Learning-Observer-Based Adaptive Tracking Control of Multiagent Systems Using Compensation Mechanism.
IEEE Transactions on Artificial Intelligence(2024)
摘要
This article focuses on the adaptive consensus tracking problem for nonlinear multiagent systems with unmeasurable state and input saturation. For unmeasurable state and nonlinearities, a learning-observer inspired by the idea of extended state observer and the learning ability of neural network are established to observe the reconstructed system. In view of input saturation, a nonlinear auxiliary system with the same order is constructed to obtain the compensation signals. Different from the previous auxiliary system, the proposed method simultaneously compensates the change of nonlinearity caused by input saturation. Subsequently, a consensus tracking error is designed to ensure that the follower tracks the neighbor compensated output. Moreover, an adaptive consensus tracking control method based on learning-observer and compensation mechanism is proposed under the backstepping framework. The stability analysis is given by virtue of the Lyapunov theory. Finally, the effectiveness and superiority of the proposed control algorithm are verified through the simulation of unmanned air vehicles with one virtual leader and four followers.
更多查看译文
关键词
Adaptive neural network (NN) consensus tracking control,compensation mechanism,learning-observer,multiagent systems (MASs),nonlinear auxiliary system
AI 理解论文
溯源树
样例
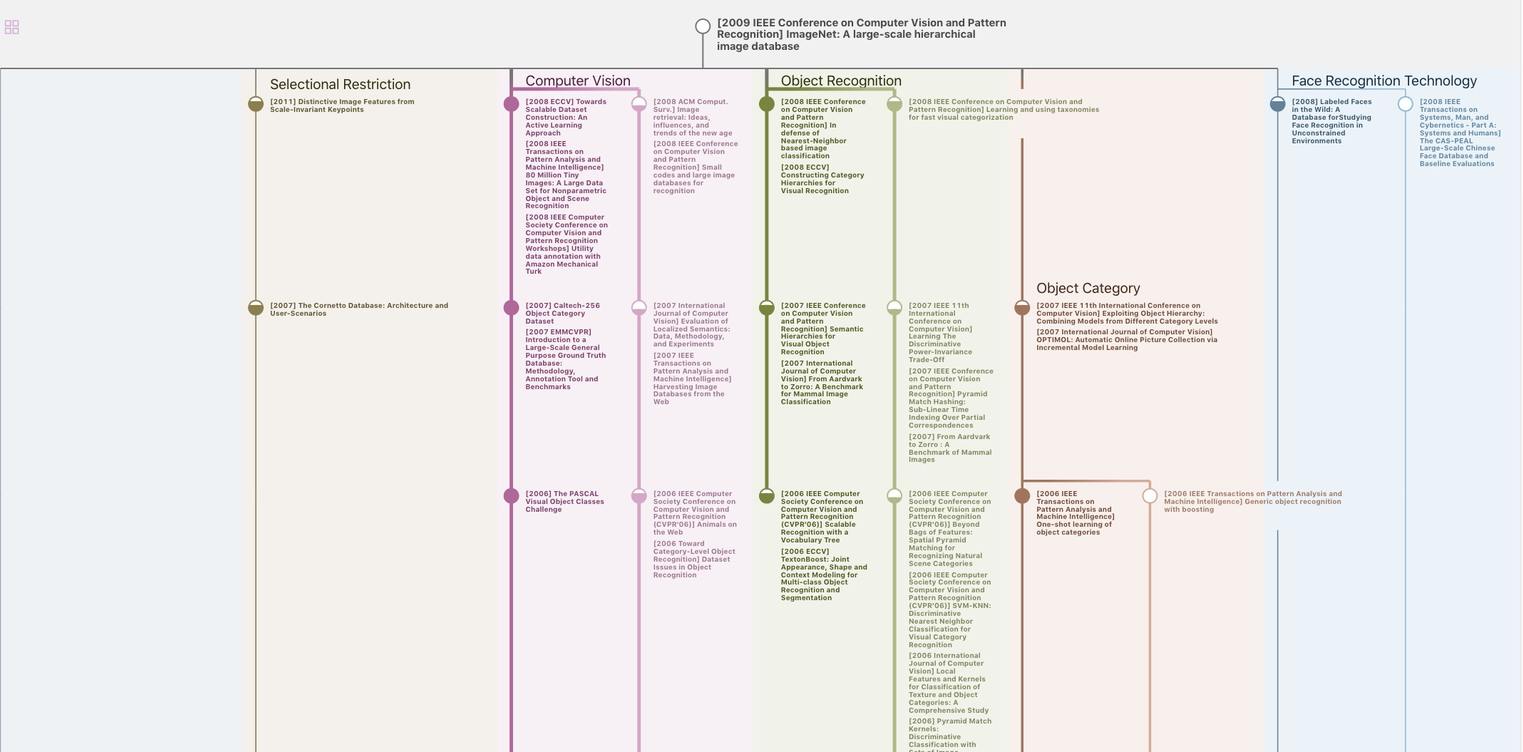
生成溯源树,研究论文发展脉络
Chat Paper
正在生成论文摘要