Global runoff partitioning based on Budyko and machine learning
crossref(2023)
摘要
<p>Partitioning runoff accurately into baseflow and quickflow is crucial for our understanding of the water cycle and for the management of droughts and floods. However, global datasets of long-term mean runoff partitioning are rare, even more so datasets relying on physically-based methods. Here, we present a new global 0.25° dataset of runoff, baseflow and quickflow using a hybrid approach of Budyko-based methods and machine learning (ML). The parameters in the Budyko curve and Budyko-based baseflow curve (BFC curve) are estimated with ML (boosted regression trees, BRT) as a function of catchment characteristics. The BRT models are trained and tested in 1226 catchments worldwide, and then applied globally at grid scale. The catchment-trained models show good performance during the testing phase with <em>R<sup>2</sup></em> equal to 0.96 and 0.87 for runoff and baseflow, respectively. The dataset developed in this study shows that 30.3±26.5% (mean ± standard deviation) of the precipitation is partitioned into runoff of which 20.6±22.1% is baseflow and 9.7±10.3% is quickflow. The global long-term mean baseflow in this study (151±181 mm yr<sup>–1</sup>) is lower than that from the Global Streamflow Characteristics Dataset (GSCD, 241±321 mm yr<sup>–1</sup>) and higher than that from ERA5-Land (79±145 mm yr<sup>–1</sup>). This study provides a unique, physically and observationally constrained global dataset of the long-term runoff partitioning. The large differences among different datasets suggest that global runoff partitioning is highly uncertain and requires further investigation.</p>
更多查看译文
AI 理解论文
溯源树
样例
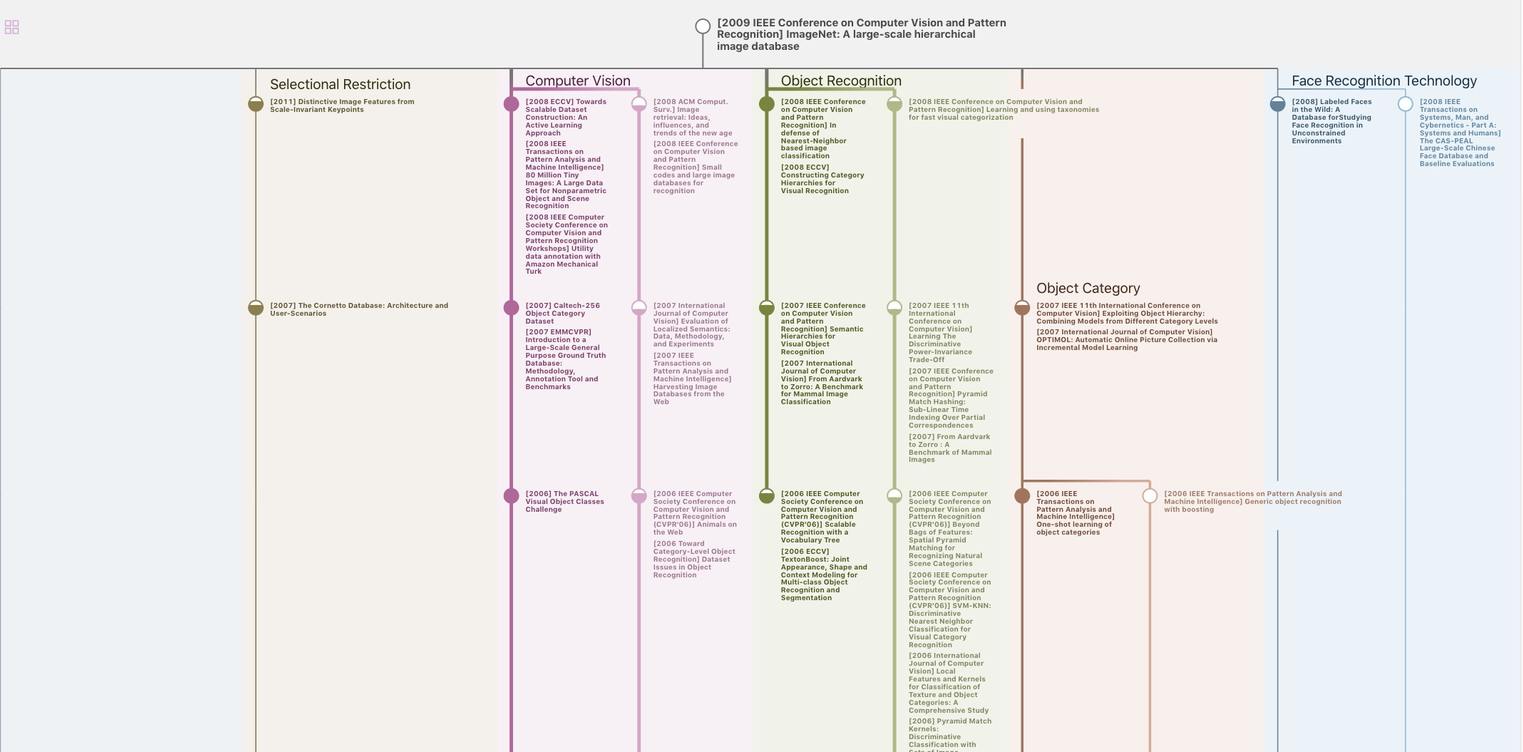
生成溯源树,研究论文发展脉络
Chat Paper
正在生成论文摘要