Deep Tabular Data Modeling With Dual-Route Structure-Adaptive Graph Networks
IEEE Transactions on Knowledge and Data Engineering(2023)
摘要
Thanks to the inherent spatial or sequential structures underlying the data like images and texts, deep architectures such as convolutional neural networks (CNNs) and the Transformer have been recognized as the preeminent approaches in image processing and language modeling. In the real world, there are a large number of tabular data without any explicit structures, which breaks the inductive bias of most neural networks like CNNs. Although multi-layer perceptrons (MLPs) obtain empirical success on tabular data, they cannot well explain the underlying relationship between multiple variables. Compared with other fields, research on deep models toward tabular data has received relatively less scrutiny. To bridge this gap, we propose Dual-Route Structure-Adaptive Graph Networks (DRSA-Net) to model the nonlinearity in tabular feature vectors without any prior. DRSA-Net adaptively learns a sparse graph structure between variables and then characterizes interactions between them from the view of dual-route message passing. We demonstrate that DRSA-Net could easily degenerate into the typical MLPs and factorization machines (FMs). Extensive experiments on recommendations, images (no spatial information after preprocessing), and some benchmark machine learning datasets show that DRSA-Net achieves comparable or superior performance with many classic algorithms and recently proposed deep models.
更多查看译文
关键词
Feature interactions,graph neural networks,graph structure learning,message passing,tabular data
AI 理解论文
溯源树
样例
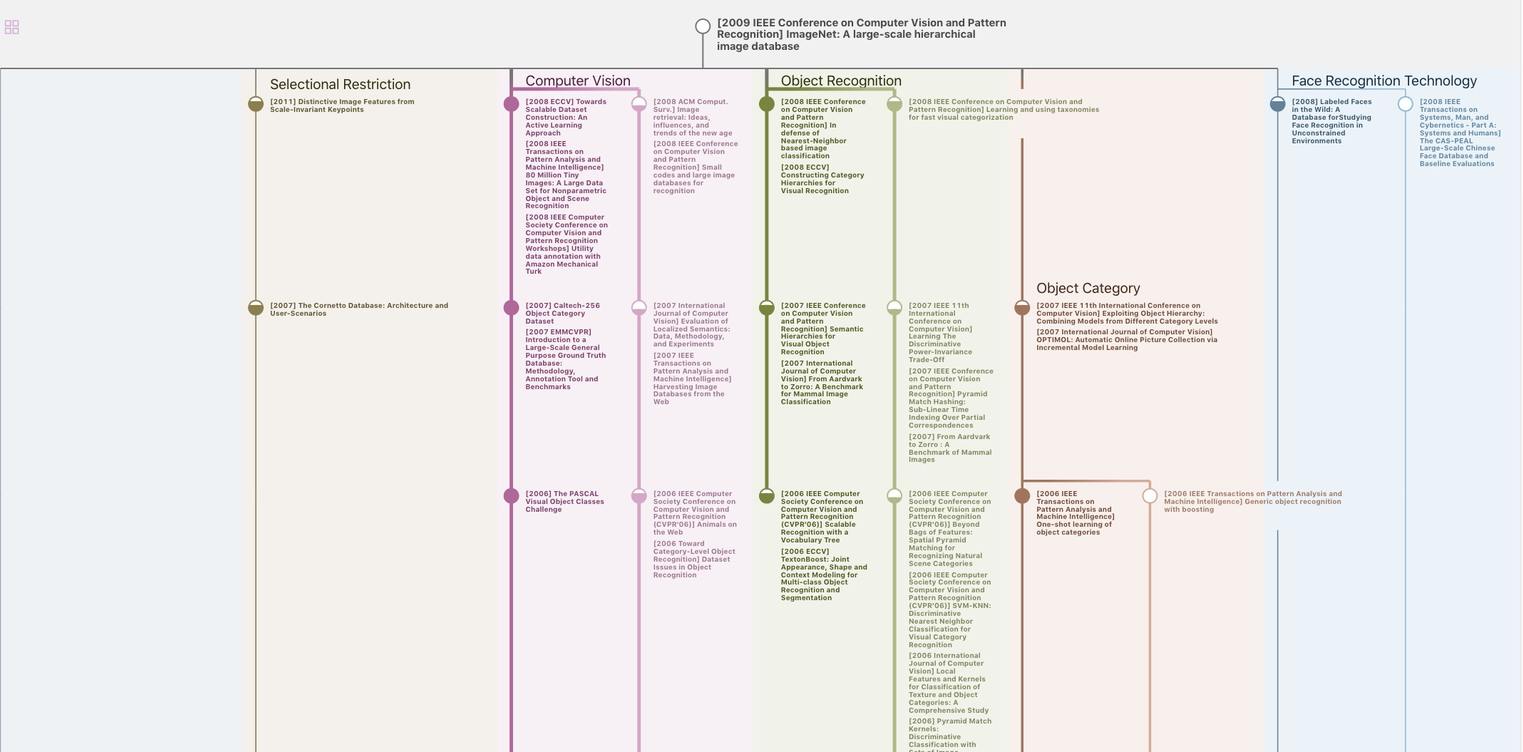
生成溯源树,研究论文发展脉络
Chat Paper
正在生成论文摘要