Progressive multitask learning for high-resolution prediction of reservoir elastic parameters
GEOPHYSICS(2023)
摘要
Reservoir elastic parameters play an important role in resource exploration; however, the band-limited characteristics of seismic data and the ill-posed nature of seismic inversion significantly affect inversion accuracy. To alleviate this problem, a high-resolution prediction method for reservoir elastic parameters based on the progressive multitask learning network (PMLN) is proposed. Our network consists of three parts: network 1 for low-frequency extension (LFE), network 2 for reservoir parameter inversion, and network 3 for image superresolution (SR). Taking the seismic frequency band as the link, network 1 is first used to predict the low-frequency information of seismic data. Then, the nonlinear mapping relationship between the high-pass-filtered seismic data (and its envelope) and full-frequency seismic data is established. Second, network 2 directly predicts the reservoir elastic parameters using the seismic data after LFE. Finally, the SR of the inversion results is achieved from the image perspective based on network 3. The three networks have a progressive relationship and can share network features, which is beneficial for improving computing efficiency. As the features extracted by the network represent different contributions to the prediction target, a channel attention mechanism is introduced. Furthermore, the loss function of network 2 is improved using dip constraints to obtain high-precision reservoir parameters. Synthetic and field data analyses find that all three networks are competent for their respective tasks, and the PMLN can obtain high-resolution prediction results of reservoir elastic parameters. Compared with traditional full-waveform inversion, the PMLN effectively improves prediction accuracy. Therefore, the PMLN is expected to become a powerful tool for predicting the elastic parameters of reservoirs.
更多查看译文
关键词
reservoir elastic parameters,progressive multitask,prediction,learning,high-resolution
AI 理解论文
溯源树
样例
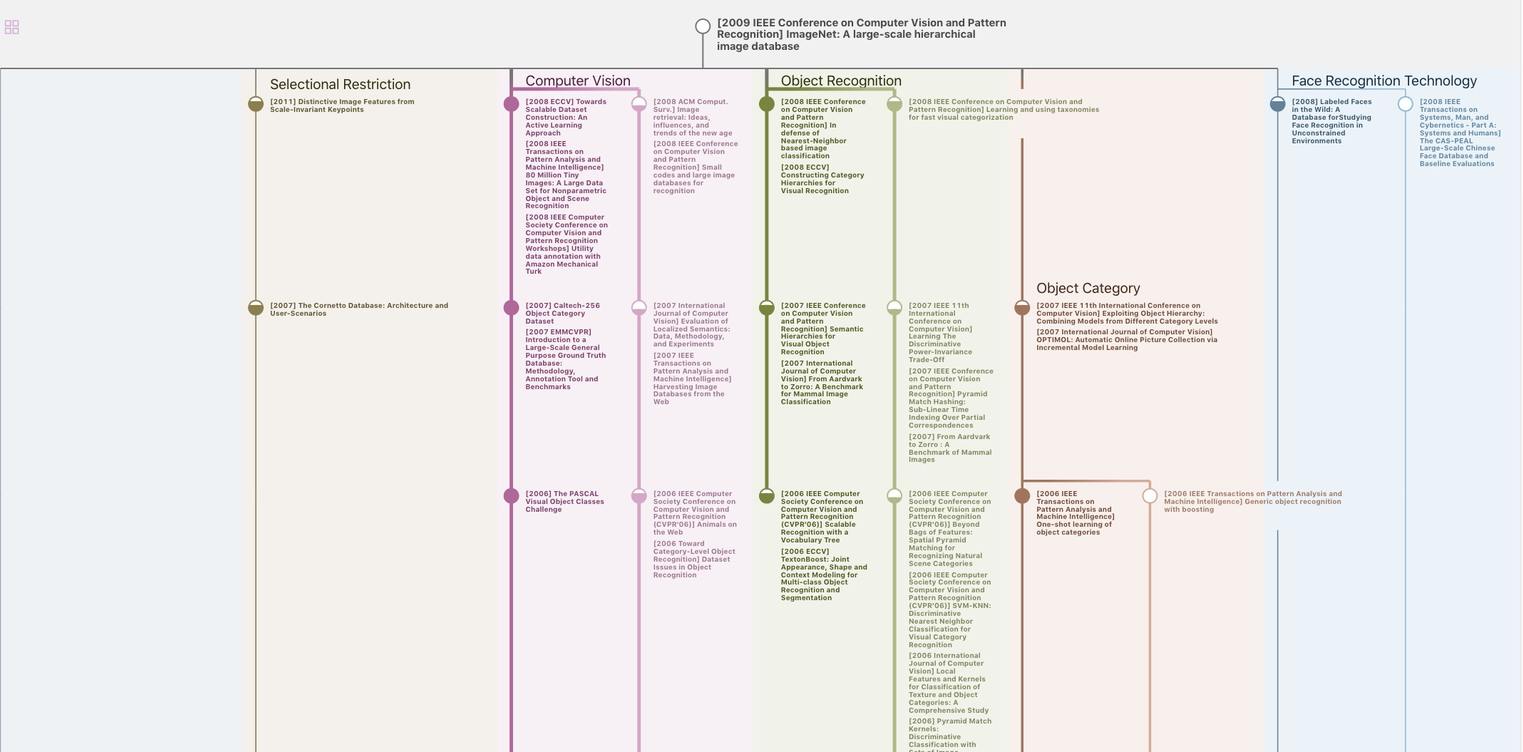
生成溯源树,研究论文发展脉络
Chat Paper
正在生成论文摘要