Image-based velocity estimations under different seeded and unseeded river flows
crossref(2023)
摘要
<p>The use of image velocimetry techniques for river monitoring has been increasing in the last few years, but there are some limitations to be solved related mainly to natural environmental conditions and operative framework (Dal Sasso et al., 2021). Along with these issues, the need for surface tracking features or homogeneously distributed materials across the cross-section represents one of the challenges for outdoor applications. In a natural environment, flows can present low seeding densities or locally distributed tracer clusters. These conditions can introduce a high variance and underestimate the flow velocity field, especially near the riverbanks.</p> <p>In this work, the Farnebäck dense optical flow method (Farnebäck 2003) implemented in SSIMS-Flow software (Ljubicic, 2022) was tested and compared with LSPIV technique (Thielicke et al., 2021) to estimate surface flow velocities under different seeding conditions. The application was carried out on the Arrow River (UK) along two meandering river reaches during low-flow conditions. Four different seeding conditions were experimented from low (natural) to high (artificial) seeding density of tracers . Tracers were manually distributed onto the water surface and videos were acquired from DJI Phantom 4 Pro. Seeding metrics were used to estimate seeding conditions including: mean tracer area, seeding density, spatial tracer distribution, and the SDI index (Pizarro et al., 2020). Conventional velocity measurements were used as benchmark purposes along various transects.</p> <p>This study highlighted the good performances of the two tested image velocimetry methods, with results comparable to traditional techniques. On the one hand, the Farnebäck optical flow method proved to be more sensitive to changing setting parameters (e.g., feature extraction rate) with respect to LSPIV. On the other hand, optical flow showed low sensitivity to seeding density (error reduction 30-40%). This is due to the capacity of the Farnebäck method integrated with an ad-hoc pooling technique for spatial velocity averaging to represent surface velocity under sporadic and uneven seeding (e.g., near the convex bank).</p> <p><strong>References </strong></p> <p>Dal Sasso, S. F., Pizarro, A., Manfreda S. (2021). Recent Advancements and Perspectives in UAS-Based Image Velocimetry. Drones 5, 3: 81.</p> <p>Farnebäck, G. (2003). Two-frame motion estimation based on polynomial expansion, Scandinavian conference on Image analysis. Springer, Berlin, Heidelberg.</p> <p>Ljubicic, R. (2022). SSIMS-Flow: UAV image velocimetry workbench, https://github.com/ljubicicrobert/SSIMS-Flow</p> <p>Pizarro, A., Dal Sasso, S.F., Manfreda, S. (2020). Refining image-velocimetry performances for streamflow monitoring: Seeding metrics to errors minimization. Hydrol. Process. 2020, 34, 5167–5175.</p> <p>Thielicke, W., Sonntag, R. (2021). Particle Image Velocimetry for MATLAB: Accuracy and Enhanced Algorithms in PIVlab. Journal of Open Research Software, 9, Ubiquity Press, 2021.</p>
更多查看译文
AI 理解论文
溯源树
样例
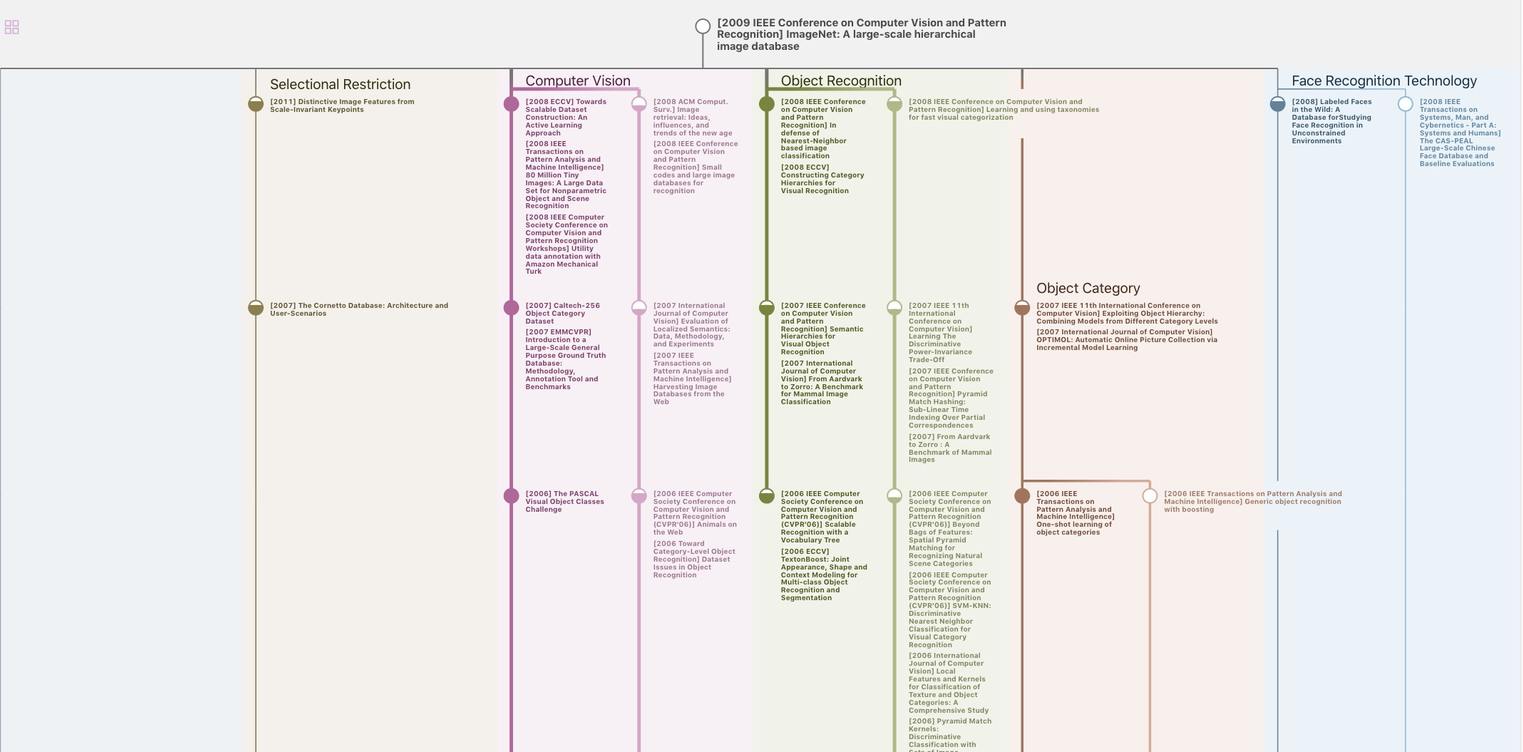
生成溯源树,研究论文发展脉络
Chat Paper
正在生成论文摘要