Development of a new approach for rapid identification and classification of uranium ore concentrate powders using textural and spectroscopy signatures
SSRN Electronic Journal(2023)
摘要
Recently, a concept for a new approach for rapid identification of uranium ore concentrate (UOC) powders using colour, textural and spectroscopy signatures was developed using a sample dataset consisting of 79 industrial uranium ore concentrate powders produced by different production routes at different facilities. The samples were first grouped into six different predefined colour categories using a colour-based classification model previously developed. In this study, a machine learning approach was used to develop supervised texture- and spectroscopy-based classification models for each colour category of uranium ore concentrates. Scanning electron microscopy images of the UOC samples were collected at 100x, 250x, 500x/1000x magnifications. A total of 524 texture features describing sample morphology were extracted from each image using First Order Statistics (FOS), Grey Level Co-Occurrence Matrix (GLCM), Grey Level Run Length Matrix (GLRLM), Grey Level Size Zone Matrix (GLSZM), Locally Binary Pattern (LBP) and Angle Measure Technique (AMT) textural feature extraction algorithms. Hyperspectral images of the samples were collected in the wavelength range 900-1700 nm and the mean spectrum of each sample was calculated, giving the spectral features of the UOCs. The texture and spectral features were used as input to the machine learning approach which consisted of a feature reduction step to identify discriminatory features and a classification step to provide the texture- and spectroscopy-based models. An average prediction accuracy of 82% and 97% were obtained for the texture- and spectral-based classification models, respectively, on hold-out test sets. The feature reduction step identified two new nuclear forensics signatures, consisting of 3-7 texture (morphological) characteristics and 2-6 characteristic wavelengths, respectively. This new approach enables rapid classification of unknown uranium ore concentrate powders using only their textural and hyperspectral images. By combining the classification results from the two models, the most probable production process and chemical composition can be identified, and the provenience of the seized sample can be narrowed down to a few matching possibilities. Moreover, after the image acquisition, the models can provide results in less than 2 min speeding up the investigation procedure, a key factor when a nuclear security event occurs.
更多查看译文
关键词
Nuclear forensics,Uranium ore concentrates,Colour analysis,Image texture analysis,Hyperspectral image analysis,Machine learning
AI 理解论文
溯源树
样例
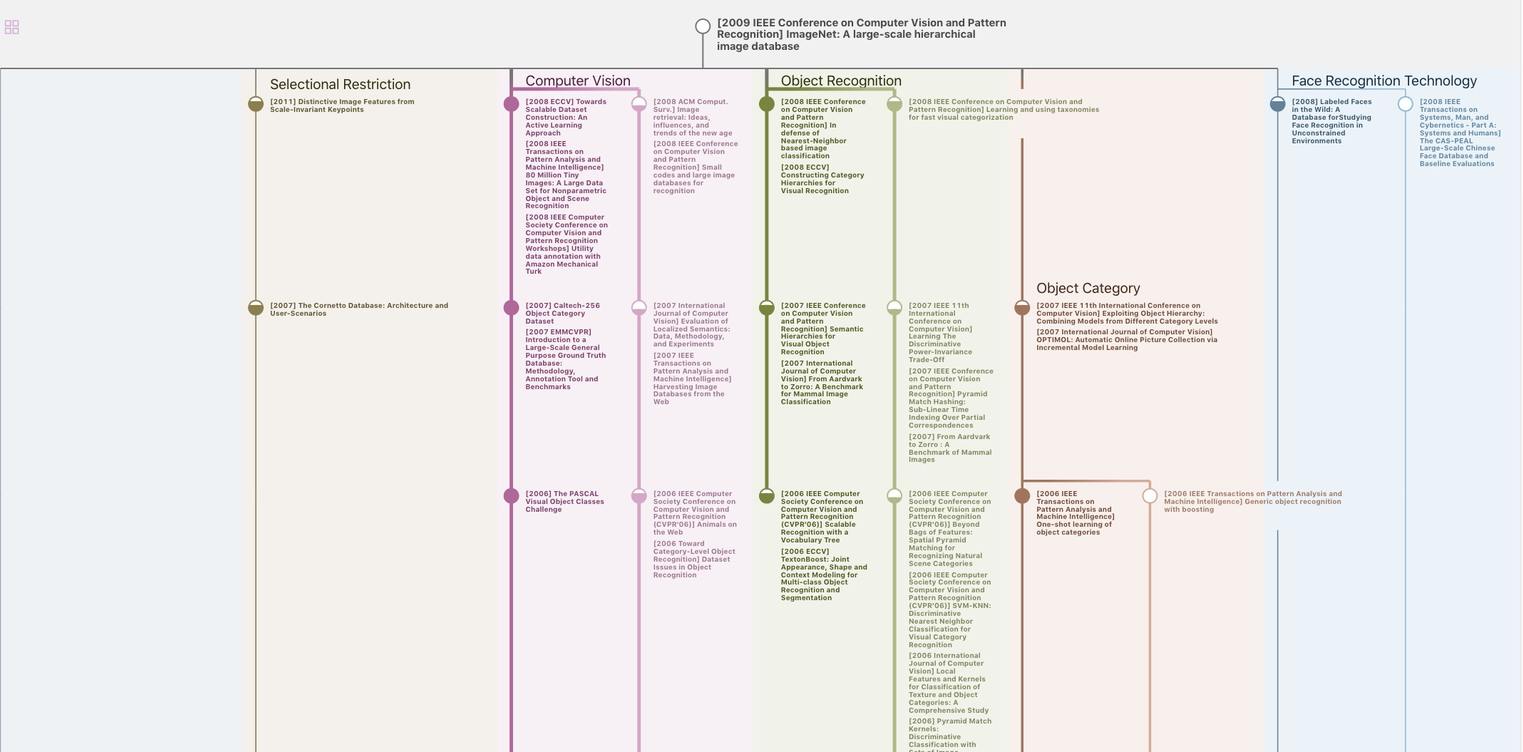
生成溯源树,研究论文发展脉络
Chat Paper
正在生成论文摘要