Learning font-style space using style-guided discriminator for few-shot font generation
EXPERT SYSTEMS WITH APPLICATIONS(2024)
摘要
Font synthesis has become a quite active field of research since manual font design requires extensive domain tradecraft expertise that is labor intensive and time consuming, especially for languages with large number of characters such as Chinese and Korean. While remarkably successful, existing methods for font synthesis have major shortcomings in their requirement to fine-tune the network for unobserved font styles, which requires large reference images. Additionally, the recent few-shot font synthesis methods are either designed for specific language systems, or they operate on low-resolution images, which limits their usage. For learning distinct font styles, most methods rely on multitask discriminators that use style labels. Such dependency on style labels ignores the semantic distances between different font styles. In this paper, we tackle this font synthesis problem by learning the font style in the embedding space. To this end, we propose a font style-guided discriminator that simultaneously acts as a style encoder and adversarial network as it learns to separate font styles in the embedding space, in which the semantic distances directly correspond to the measure of font style similarity while simultaneously guiding the generator. We also design the network architecture and training procedure that mitigates the problems discussed above. Thanks to this approach, the proposed method outperforms existing state-of-the-art few-shot font generation methods both qualitatively and quantitatively.
更多查看译文
关键词
Font generation,Generative adversarial networks,Style transfer,Similarity learning,Metric learning
AI 理解论文
溯源树
样例
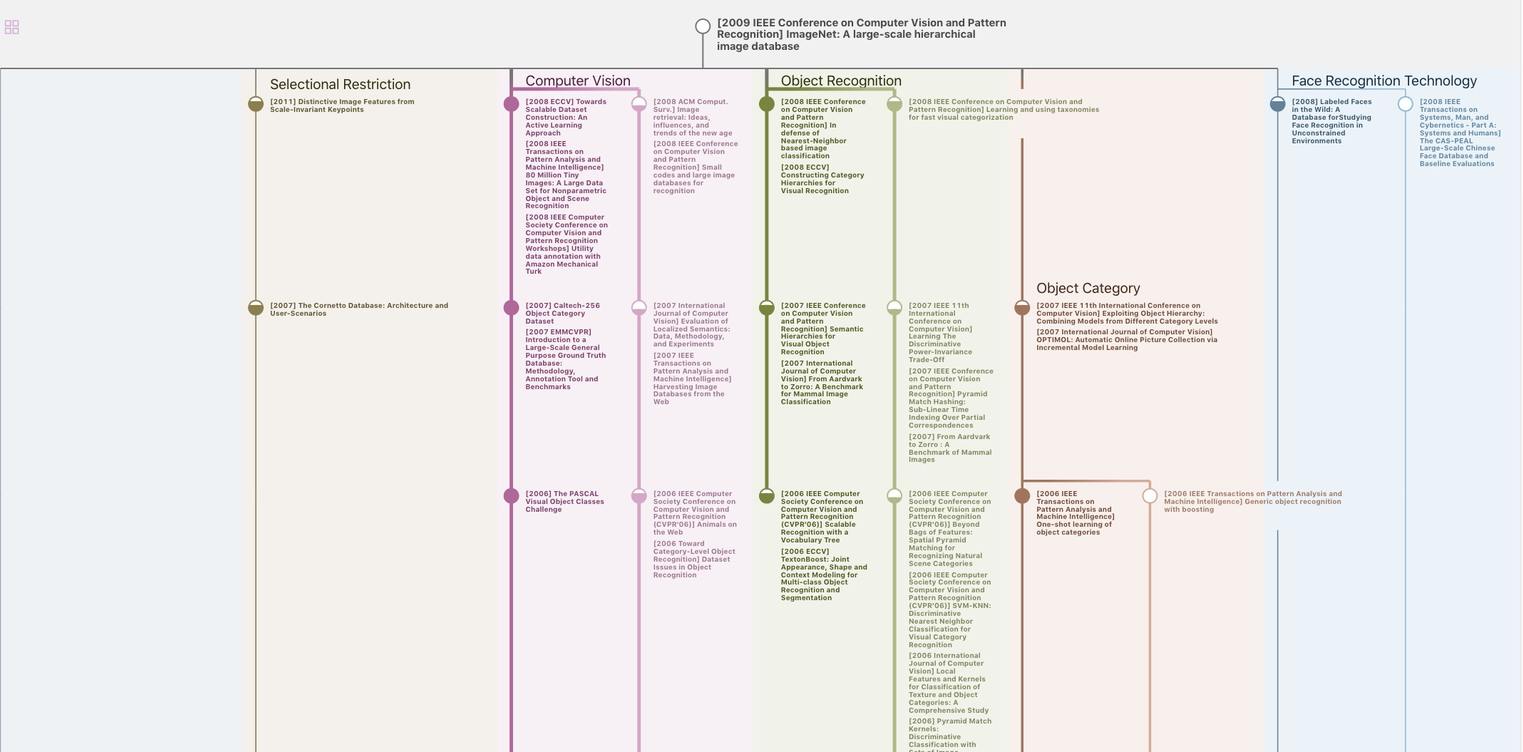
生成溯源树,研究论文发展脉络
Chat Paper
正在生成论文摘要