DailyMelt: Diffusion-based Models for Spatiotemporal Downscaling of (Ant-)arctic Surface Meltwater Maps
crossref(2023)
摘要
<p><strong>Motivation. </strong>Ice melting in Greenland and Antarctica has increasingly contributed to rising sea levels. Yet, the exact speed of melting, existence of abrupt tipping points, and in-detail links to climate change remain uncertain. Ice shelves essentially prevent the ice sheet from slipping into the ocean and better prediction of collapses is needed. Meltwater at the surface of ice shelves indicates ice shelf collapse through destabilizing ice shelves via fracturing and flexural processes (Banwell et al., 2013) and is likely impacted by a warming climate ( Kingslake et al., 2017). Maps of meltwater have been created from in-situ and remote observations, but their low and irregular spatiotemporal resolution severely limits studies (Kingslake et al., 2019).</p> <p><strong><img src="" alt="" /></strong></p> <p><strong>Research Gap.</strong> In particular, there <em>does not exist daily high-resolution (< 500m) maps of surface meltwater</em>. We propose the first daily high-resolution surface meltwater maps by developing a deep learning-based downscaling method, called <em>DailyMelt</em>, that fuses observations and simulations of varying spatiotemporal resolution, as illustrated in Fig.1. The created maps will improve understanding of the origin, transport, and controlling physical processes of surface meltwater. Moreover, they will act as unified source to improve sea level rise and meltwater predictions in climate models. </p> <p><strong>Data. </strong>To synthesize surface meltwater maps, we leverage observations from satellites (MODIS, Sen-1 SAR) which are high-resolution (500m, 10m), but have substantial temporal gaps due to repeat time and cloud coverage. We fuse them with simulations (MAR) and passive microwave observations (MEaSURE) that are daily, but low-resolution (6km, 3.125km). In a significant remote sensing effort, we have downloaded, reprojected, and regridded all products into daily observations for our study area over Greenland’s Helheim glacier. </p> <p><strong>Approach and expected results. </strong>Within deep generative vision models, diffusion-based models promise sharp and probabilistic predictions. We have implemented SRDiff (Li H. et al., 2022) and tested it on spatially downscaling external data. As a baseline model, we have implemented a statistical downscaling model that is a local hybrid physics-linear regression model (Noel et al., 2016). In our planned benchmark, we expect a baseline UNet architecture that minimizes RMSE to create blurry maps and a generative adversarial network that minimizes adversarial loss to create sharp but deterministic maps. We have started with spatial downscaling and will include temporal downscaling. </p> <p>In summary, we will create the first daily high-resolution (500m) surface meltwater maps, have introduced the first diffusion-based model for downscaling Earth sciences data, and have created the first benchmark dataset for downscaling surface meltwater maps.</p> <p> </p> <p><strong>References.</strong></p> <p>Banwell, A. F., et al. (2013), Breakup of the Larsen B Ice Shelf triggered by chain reaction drainage of supraglacial lakes, Geophys. Res. Lett., 40 </p> <p>Kingslake J, et al. (2017), Widespread movement of meltwater onto and across Antarctic ice shelves, Nature, 544(7650)</p> <p>Kingslake J., et al. (2019), Antarctic Surface Hydrology and Ice Shelf Stability Workshop report, US Antarctic Program Data Center</p> <p>Li H., et al. (2022), SRDiff: Single image super-resolution with diffusion probabilistic models, Neurocomputing, 479</p> <p>Noël, B., et al. (2016), A daily, 1 km resolution data set of downscaled Greenland ice sheet surface mass balance (1958–2015), The Cryosphere, 10</p>
更多查看译文
AI 理解论文
溯源树
样例
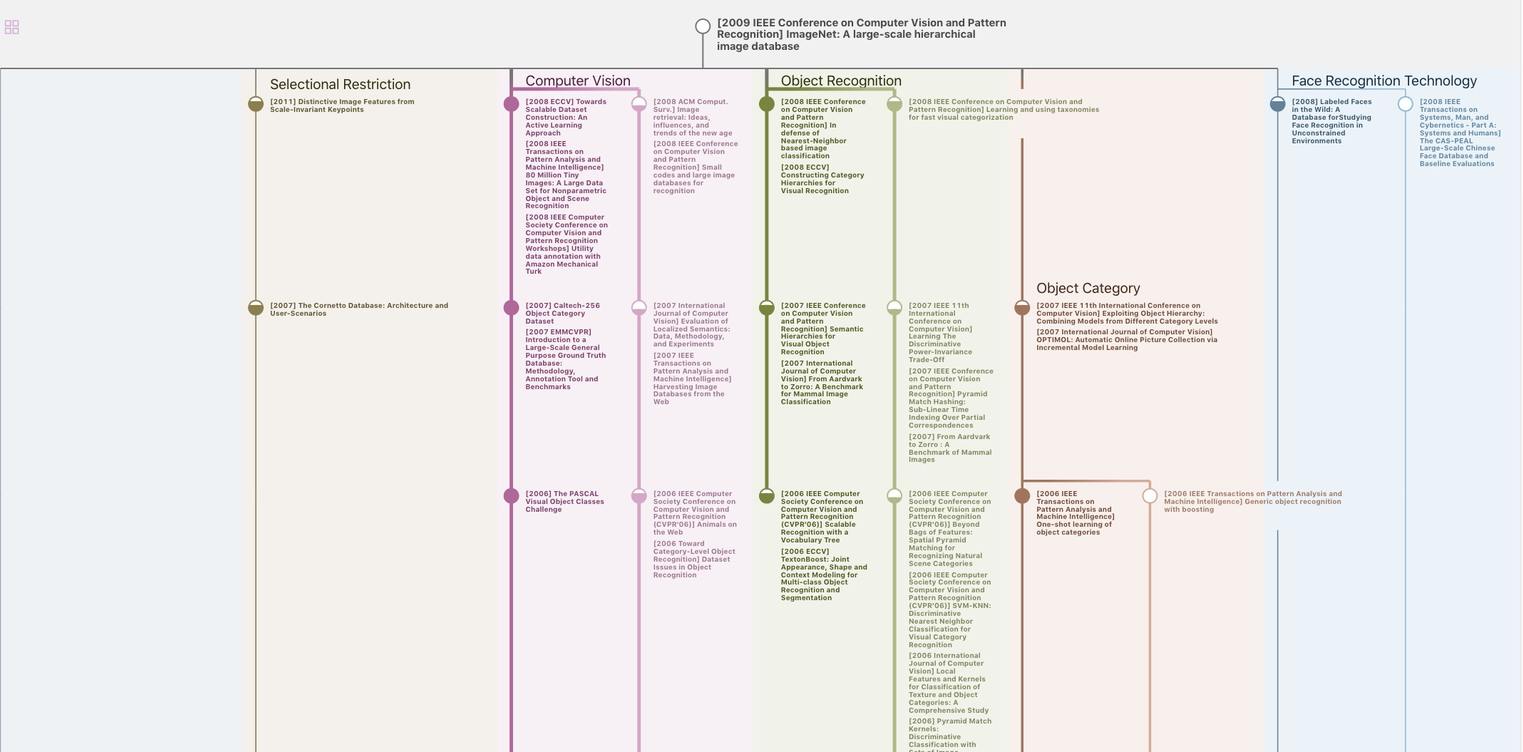
生成溯源树,研究论文发展脉络
Chat Paper
正在生成论文摘要