Small Dataset-Based Closed-Loop State of Charge Estimation for Pure Electric Construction Machinery With Large Sensor Error: A Case Study of 5-ton Loader
IEEE Transactions on Transportation Electrification(2023)
Abstract
Pure electric construction machinery (PECM) is the future development trend for construction machinery. Compared with other electric drive systems, the PECM systems are often accompanied by complex working conditions, frequent high current pulse signals, limited memory sources, and heavier sensor noise. To improve the robustness and accuracy of state of charge (SOC) estimation for the PECM using a small dataset with large sensor error, a hybrid approach combined machine learning method with physical method is proposed in this article. First, the Gaussian process regression (GPR) is employed to train an open-loop SOC estimation model with a simple moving window method. Second, a framework of hybrid SOC estimation is proposed based on an improved version of the Kalman filter to improve the robustness of the GPR model with large sensor error. Finally, a series of experiments are carried out to evaluate the proposed approach considering temperature variation, measurement drift noise, and users' habits. It is worth noting that the sampling frequency of training data is only 0.1 Hz. The proposed approach is compared with the most widely used machine learning methods, which verifies the high accuracy and strong robustness of the proposed approach.
MoreTranslated text
Key words
charge estimation,pure electric construction machinery,large sensor error,dataset-based,closed-loop
AI Read Science
Must-Reading Tree
Example
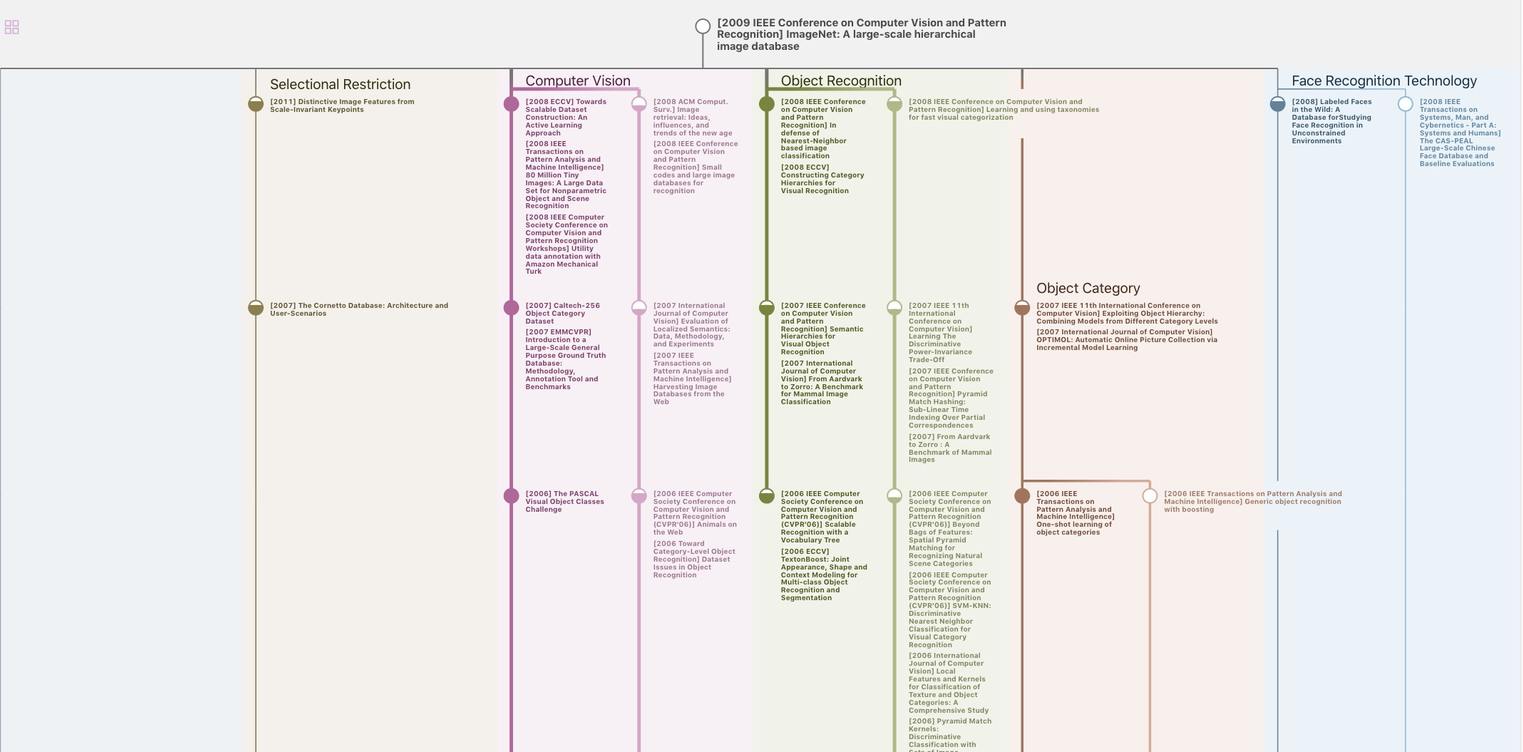
Generate MRT to find the research sequence of this paper
Chat Paper
Summary is being generated by the instructions you defined