Adaptive Programmable Networks for In Materia Neuromorphic Computing
Research Square (Research Square)(2022)
Abstract
Abstract Nanomagnetic artificial spin-systems are ideal candidates for neuromorphic hardware. Their passive memory, state-dependent dynamics and nonlinear GHz spin-wave response provide powerful computation. However, any single physical reservoir must trade-off between performance metrics including nonlinearity and memory-capacity, with the compromise typically hard-coded. Here, we present three artificial spin-systems and show how tuning system geometry and dynamics defines computing performance. We engineer networks where each node is a high-dimensional physical reservoir, implementing parallel, deep and multilayer physical neural network architectures. This solves the issue of physical reservoir performance compromise, allowing a small suite of synergistic physical systems to address diverse tasks and provide a broad range of reprogrammable computationally-distinct configurations. These networks outperform any single reservoir across a broad taskset. Crucially, we move beyond reservoir computing to present a method for reconfigurably programming inter-layer network connections, enabling on-demand task optimised performance.
MoreTranslated text
Key words
materia neuromorphic computing,networks
AI Read Science
Must-Reading Tree
Example
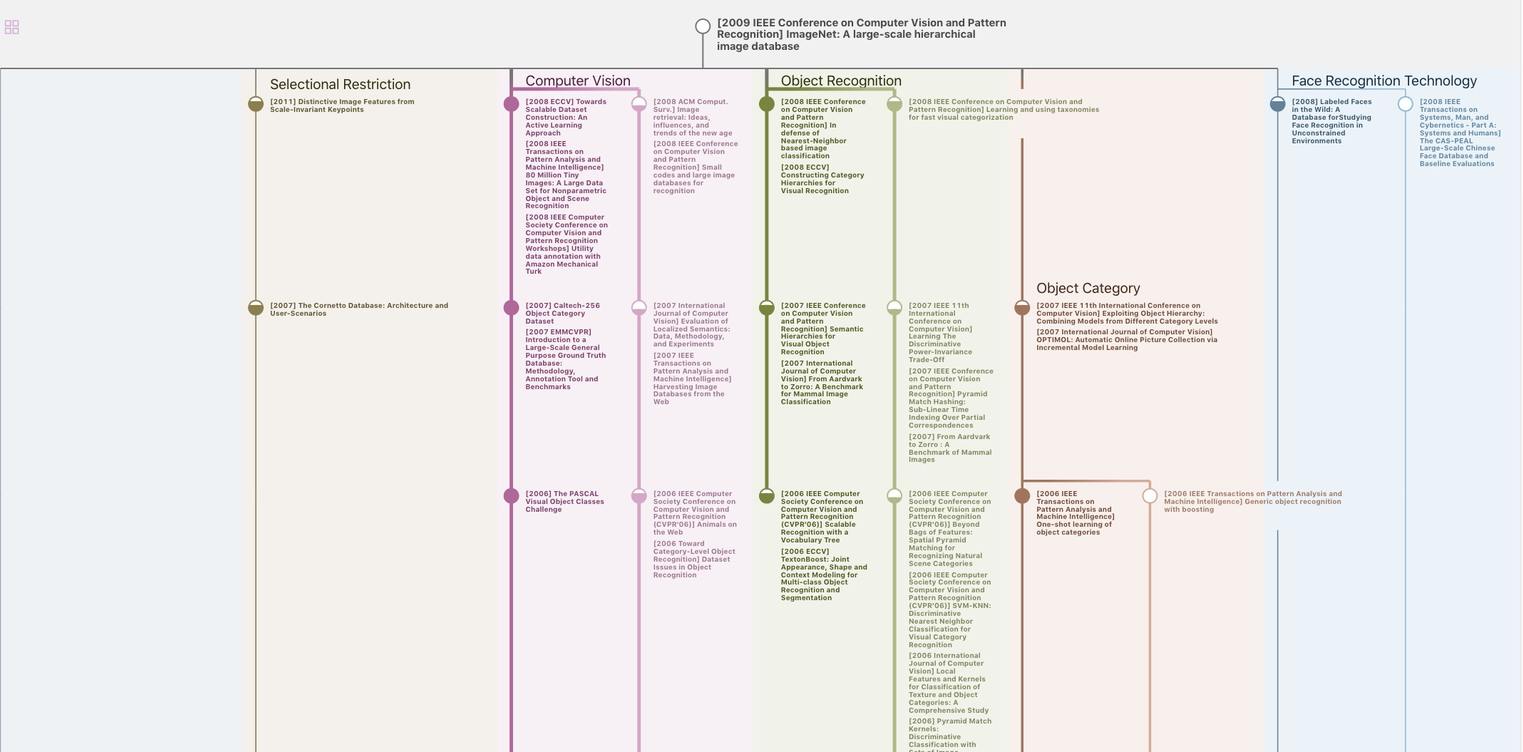
Generate MRT to find the research sequence of this paper
Chat Paper
Summary is being generated by the instructions you defined