Identifying burnt areas and flood monitoring based on multi-spectral passive and microwave active remote sensing in tropical peatlands.
crossref(2023)
Abstract
<p>Destabilisation of hydrological conditions and associated fire occurrence are the most significant barriers hindering degraded tropical peatland revegetation. For this reason, the monitoring of fires and hydrological conditions is crucial for guiding drained tropical peatland restoration. One of the best tools for large-scale monitoring of the natural environment, especially when access and <em>in situ</em> information are limited, is satellite remote sensing, and fusion of active and passive remote sensing data can provide new insights into dynamic systems such as peatlands. There is usually a relationship between automation, complexity and processing time leading to variations in the method's effectiveness, including reliability and accuracy. The main goal of this work was to develop a rapid method for ease of use by non-specialist users, which has capability to deliver reliable results describing the mapping of the burnt and flooded areas. In this case, two types of data, from multi-spectral passive and microwave active remote sensing sensors, were combined to monitor fires and floods in a 5,000 km² area of tropical peatland of varying land use and level of degradation in Central Kalimantan. Both imaging techniques provide different information. The vegetation index of the differenced Normalised Burn Ratio (dNBR), calculated based on Sentinel-2 and Landsat-8 data, delivers information for mapping burned areas. The backscattering coefficient from Sentinel-1 data can identify permanent and ephemeral water bodies. These methods were effective for detection of burnt areas and water bodies, but there were limitations of the passive sensors' image availability due to cloud cover. In addition, using dNBR and backscattering coefficient separately in some cases caused false positive results (e.g. burnt areas classified as water bodies, or burnt areas detected in the main river bed). The fusion of two data sources increased fire and flood mapping accuracy by eliminating misclassification errors, compared to using them separately, thus indicating their strong complementarity. This combined method allowed analysis of the history of fires and flooding in 2015-2022, and the relationship between these; preliminary results to be presented.</p>
MoreTranslated text
AI Read Science
Must-Reading Tree
Example
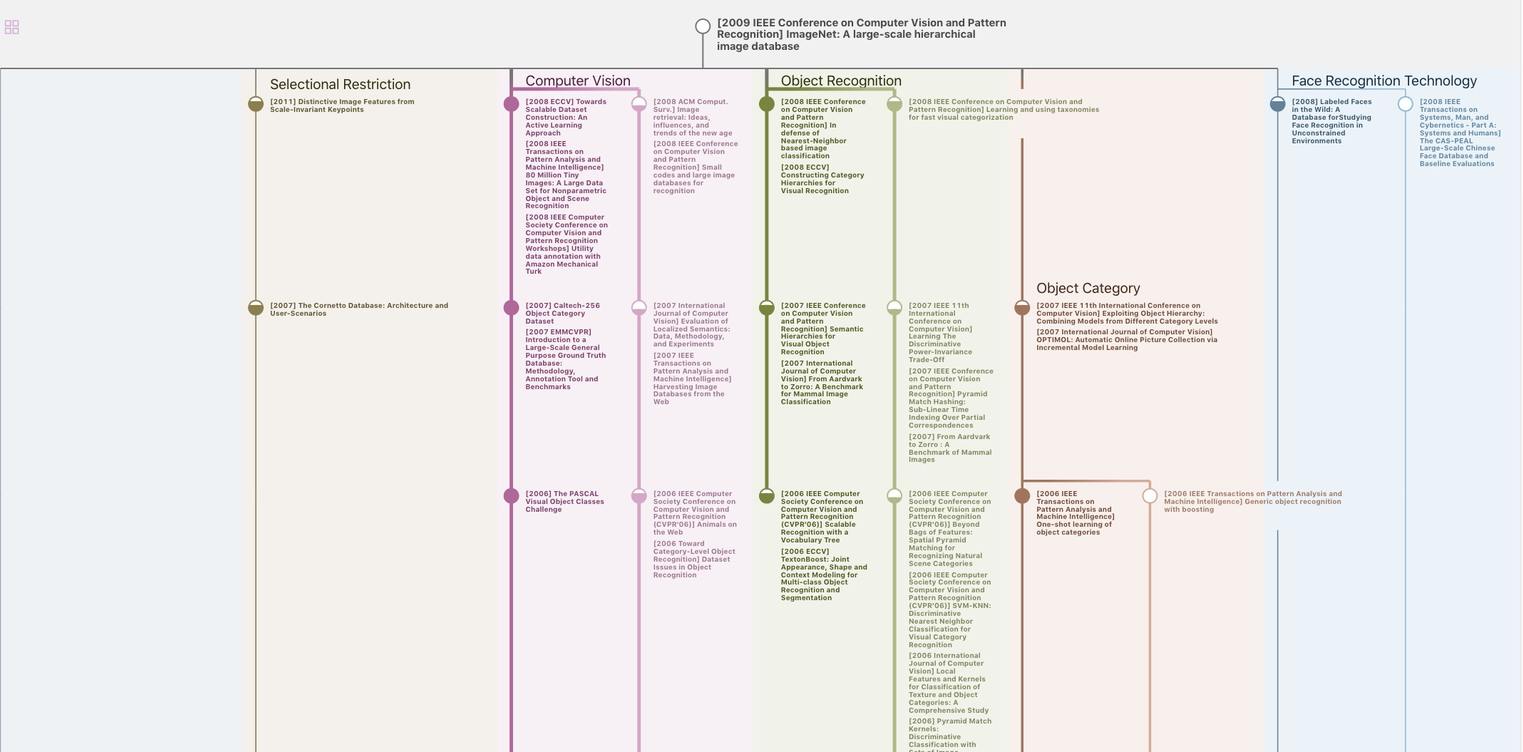
Generate MRT to find the research sequence of this paper
Chat Paper
Summary is being generated by the instructions you defined