Forecasting the loss given default of bank loans with a hybrid multilayer LGD model by extending multidimensional signals
Journal of Risk Model Validation(2022)
Abstract
According to the Basel II and Basel III accords, loss given default (LGD) is an important component of credit risk assessment. To improve the accuracy of LGD prediction, this paper uses signaling theory and machine learning methods to study the LGD predictions of commercial banks by considering the venture quality and level of uncertainty. First, from the perspective of signal costs, signaling theory is used to analyze the impacts of venture quality and extended level of uncertainty signals on LGD. Then, a new hybrid multilayer LGD model-based hierarchical framework is proposed for studying the LGD predictions of commercial banks in a highdimensional unbalanced data context. The experimental results demonstrate that the venture quality and level of uncertainty significantly affect LGD. Additionally, the hybrid multilayer LGD model - consisting of logistic classification, random oversampling examples combined with random forest classification and ordinary least squares regression - not only yields high levels of prediction accuracy and interpretability in high-dimensional unbalanced data contexts but is also robust regardless of the selection of the training set and samples used. The results of the study serve as an important reference for decision-making by commercial banks in their assessment of default losses and the risk management of small/startup companies.
MoreTranslated text
Key words
credit risk assessment, loss given default (LGD) prediction, signaling theory, machine learning, interpretability
AI Read Science
Must-Reading Tree
Example
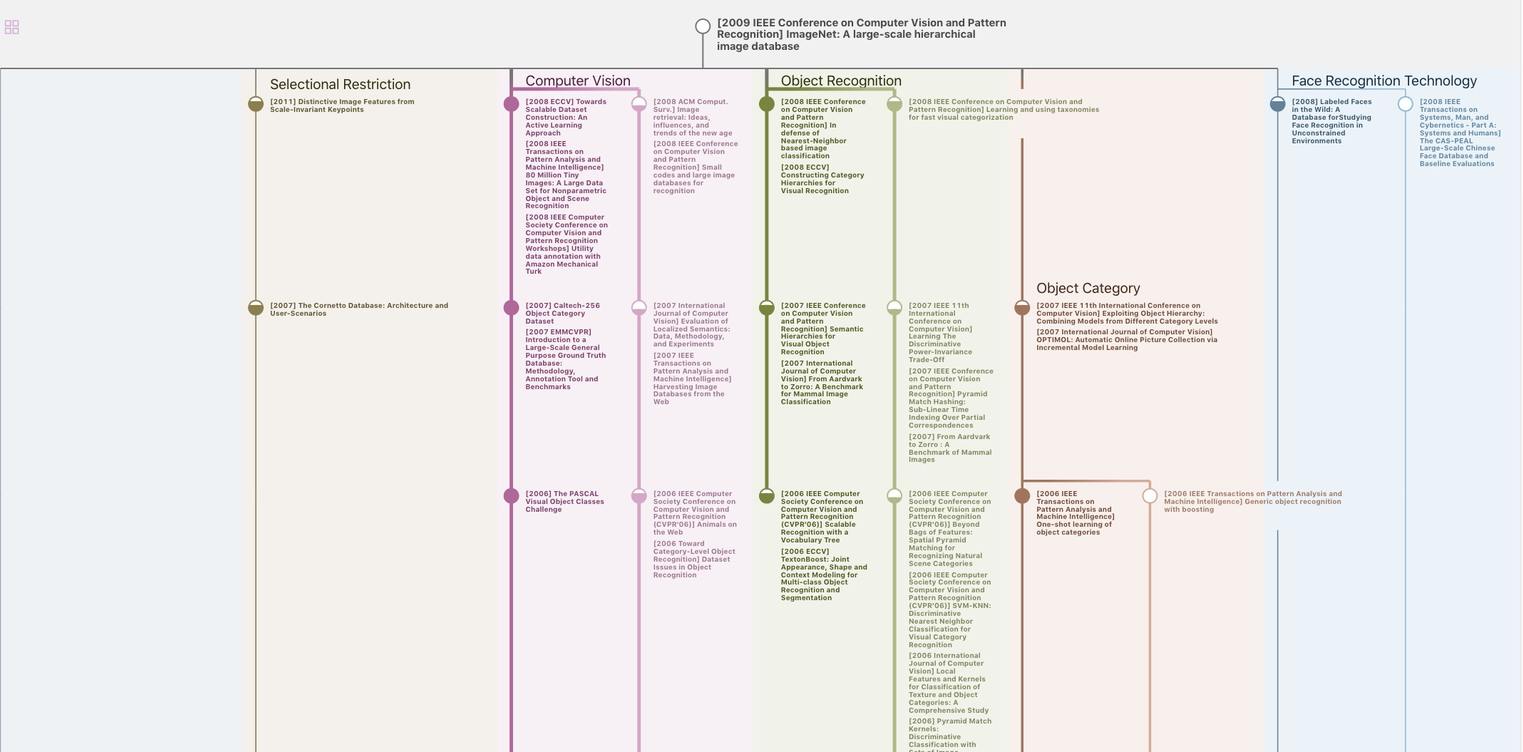
Generate MRT to find the research sequence of this paper
Chat Paper
Summary is being generated by the instructions you defined