Self-supervised deep learning for highly efficient spatial immunophenotyping
eBioMedicine(2023)
摘要
Background Efficient biomarker discovery and clinical translation depend on the fast and accurate analytical output from crucial technologies such as multiplex imaging. However, reliable cell classification often requires extensive annotations. Label-efficient strategies are urgently needed to reveal diverse cell distribution and spatial interactions in large-scale multiplex datasets.Methods This study proposed Self-supervised Learning for Antigen Detection (SANDI) for accurate cell phenotyping while mitigating the annotation burden. The model first learns intrinsic pairwise similarities in unlabelled cell images, followed by a classification step to map learnt features to cell labels using a small set of annotated references. We acquired four multiplex immunohistochemistry datasets and one imaging mass cytometry dataset, comprising 2825 to 15,258 single-cell images to train and test the model.Findings With 1% annotations (18-114 cells), SANDI achieved weighted F1-scores ranging from 0.82 to 0.98 across the five datasets, which was comparable to the fully supervised classifier trained on 1828-11,459 annotated cells (-0.002 to -0.053 of averaged weighted F1-score, Wilcoxon rank-sum test, P = 0.31). Leveraging the immune checkpoint markers stained in ovarian cancer slides, SANDI-based cell identification reveals spatial expulsion between PD1-expressing T helper cells and T regulatory cells, suggesting an interplay between PD1 expression and T regulatory cell-mediated immunosuppression.Interpretation By striking a fine balance between minimal expert guidance and the power of deep learning to learn similarity within abundant data, SANDI presents new opportunities for efficient, large-scale learning for histology multiplex imaging data.
更多查看译文
关键词
Deep learning,Self-supervised learning,Cell classification,Multiplex imaging,Multiplex immunohistochemistry,Imaging mass cytometry
AI 理解论文
溯源树
样例
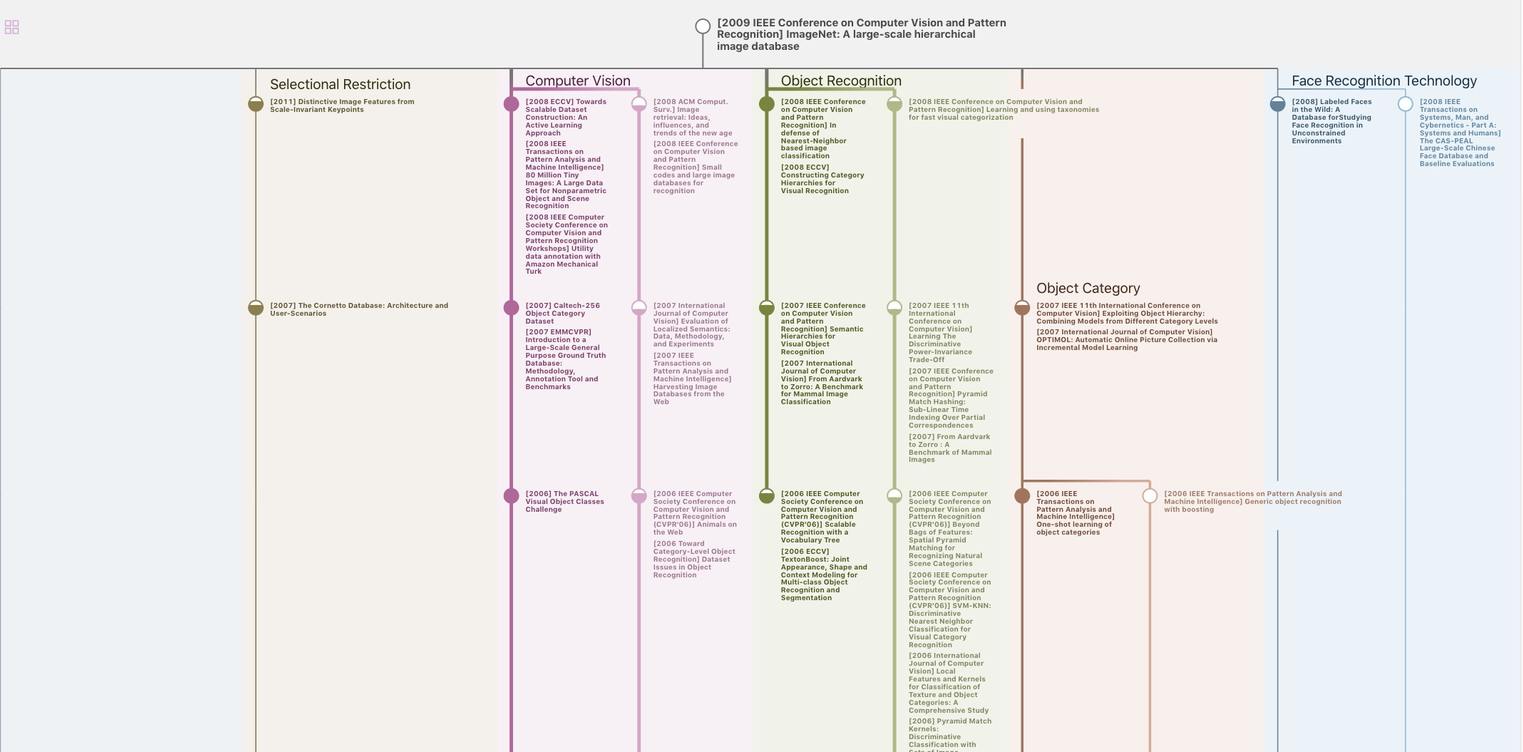
生成溯源树,研究论文发展脉络
Chat Paper
正在生成论文摘要