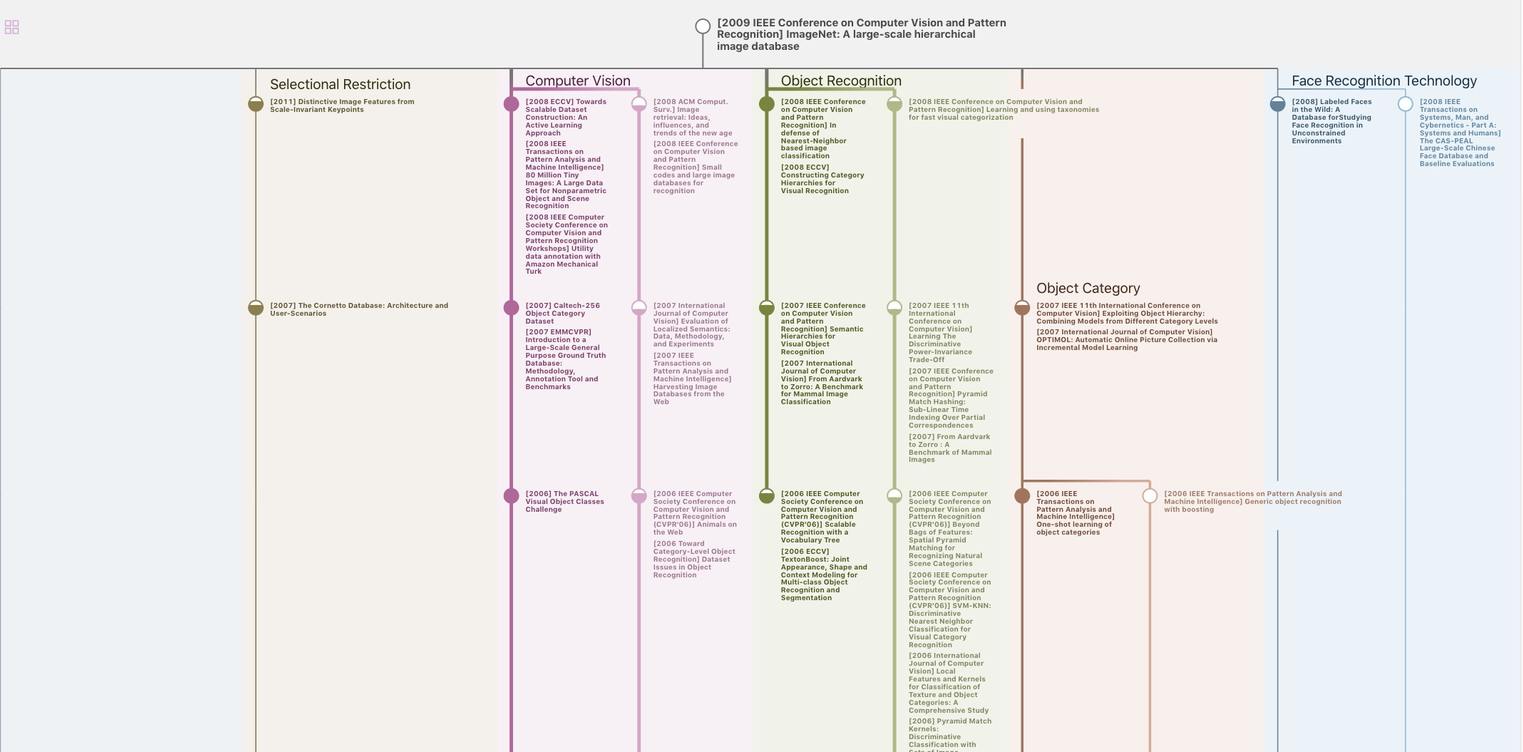
生成溯源树,研究论文发展脉络
Chat Paper
正在生成论文摘要