Regional Detection of Landslide and Flash Flood Events in the East African Rift
crossref(2023)
AI Read Science
Must-Reading Tree
Example
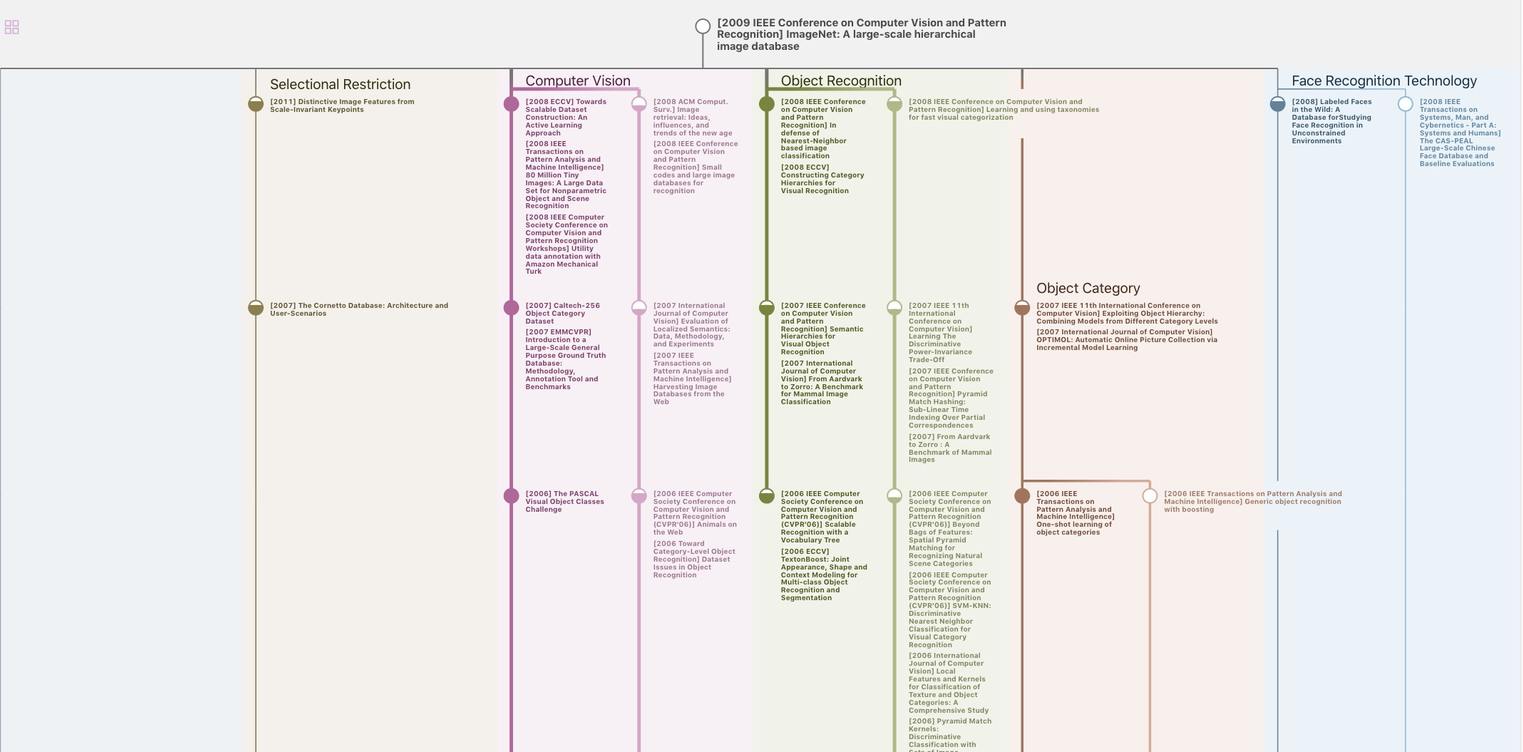
Generate MRT to find the research sequence of this paper
Chat Paper
Summary is being generated by the instructions you defined