Full-Scene Defocus Blur Detection With DeFBD+ via Multi-Level Distillation Learning.
IEEE Transactions on Multimedia(2023)
摘要
Existing defocus blur detection (DBD) methods generally perform well on a single type of unfocused blur scene (e.g., foreground focus), thereby suffering from the performance degradation for the other types of unfocused blur scenes. In this paper, we present the first exploration on full-scene DBD, and propose a separate-and-combine framework to achieve excellent performance for diverse defocus blur scenes. We firstly structure full-scene DBD dataset (named as DeFBD+) through collecting more types of unfocused blur scenes (e.g., background focus, full focus and full out of focus) with pixel-level annotations. Then, to avoid performance degradation caused by mutual interference from local feature representation and global content perception, we implement a pixel-level DBD network and an image-level DBD classification network to learn these two abilities separately. After that, we propose an isomeric distillation mechanism to combine these two abilities. Extensive experiments show that the proposed approach achieves superior performance compared with state-of-the-art methods.
更多查看译文
关键词
Full-scene defocus blur detection,local feature representation,global content perception,isomeric distillation mechanism
AI 理解论文
溯源树
样例
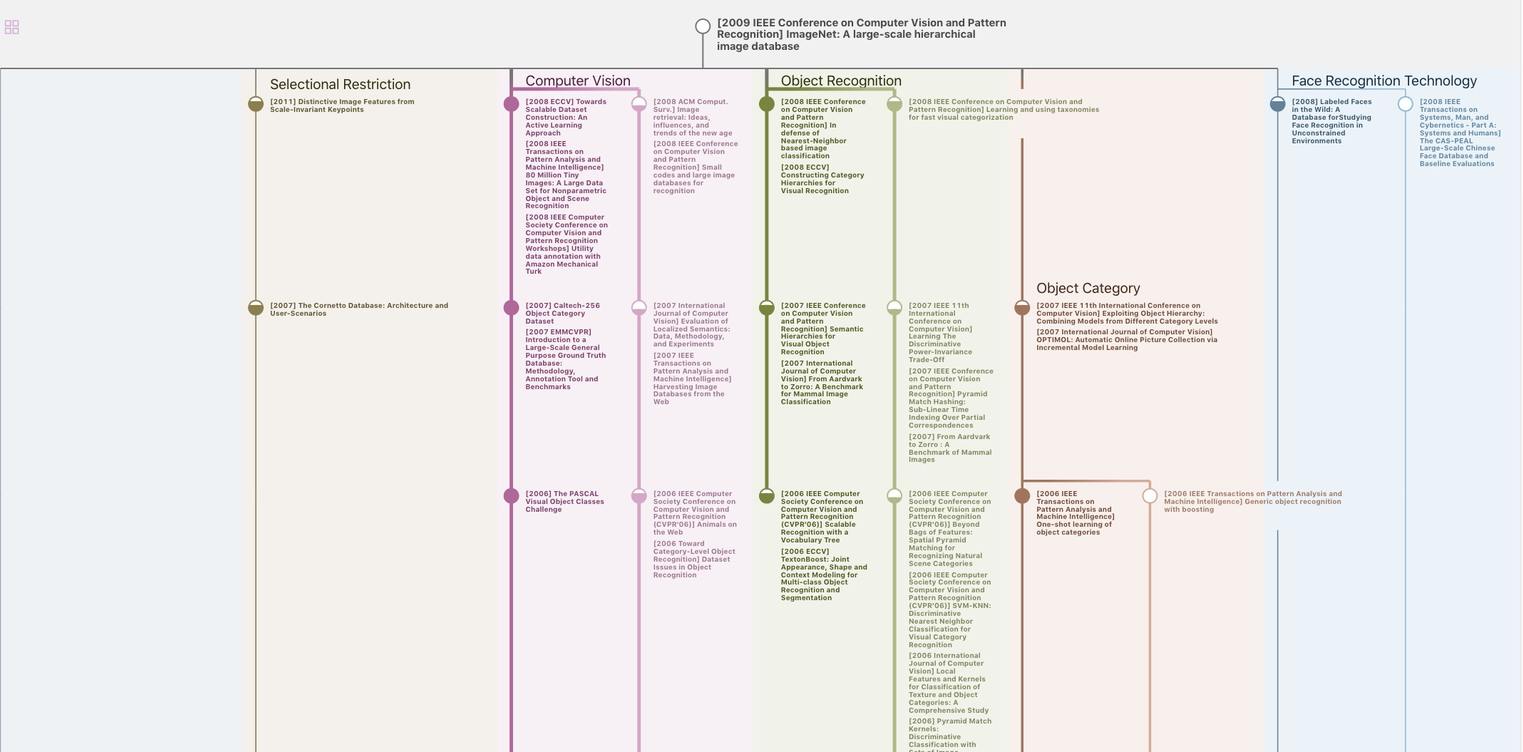
生成溯源树,研究论文发展脉络
Chat Paper
正在生成论文摘要