Multi-view Feature Learning for the Over-penalty in Adversarial Domain Adaptation
DATA INTELLIGENCE(2024)
摘要
Domain adaptation aims to transfer knowledge from the labeled source domain to an unlabeled target domain that follows a similar but different distribution. Recently, adversarial-based methods have achieved remarkable success due to the excellent performance of domain-invariant feature presentation learning. However, the adversarial methods learn the transferability at the expense of the discriminability in feature representation, leading to low generalization to the target domain. To this end, we propose a Multi-view Feature Learning method for the Over-penalty in Adversarial Domain Adaptation. Specifically, multi-view representation learning is proposed to enrich the discriminative information contained in domain-invariant feature representation, which will counter the over-penalty for discriminability in adversarial training. Besides, the class distribution in the intra-domain is proposed to replace that in the inter-domain to capture more discriminative information in the learning of transferrable features. Extensive experiments show that our method can improve the discriminability while maintaining transferability and exceeds the most advanced methods in the domain adaptation benchmark datasets.
更多查看译文
关键词
domain adaptation,adversarial learning,multi-view learning
AI 理解论文
溯源树
样例
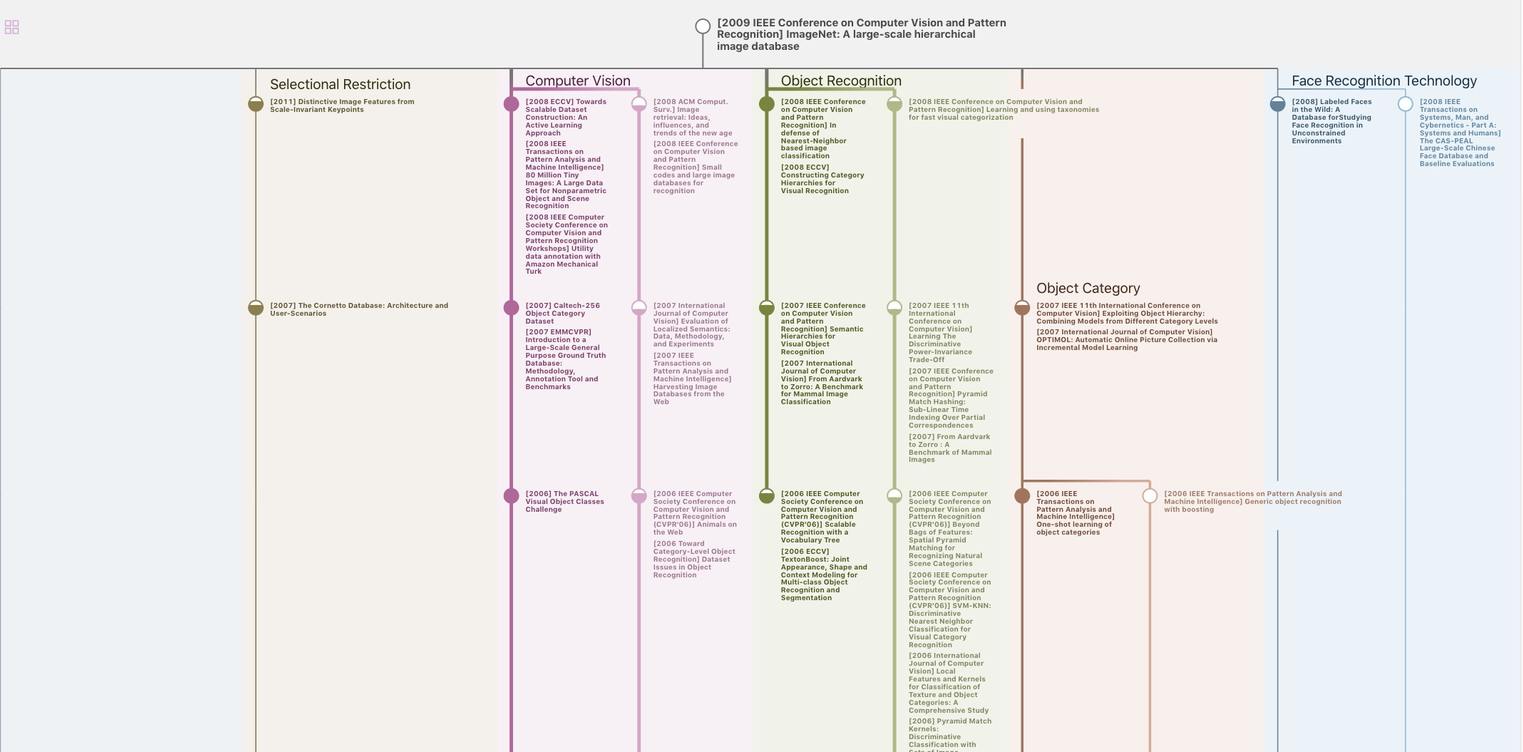
生成溯源树,研究论文发展脉络
Chat Paper
正在生成论文摘要