A Robust Dissimilarity Distribution Analytics With Laplace Distribution for Incipient Fault Detection
IEEE Transactions on Industrial Electronics(2023)
摘要
Incipient faults with small magnitudes are usually masked by the data outliers and ambient noise, and thus the robustness should be taken into consideration when developing monitoring models for them. In this study, a robust dissimilarity distribution analytics (RDDA) method is proposed for incipient industrial fault detection. The probabilistic model of the RDDA method is formulated with Laplace distribution, and thus it is more robust to the disturbance when compared with the Gaussian distribution based monitoring models. Using the variational inference, the maximum likelihood estimations of the latent variables and model parameters in the RDDA method can be derived. After that, a monitoring strategy is established based on the obtained results with both static and dynamic statistics, which are designed using the dissimilarity between the distributions of different datasets. Since the missing data problem is also considered, the proposed RDDA method is more suitable for practical industrial applications. The proposed method is applied to identify the operation status of a deaerator. Experimental results illustrate that the proposed method can be established using the historical data with missing values, and it can accurately detect the incipient faults with small magnitude.
更多查看译文
关键词
Dissimilarity distribution analytics,incipient fault,laplace distribution,variational inference
AI 理解论文
溯源树
样例
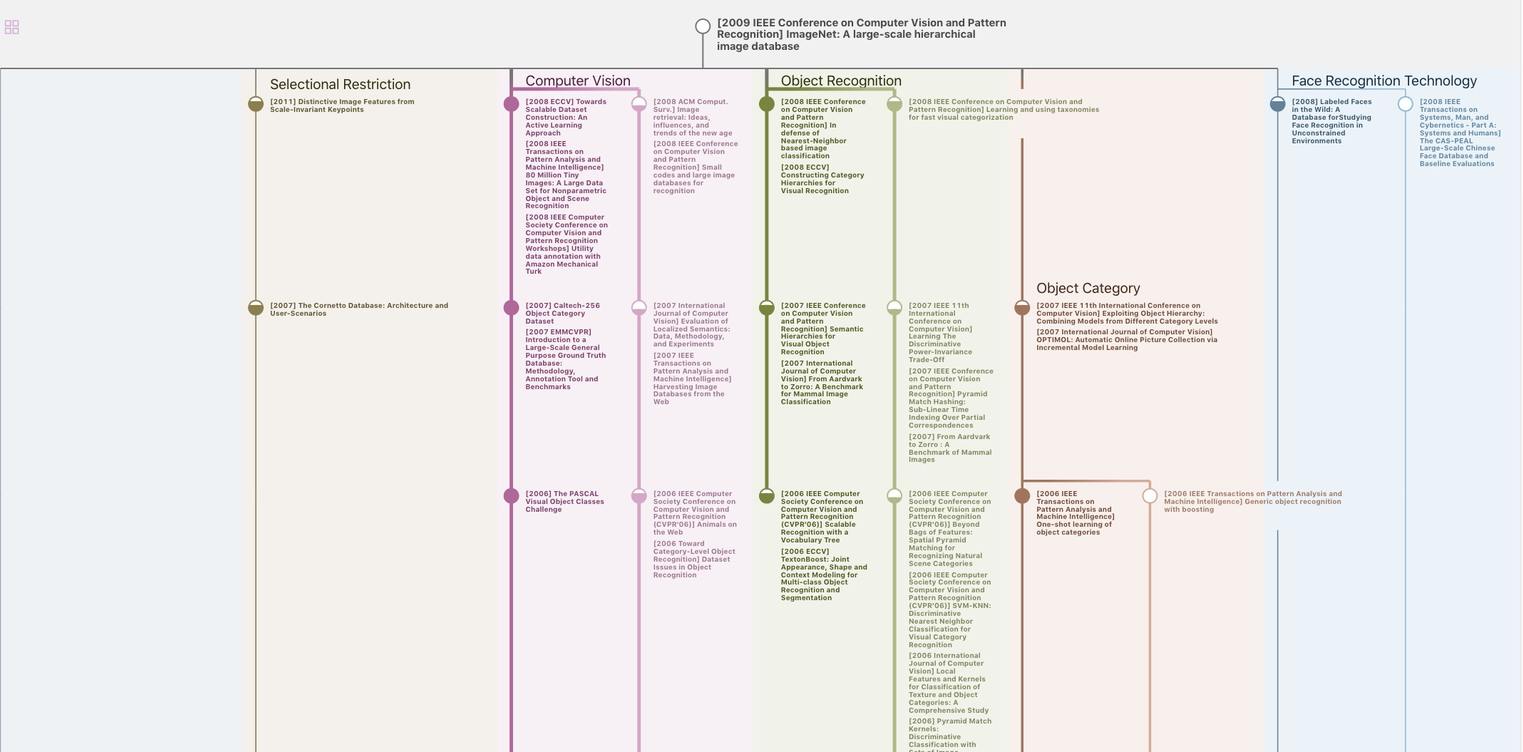
生成溯源树,研究论文发展脉络
Chat Paper
正在生成论文摘要