Spatiotemporal Relationship Cognitive Learning for Multirobot Air Combat
IEEE TRANSACTIONS ON COGNITIVE AND DEVELOPMENTAL SYSTEMS(2023)
摘要
cognition is crucial to learning-based multirobot systems (MRSs). As an advanced application of MRSs for fierce confrontation, the relationships among autonomous air combat robots inherently present complex time-varying characteristics, which makes relationship cognition even more difficult. However, previous studies have only focused on spatial cooperative relationships, thus ignoring the potential impact of the temporal dynamics of relationships on long-term cooperative behaviors. To tackle this drawback, we propose a novel multiagent deep reinforcement learning (MADRL)-based autonomous air combat robots collaboration algorithm, called spatiotemporal aerial robots relationship co-optimization (STARCO). STARCO formulates the complex dynamic relationship cognition problem into a spatiotemporal deep graph neural network (GNN) learning problem. On this basis, we overcome the limitations of previous methods, by accurately capturing the key spatiotemporal patterns from aggressive air combat, and enable global collaborative decision making through joint strategy optimization. An empirical study shows that STARCO outperforms several state-of-the-art MARL baselines by 24.6% in learning performance. We also demonstrate that STARCO is capable of evolving various cooperative strategies comparable to human expert knowledge.
更多查看译文
关键词
Air combat,graph neural network (GNN),multiagent deep reinforcement learning (MADRL),relationship robot,spatiotemporal
AI 理解论文
溯源树
样例
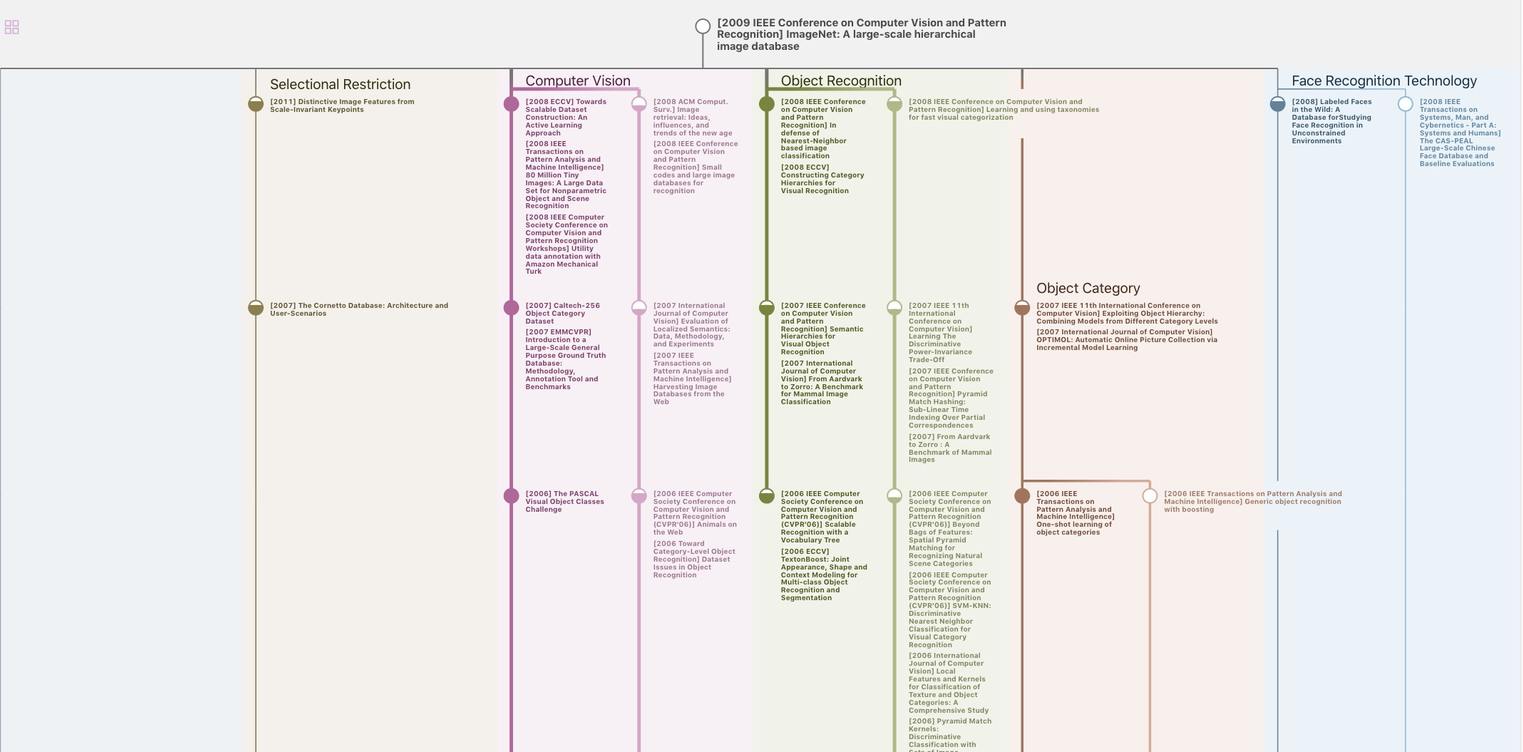
生成溯源树,研究论文发展脉络
Chat Paper
正在生成论文摘要