Power Allocation Strategy for Urban Rail HESS Based on Deep Reinforcement Learning Sequential Decision Optimization
IEEE Transactions on Transportation Electrification(2023)
摘要
A hybrid energy storage system (HESS) is adopted to tackle the traction network voltage fluctuation problem caused by high power and large energy demand during the starting and braking of urban rail trains. The system is composed of on- board ultracapacitors and ground lithium batteries, aiming to smooth out the power fluctuation to realize "peak-shaving and valley-filling." Based on deep reinforcement learning (DRL) online sequence decision, a dynamic power allocation strategy is proposed to improve the energy-saving and voltage stabilization of dc traction networks, as well as HESS life protection. Furthermore, to enhance the DRL's efficiency under time-varying operating conditions, an annealing bias-priority experience replay twin delayed deep deterministic policy gradient algorithm (A-TD3) is proposed to train the replay buffer in DRL. The online learning and optimization strategy is implemented via the mechanism of "trial and error" and "feedback" of the agent. RT-LAB semi-physical real-time simulation systems are adopted to verify the effectiveness of the proposed strategy. Compared with the traditional filtering algorithms and DRL algorithms, the results show that the proposed method converges faster and is more energy saving and stable while effectively protecting the energy storage components.
更多查看译文
关键词
Deep reinforcement learning (DRL),hybrid energy storage system (HESS),power dynamic allocation,regenerative braking energy,state of charge (SOC)
AI 理解论文
溯源树
样例
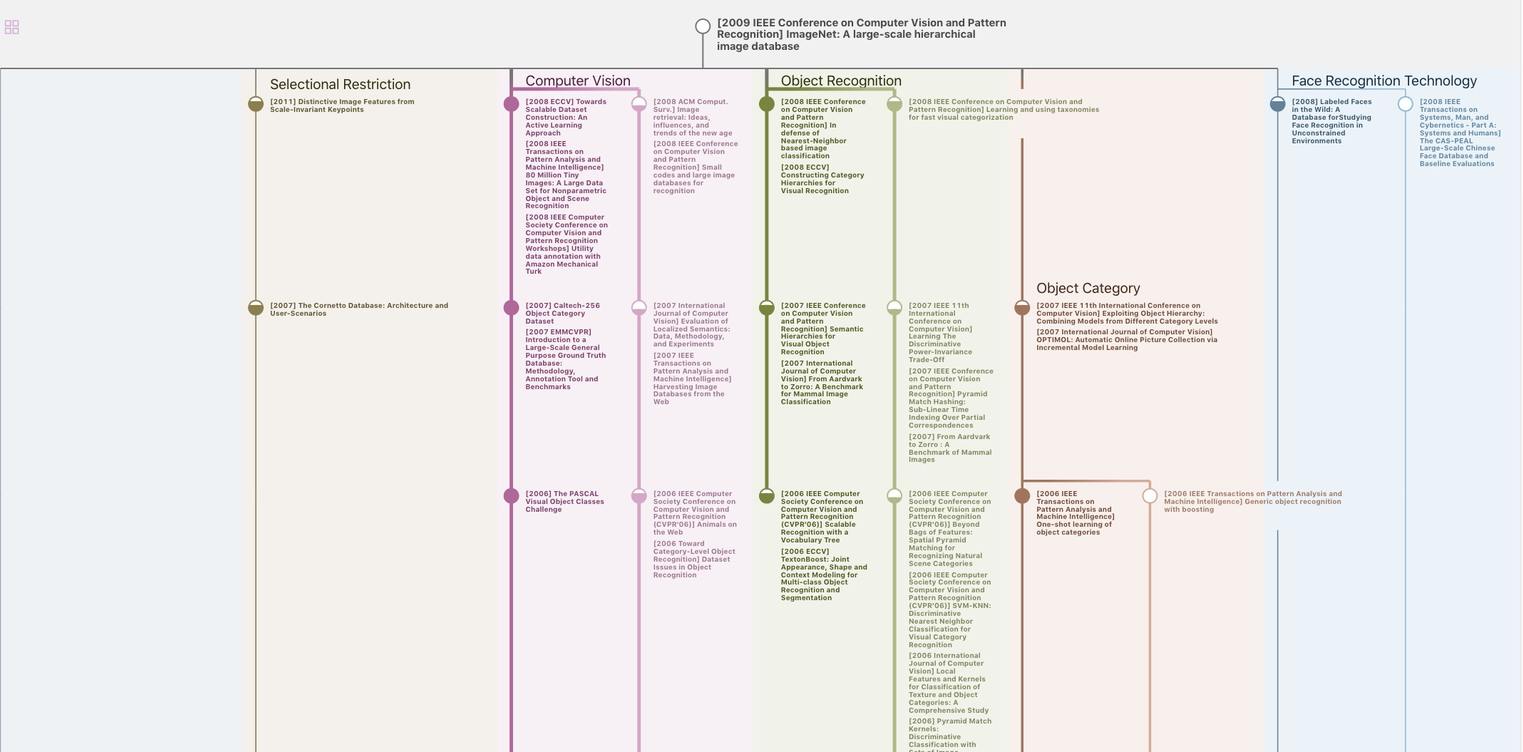
生成溯源树,研究论文发展脉络
Chat Paper
正在生成论文摘要