PKLNet: Keypoint Localization Neural Network for Touchless Palmprint Recognition Based on Edge-Aware Regression
IEEE Journal of Selected Topics in Signal Processing(2023)
摘要
The usage of touchless palmprint recognition (PPR) increases due to its acceptant interaction mode. However, the region of interest (ROI) localization at a distance is quite challenging for touchless PPR in real-world scenarios with complex backgrounds and hand poses. To address this issue, we proposed a palm keypoint localization neural network (PKLNet) that combines information on the hand region, palm boundary, and finger valley edges to achieve accurate and robust keypoint localization. First, a two-stage neural network was proposed. It effectively adopted the transformer framework to capture global relations of the palm boundary points to perform palm region segmentation and ROI keypoint coordinate regression. Second, an image synthesis-based training strategy was developed based on conventional palmprint ROI localization methods. The obtained ROI localizer (namely PalmKit) can automatically generate palm region masks and keypoint coordinates, which can significantly simplify the data annotation process and hence liberate the heavy manual labor. Finally, extensive experiments were performed on various touchless palmprint datasets. The proposed PKLNet obtained an 82.1% success rate and a 7.7 pixels median localization error in the cross-dataset test. The results demonstrate that the proposed PKLNet is robust to palm rotation, translation, and inference from complex backgrounds, ensuring the usability of the touchless PPR technique in real-world application scenarios.
更多查看译文
关键词
Biometrics,touchless palmprint recognition (PPR),region of interest (ROI) localization,keypoint coordinate regression (KCR),edge-aware loss,weakly-supervised training
AI 理解论文
溯源树
样例
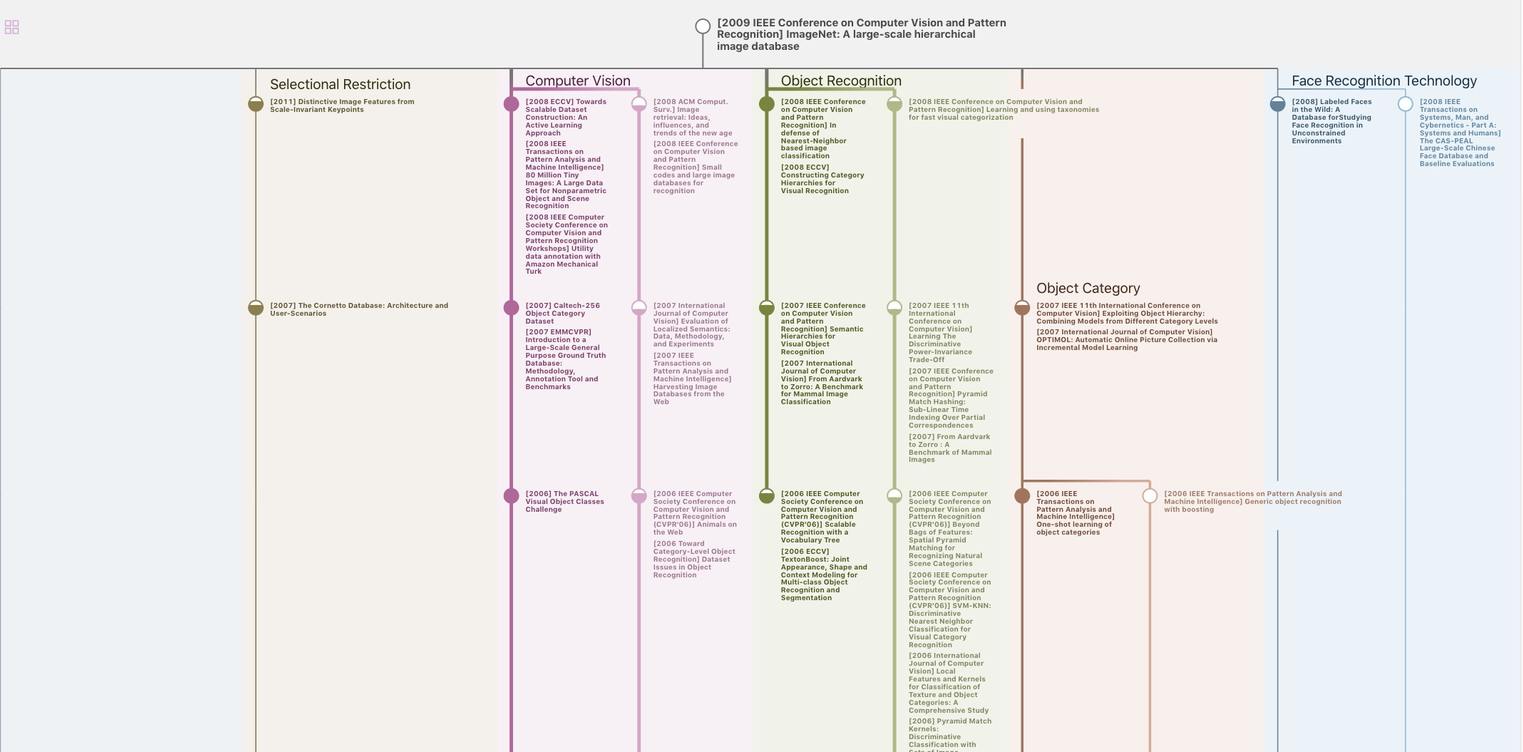
生成溯源树,研究论文发展脉络
Chat Paper
正在生成论文摘要