Worker-Churn-Based Task Assignment With Context-LSTM in Spatial Crowdsourcing
IEEE Transactions on Knowledge and Data Engineering(2023)
摘要
The pervasiveness of GPS-enabled devices and wireless communication technologies flourish the market of Spatial Crowdsourcing (SC), which consists of location-based tasks and requires workers to be at specific locations physically to complete them. In this work, we study the problem of worker-churn-based task assignment in SC, where tasks are assigned by considering workers’ churn. In particular, we aim to maximize the total rewards of task assignments based on the worker churn prediction. To solve the problem, we propose a two-phase framework, which consists of a worker churn prediction and a task assignment phase. In the first phase, we use an LSTM-based model to extract latent feelings of workers based on historical data and then estimate idle time intervals of workers. In the assignment phase, we design an efficient greedy algorithm and a Kuhn-Munkras-based algorithm that can achieve the optimal task assignment. To improve the accuracy of the idle time interval estimation for workers, we adopt a context-dependent LSTM model, which involves interactions between inputs and their context. We further optimize the original task assignment framework by proposing a travel distance optimization strategy to reduce the overall travel distance. Extensive experiments offer insight into the effectiveness and efficiency of the proposed solutions.
更多查看译文
关键词
task assignment,spatial,worker-churn-based,context-lstm
AI 理解论文
溯源树
样例
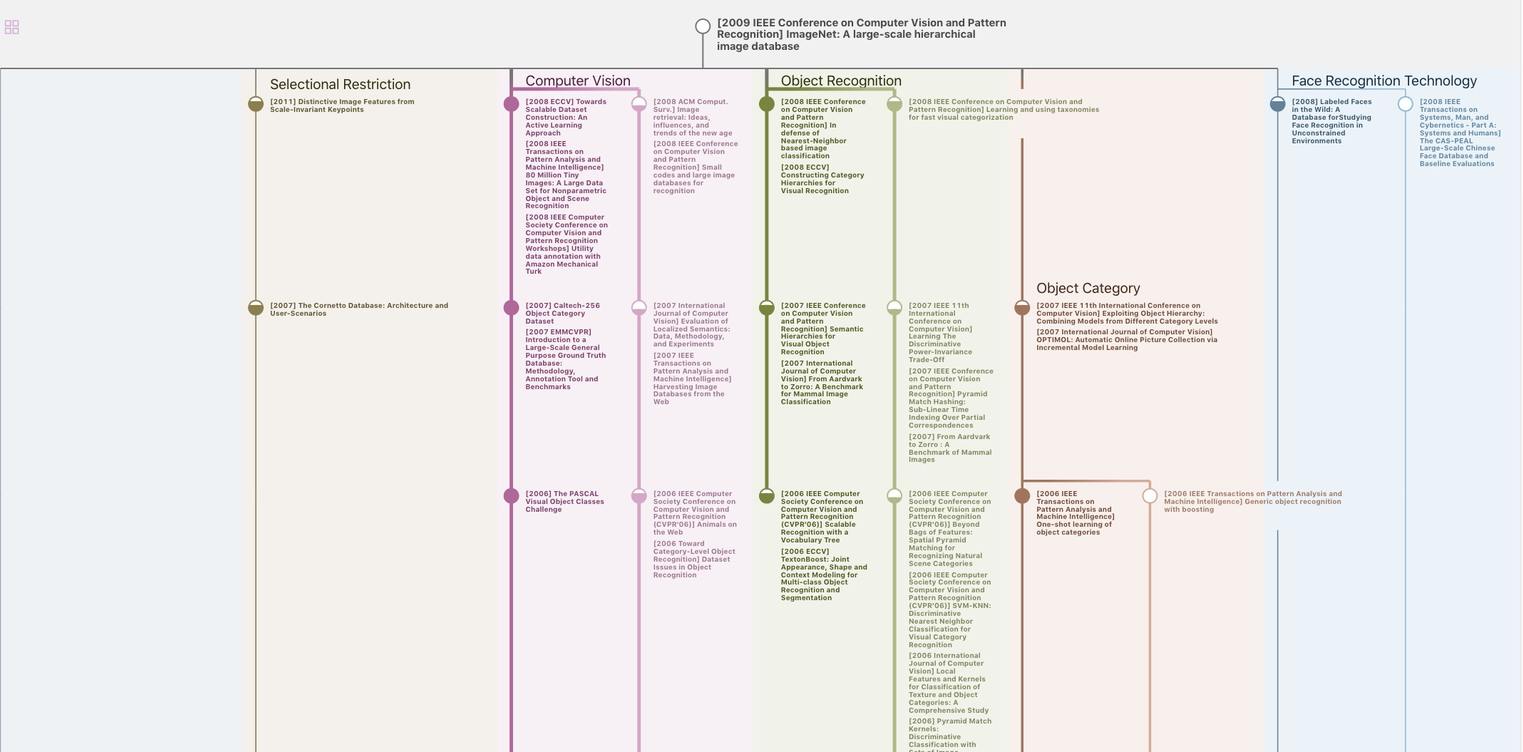
生成溯源树,研究论文发展脉络
Chat Paper
正在生成论文摘要