A Prototype Network Enhanced Relation Semantic Representation for Few-shot Relation Extraction
Human-Centric Intelligent Systems(2022)
Abstract
Few-shot relation extraction is one of the current research focuses. The key to this research is to fully extract the relation semantic information through very little training data. Intuitively, raising the relation semantics awareness in sentences can improve the efficiency of the model to extract relation features to alleviate the overfitting problem in few-shot learning. Therefore, we propose an enhanced relation semantic feature model based on prototype network to extract relations from few-shot texts. Firstly, we design a multi-level embedding encoder with position information and Transformer, which uses local information in the text to enhance the relation semantics representation. Secondly, the encoded relation features are fed into the novel prototype network, which designs a method that utilizes query prototype-level attention to guide the extraction of supporting prototypes, thereby enhancing the prototypes representation to better classify the relations in query sentences. Finally, through experimental comparison and discussion, we prove and analyze the effectiveness of the proposed multi-level embedding encoder, and prototype-level attention can enhance the stability of the model. Furthermore, our model has substantial improvements over baseline methods.
MoreTranslated text
Key words
The few-shot relation extraction, The local relation information, Multi-level embedding representation, The prototype-level attention
AI Read Science
Must-Reading Tree
Example
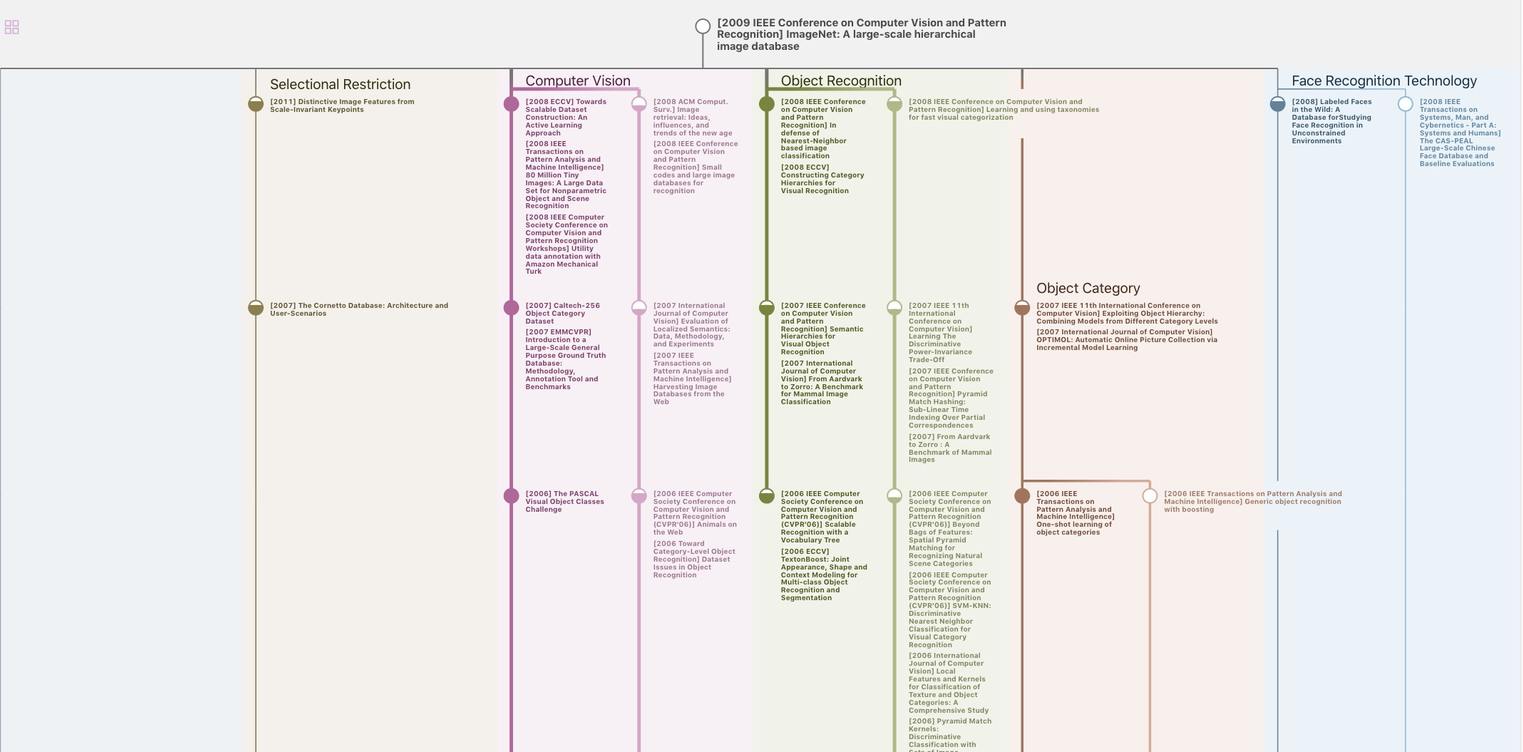
Generate MRT to find the research sequence of this paper
Chat Paper
Summary is being generated by the instructions you defined