Online Dominant Generalized Eigenvectors Extraction via a Randomized Algorithm
IEEE Transactions on Vehicular Technology(2023)
摘要
This paper is concerned with online algorithms for the generalized Hermitian eigenvalue problem (GHEP). We first present an algorithm based on randomization, termed alternate-projections randomized eigenvalue decomposition (APR-EVD), to solve the standard eigenvalue problem. The APR-EVD algorithm is computationally efficient and can be computed by making only one pass through the input matrix. We then develop two online algorithms based on APR-EVD for the dominant generalized eigenvectors extraction. Our proposed algorithms use the fact that GHEP is transformed into a standard eigenvalue problem, however to avert computations of a matrix inverse and inverse of the square root of a matrix, which are prohibitive, they exploit the rank-1 strategy for the transformation. Our algorithms are devised for extracting generalized eigenvectors for scenarios in which observed stochastic signals have unknown covariance matrices. The effectiveness and practical applicability of our proposed algorithms are validated through numerical experiments with synthetic and real-world data.
更多查看译文
关键词
Dominant generalized eigenvector extraction,fast subspace estimation and tracking,matrix decomposition,online algorithms,Randomized algorithms,real-time hyperspectral image denoising
AI 理解论文
溯源树
样例
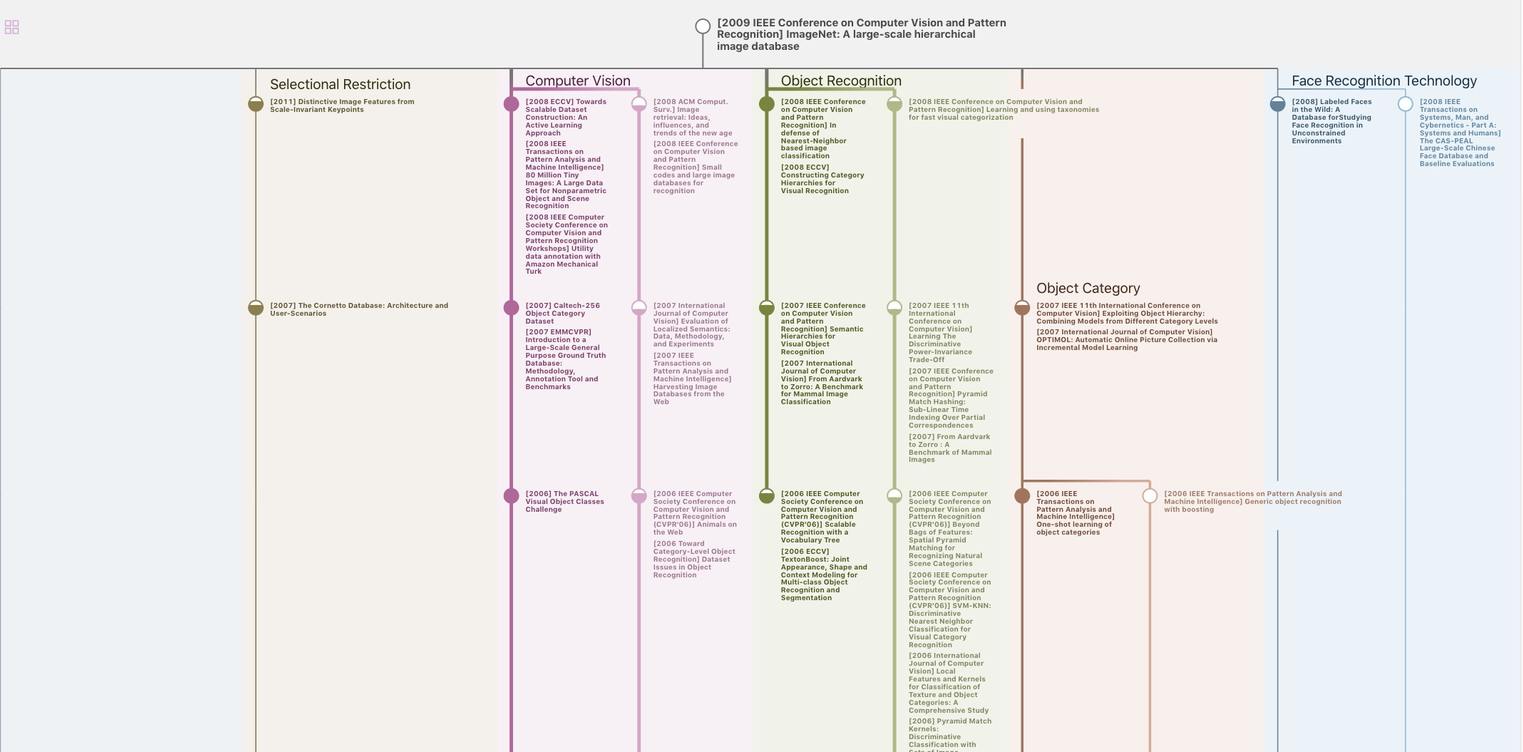
生成溯源树,研究论文发展脉络
Chat Paper
正在生成论文摘要