CEM Radiomics for Distinguishing Lesion from Background Parenchymal Enhancement in Patients with Invasive Breast Cancer
18th International Symposium on Medical Information Processing and Analysis(2023)
Abstract
In this IRB approved retrospective study 41 women with biopsy-proven invasive breast cancers (IBC) were imaged using contrast-enhanced mammography (CEM), prior to any treatment. Size-matched regions of interest (ROIs) were manually contoured by an experienced breast radiologist on the CEM capturing the breast lesion and breast parenchymal enhancement (BPE), respectively. Radiomics analysis was performed using LifEx software and 109 radiomics metrics spanning 6 different texture families were extracted from each ROI. Predictive models of lesion malignancy were developed using multiple classifiers and used to subclassify breast cancers based on their hormone receptor status. The 10-fold cross validation was used to construct the decision classifier and performance was assessed. CEM radiomics models based on Random Forest, Real Adaboost, and ElasticNet classifiers achieved an AUC of 0.83, 0.82 and 0.74, respectively in discriminating malignant breast lesions from varying amounts of BPE. Accounting for the varying levels of BPE, revealed a reduction in AUC-based prediction of lesion vs. BPE as the qualitative assessment of BPE increased from minimal to moderate (AUCs of 0.89 vs 0.74). Further analyses of the IBC based on their hormone receptor status showed that triple negative breast lesions showed statistically significant differences in multiple radiomics metrics compared to ER+ PR+ HER2- and HER2+. The predicted probability of the radiomics model was significantly different across three receptor-based subtypes and between high and low nuclear grade breast cancers. CEM Radiomics demonstrated good discrimination (AUC>0.8) of malignant breast lesions despite varying BPE levels and supports breast lesion subtyping.
MoreTranslated text
Key words
Breast cancer,CEM,Radiomics,Lesion characterization,Quantitative imaging
AI Read Science
Must-Reading Tree
Example
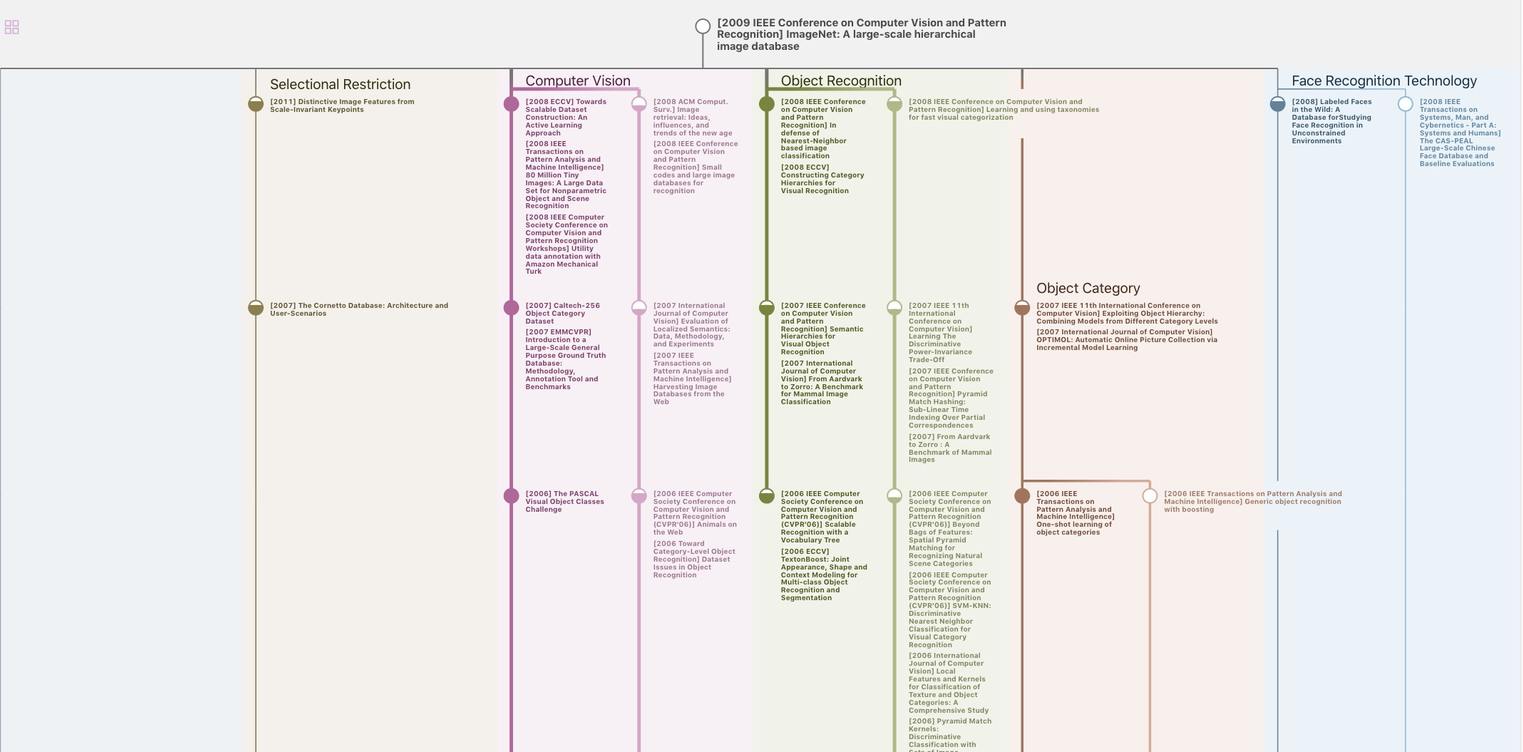
Generate MRT to find the research sequence of this paper
Chat Paper
Summary is being generated by the instructions you defined