Upsampling Monte Carlo neutron transport simulation tallies using a convolutional neural network
ENERGY AND AI(2023)
摘要
The physical quantities calculated by nuclear reactor Monte Carlo simulations are typically recorded on a grid of two or three spatial dimensions and one dimension of neutron energy. Because of this, increasing the resolution of the calculated quantities can have a significant impact on the memory and CPU time required to run a simulation. Convolutional neural networks have been shown to accurately upsample coarse-resolution photographic images to resolutions multiple times finer than the originals. Here we show that a convolutional neural network can accurately upsample flux tallies in a Monte Carlo neutron transport simulation by a factor of two along the spatial and energy dimensions. Neutron flux tallies in pressurized water reactor assemblies were calculated using OpenMC at a 64 x 64 pixel spatial resolution and 8 neutron energy groups for input to the neural network. The network upsamples the low-resolution neutron flux to 128 x 128 pixel spatial resolution and 16 neutron energy groups. High-resolution neutron flux tallies and their uncertainties were also calculated with OpenMC and compared with the network's predictions. The upsampled data and the high-resolution tally results agree to within the statistical uncertainty calculated by OpenMC.
更多查看译文
关键词
OpenMC,Convolutional neural network,Residual network,Neutron Monte Carlo
AI 理解论文
溯源树
样例
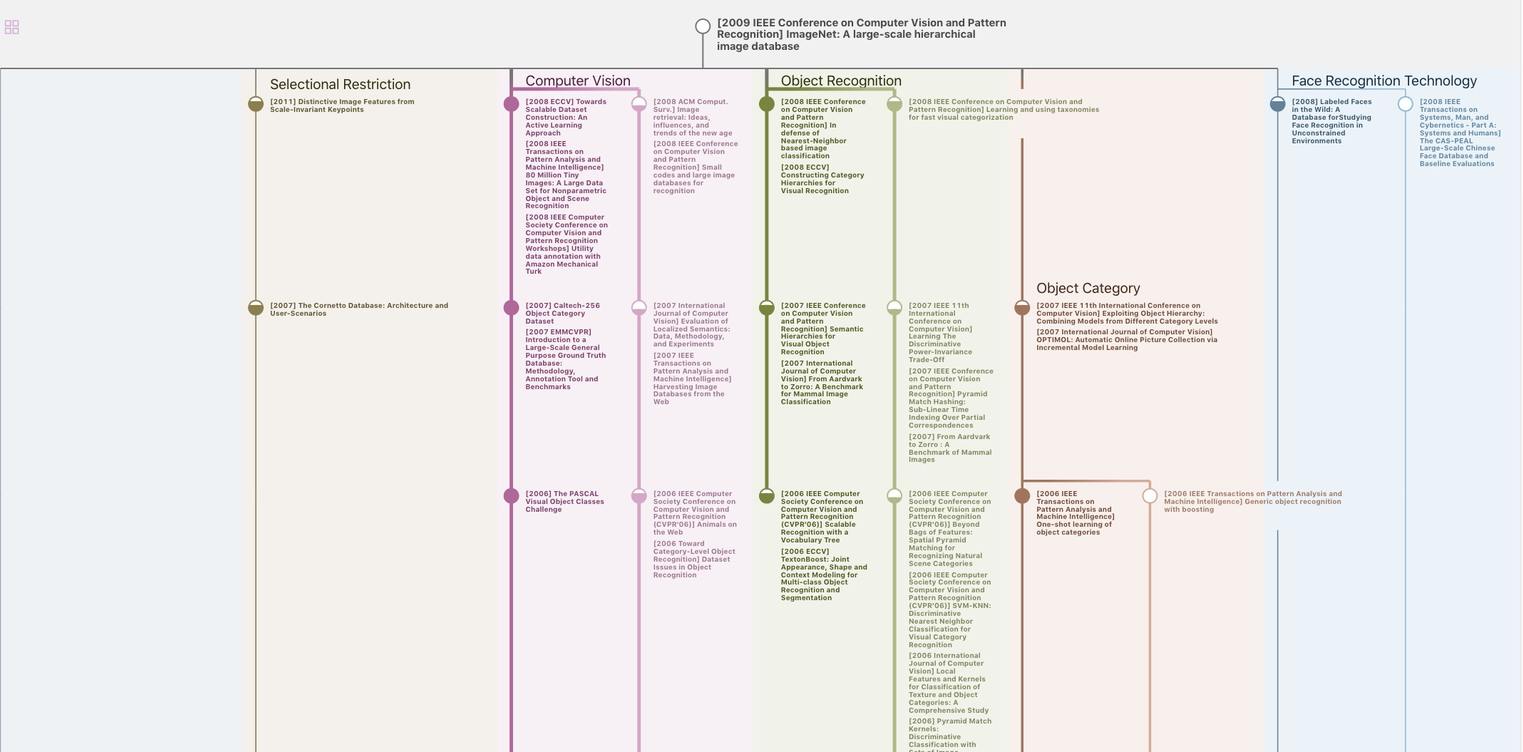
生成溯源树,研究论文发展脉络
Chat Paper
正在生成论文摘要