Tensor-Based Forward-Backward Algorithms in Physics-Informed Coupled Hidden Markov Model
IEEE Transactions on Artificial Intelligence(2022)
摘要
Imposing data-driven with physical laws for user activity prediction could effectively solve various physical problems such as smart care, surveillance and human-robot. In the growing field of artificial intelligence, the application of activity prediction based on physical Coupled Hidden Markov Model (CHMM) and tensor theory with physical properties has attracted increasing attentions. However, existing CHMMs usually only consider the time-series characteristic of data, while ignoring physical characteristics of user activity such as periodicity, timing and correlation. Moreover, they are all matrix-based models, which could not holistically analyze the dependencies among physical states. The above disadvantages lead to lower prediction accuracy of CHMM. To remove these disadvantages, three physics-informed tensor-based CHMMs are first constructed by incorporating prior physical knowledge. Then the corresponding forward-backward algorithms are designed for resolving the evaluation problem of CHMM. These algorithms could overall model multiple physical features by imposing physics and prior knowledge into CHMM during training to improve the precision of probabilistic computing. The algorithms reduce the dependence of the model on training data by adding physical features. Finally, the comparative experiments show that our algorithms have better performances than existing prediction methods in precision and efficiency. In addition, further selfcomparison experiments verify that our algorithms are effective and practical.
更多查看译文
关键词
Activity Prediction,Coupled Hidden Markov Model (CHMM),Forward-Backward Algorithms,Physics- Informed Machine Learning,Tensor Algebra
AI 理解论文
溯源树
样例
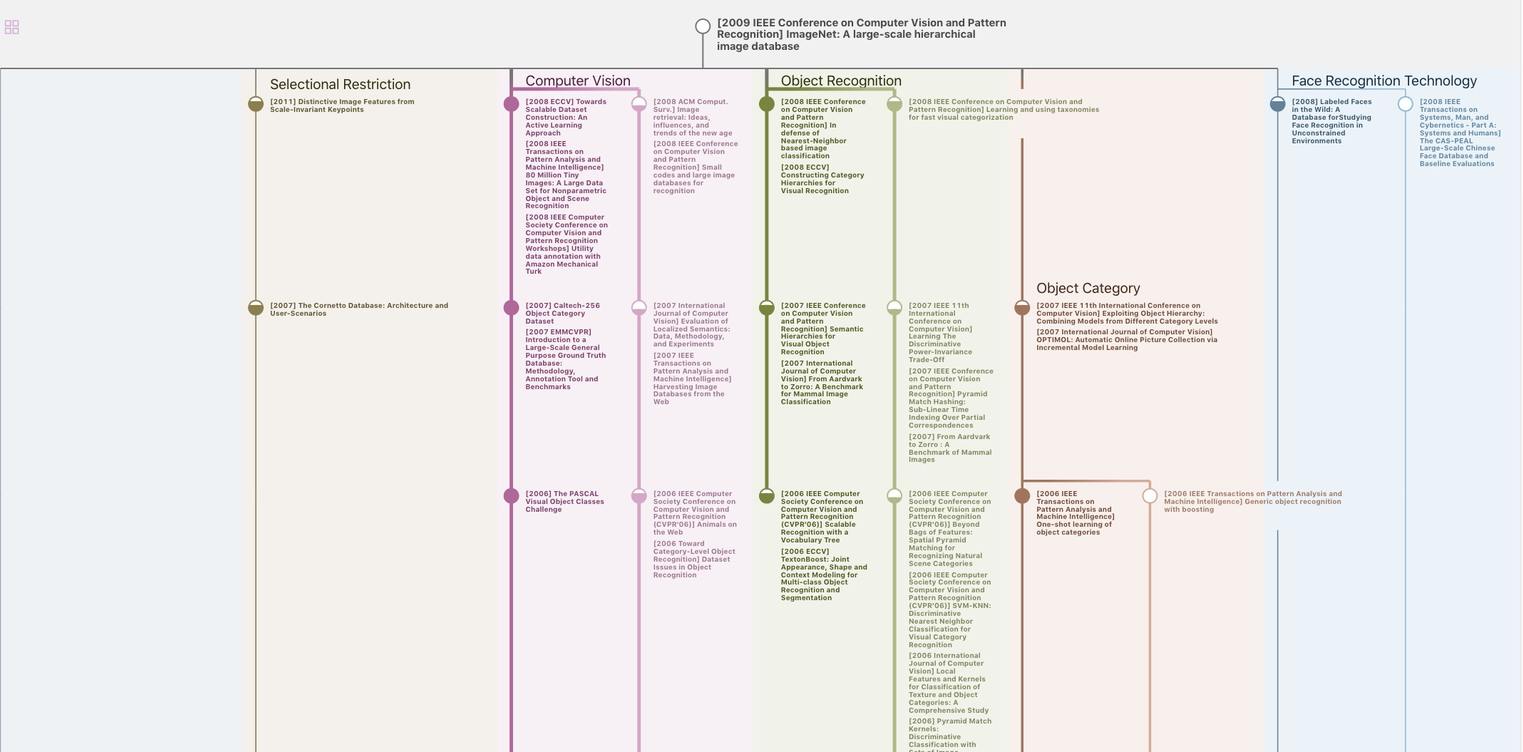
生成溯源树,研究论文发展脉络
Chat Paper
正在生成论文摘要