Usage of 3D U-net Convolutional Neural Network for the inspection of aerospace components
e-Journal of Nondestructive Testing(2023)
摘要
This work illustrates the use of deep learning methods applied on X-ray computed tomography (XCT) datasets to segment pores and fibres in reinforced composite components from the aeronautic industry by binary semantic segmentation. We first apply data pre-processing, and then employ a modified 3D U-Net, representing a convolutional neural network. Tweaking hyper-parameters, we have reached an optimal model for our datasets. One of the models has reached 99% segmentation accuracy when testing using a Dice function. In our experiments, pores and fibres in XCT datasets of aerospace components, more specifically of glass and carbon fibre reinforced composites, were segmented and analysed. In order to compare this modified 3D U-Net architecture with segmentation methods currently used in the industry, the datasets were also input to conventional Otsu thresholding. Our results shows that modified 3D U-Net performs better than Otsu thresholding, especially on the segmentation of small pores. Modified 3D U-Net also showed reasonable prediction accuracy when testing with an optimised model which was trained with a low number of dataset both for fibre and pore segmentation.
更多查看译文
关键词
inspection,neural network,3d,components,u-net
AI 理解论文
溯源树
样例
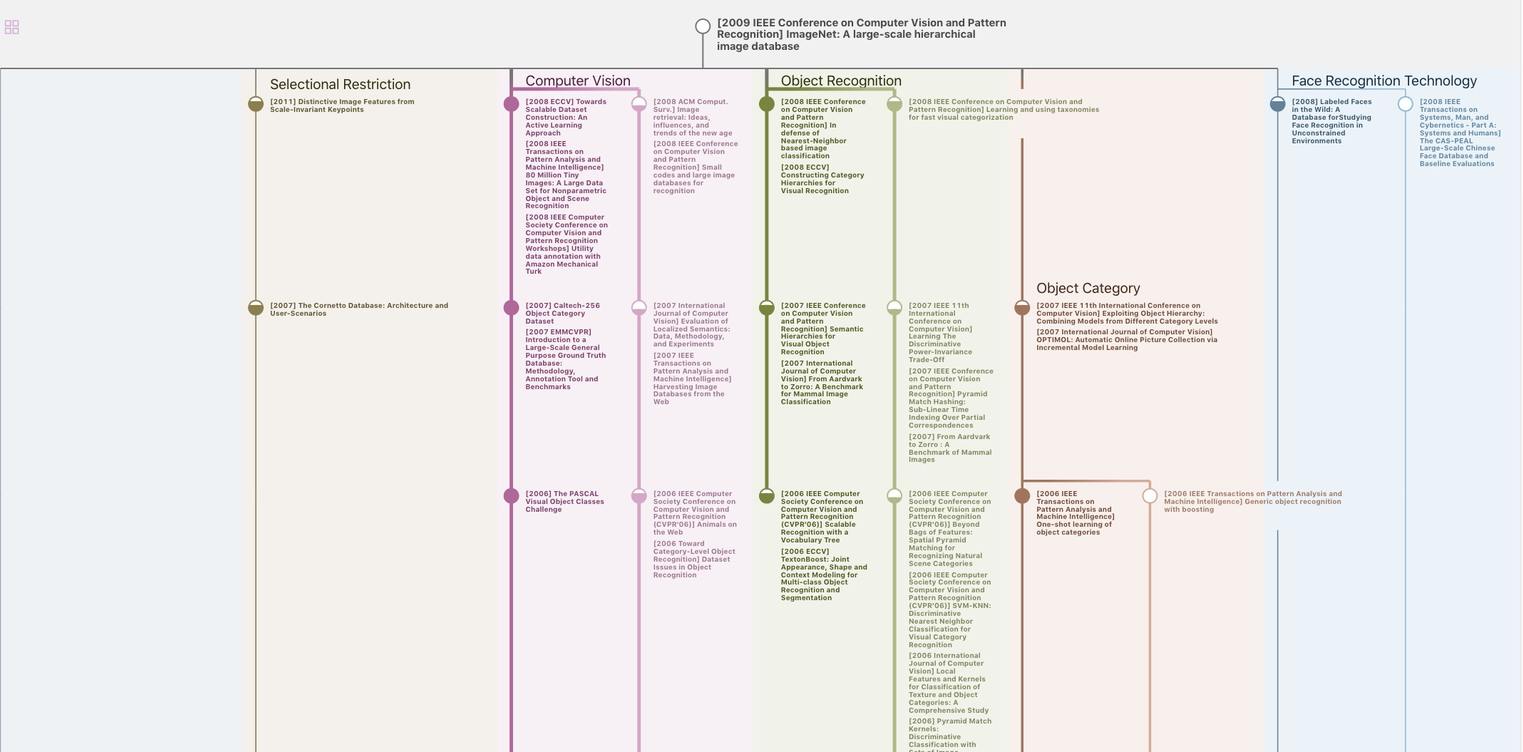
生成溯源树,研究论文发展脉络
Chat Paper
正在生成论文摘要