Learning-based Trajectory Optimization for a Twin Robotic CT System
e-Journal of Nondestructive Testing(2023)
摘要
Non-circular twin robot computed tomography (CT) systems provide a high degree of flexibility in positioning the X-ray source and detector. This makes the design of optimal CT trajectories particularly important, as it can potentially allow for a reduction in the number of projections required for image acquisition. Therefore, the optimization of CT trajectories is a key area of research in this field. This paper presents a method for optimising the trajectory of a non-circular twin robot computed tomography system, using a so-called detectability index to guide the optimization process. The proposed method involves simulating possible X-ray projections that are reachable by the robotic CT system and using a regressive ResNet-34 to predict the detectability index of each projection. An integer optimization problem is then used to generate an optimised CT trajectory based on this prediction, with the haversine distance between projections serving as an additional constraint. The performance of the ResNet-34 is evaluated on unseen projections. It effectively predicted the detectability index for a spherical sampling space, allowing for the optimization of arbitrary CT trajectories. In addition, the method was able to identify valuable projections according to the detectability index, enabling the reconstruction of a test object with only 30 out of 800 of the previously acquired projections without significant loss of information in the reconstruction domain.
更多查看译文
关键词
trajectory optimization,robotic,learning-based
AI 理解论文
溯源树
样例
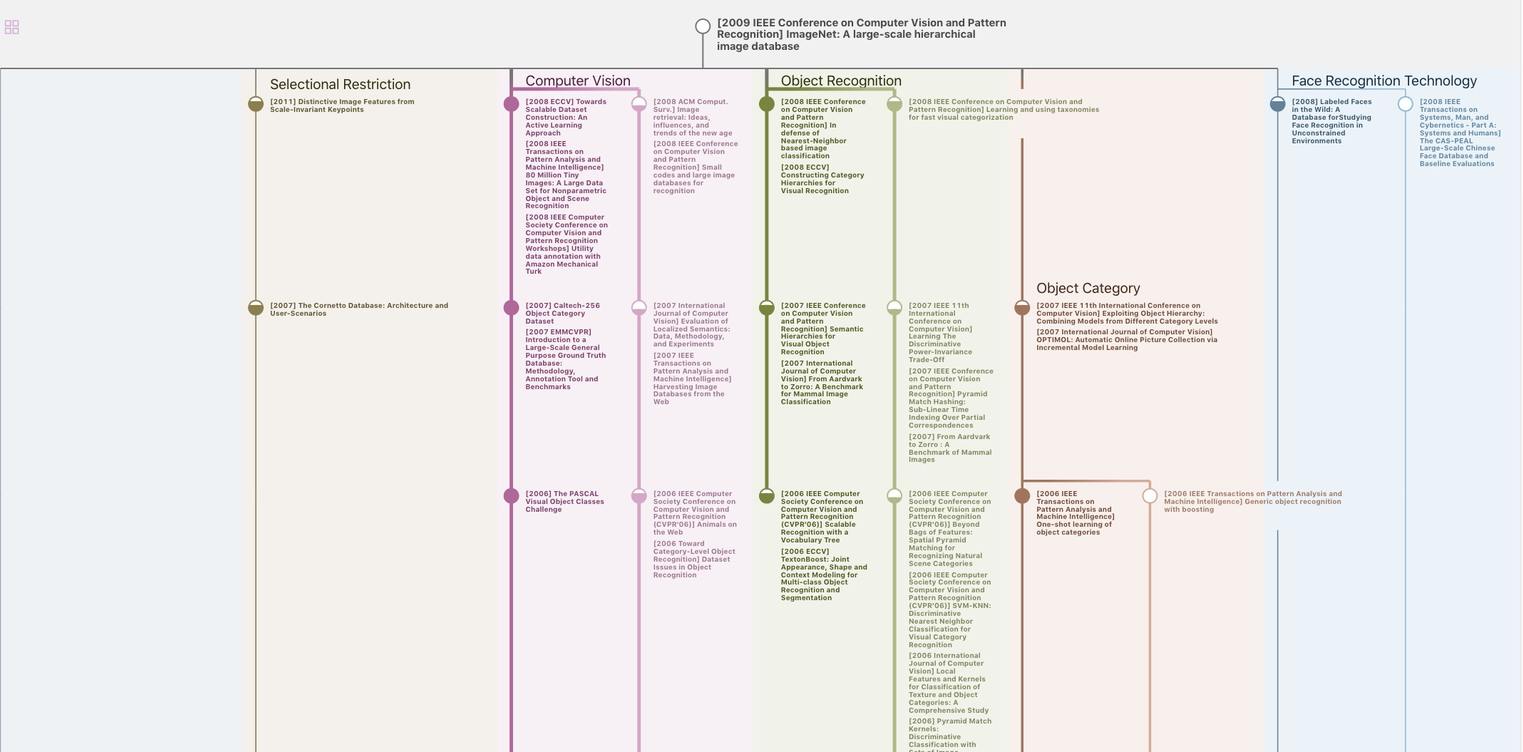
生成溯源树,研究论文发展脉络
Chat Paper
正在生成论文摘要