CVCNet: Learning Cost Volume Compression for Efficient Stereo Matching
IEEE TRANSACTIONS ON MULTIMEDIA(2023)
Abstract
State-of-the-art deep learning based stereo matching algorithms usually rely on full-size cost volumes for highly accurate disparity estimation. The full-size cost volume processes all possible disparity candidates equally without considering their different matching uncertainties. Consequently, considerable redundant computation is involved on those candidates with very low matching uncertainties, making these methods difficult to be deployed in real-time applications. To tackle this problem, we propose CVCNet featuring an adaptive disparity range prediction module (ADR) and a disparity refinement module (DRM). The ADR adaptively predicts pixel-wise disparity range to discard the "unimportant" disparity candidates. It enables our network to obtain a compressed cost volume. Besides, the DRM improves disparity range prediction and refines the predicted disparity map. With the proposed modules, our CVCNet learns to build a compressed cost volume to achieve efficient disparity estimation. Experimental results on the KITTI and SceneFlow datasets show that our method achieves state-of-the-art performance, and runs at a significant order of magnitude faster speed than existing 3D CNN based methods. Particularly, our method ranks 1st on the KITTI 2012 and KITTI 2015 benchmarks among all published methods with running time shorter than 100 ms.
MoreTranslated text
Key words
Computer vision,image matching,machine vision,robot vision systems,stereo vision
AI Read Science
Must-Reading Tree
Example
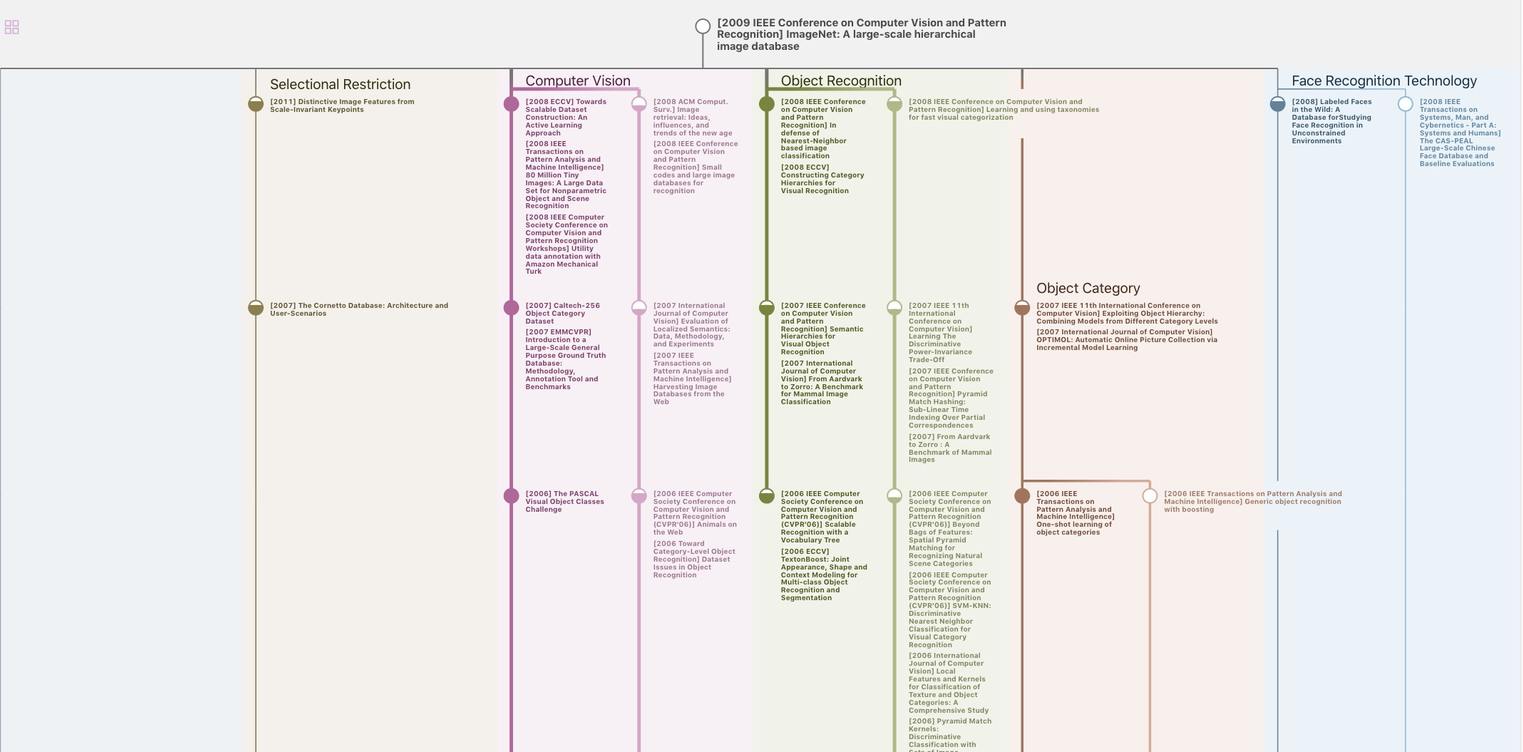
Generate MRT to find the research sequence of this paper
Chat Paper
Summary is being generated by the instructions you defined