Input Design for Regularized System Identification: Stationary Conditions and Sphere Preserving Algorithm
IEEE Transactions on Automatic Control(2023)
摘要
This article studies input design of kernel-based regularization methods for linear dynamical systems, which has been formulated as a nonconvex optimization problem with the criterion being a scalar measure of the posterior covariance of the Bayesian estimate, subject to a spherical constraint on the input. The nonconvex nature of such input design problems poses significant challenges in deriving optimality conditions and efficient numerical algorithms. In this work, we first derive a sufficient condition for guaranteeing that a stationary point of the regularized input design problem is a global minimum. Next, we propose a spherical constraint preserving (SCP) algorithm to efficiently reach a stationary point of the design problem. Numerical simulation results show that the SCP algorithm finds the global minimum of the original design problem for all simulated cases and its average computational time is only approximately one tenth of that of the algorithms for previous methods.
更多查看译文
关键词
Input design,regularized system identification,spherical constraint preserving (SCP) algorithm,stationary points
AI 理解论文
溯源树
样例
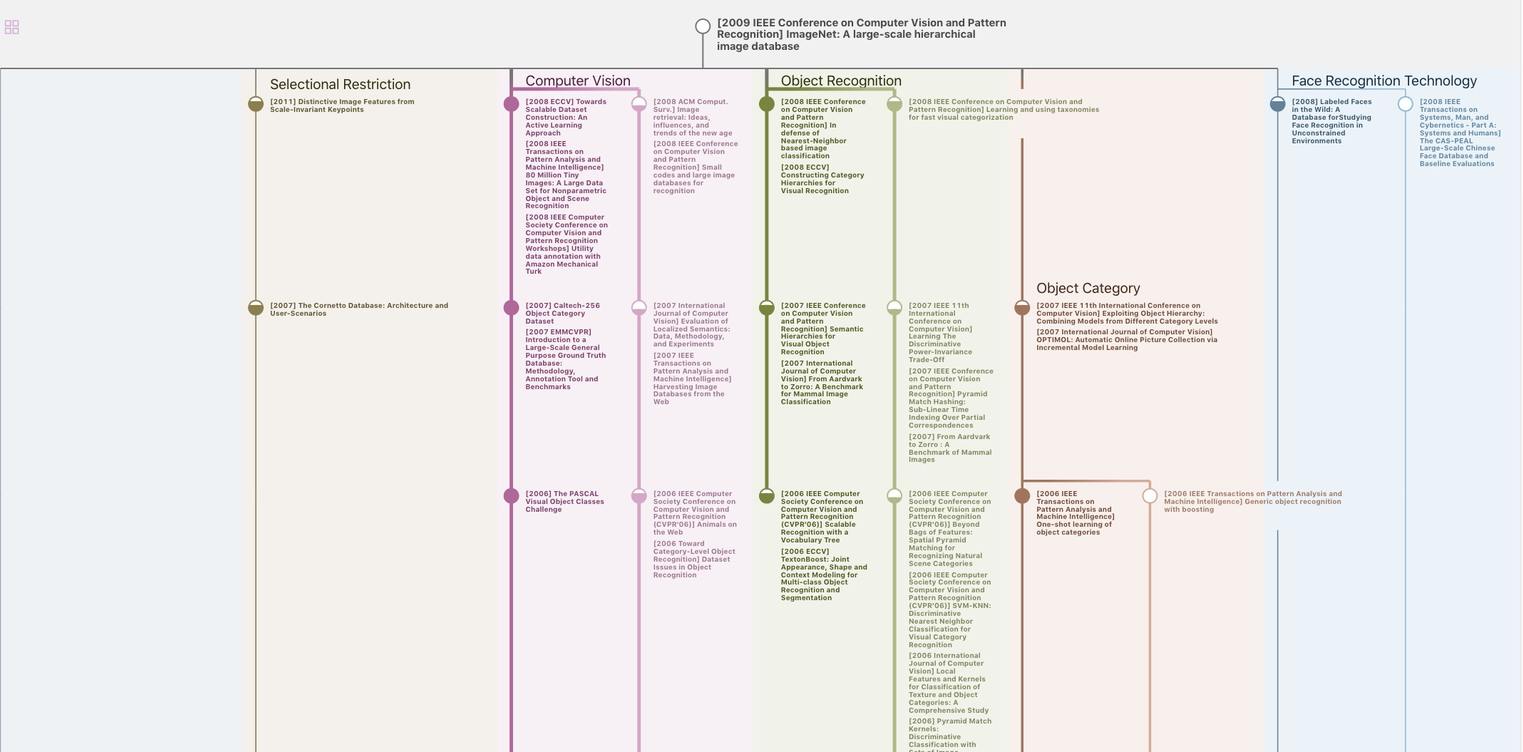
生成溯源树,研究论文发展脉络
Chat Paper
正在生成论文摘要