Body location embedded 3D U-Net (BLE-U-Net) for ovarian cancer ascites segmentation on CT scans
18th International Symposium on Medical Information Processing and Analysis(2023)
Abstract
Ascites is often regarded as the hallmark of advanced ovarian cancer, which is the most lethal gynecologic malignancy. Ascites segmentation contributes to track the progress of ovarian cancer development by providing accurate ascites measurement, which can effectively guide subsequent treatment and potentially reduce the mortality. Segmentation of ascites is challenging due to the presence of iso-intense fluids such as bile, urine, etc., near the ascites region. In this work we propose a novel 3D U-Net segmentation method called body location embedded U-Net (BLE-U-Net) that integrates anatomical location information with the segmentation process. BLE-U-Net incorporates body part regression to predict the approximate anatomical location of each CT slice along the z-axis. The regression scores are discretized to indicate different body regions and embedded into a modified 3D U-Net to improve the ascites segmentation. Twenty contrast-enhanced body CT scans were used to evaluate the proposed method. Dice coefficients of 38 +/- 10 and 65 +/- 06 were achieved for a conventional 3D U-Net and BLE-U-Net, respectively (with t-test p <0.05). Volumes of segmented ascites were 0.51 +/- 0.74 and 0.57 +/- 0.85 liters for each method where the ground-truth volume was 0.58 +/- 0.84 liters. These results suggest that the embedded location information is the key factor to improve the ascites segmentation, which could potentially benefit ovarian cancer diagnosis and treatment.
MoreTranslated text
Key words
Ovarian cancer, Ascites, Location embedded 3D U-Net, Body part regressor
AI Read Science
Must-Reading Tree
Example
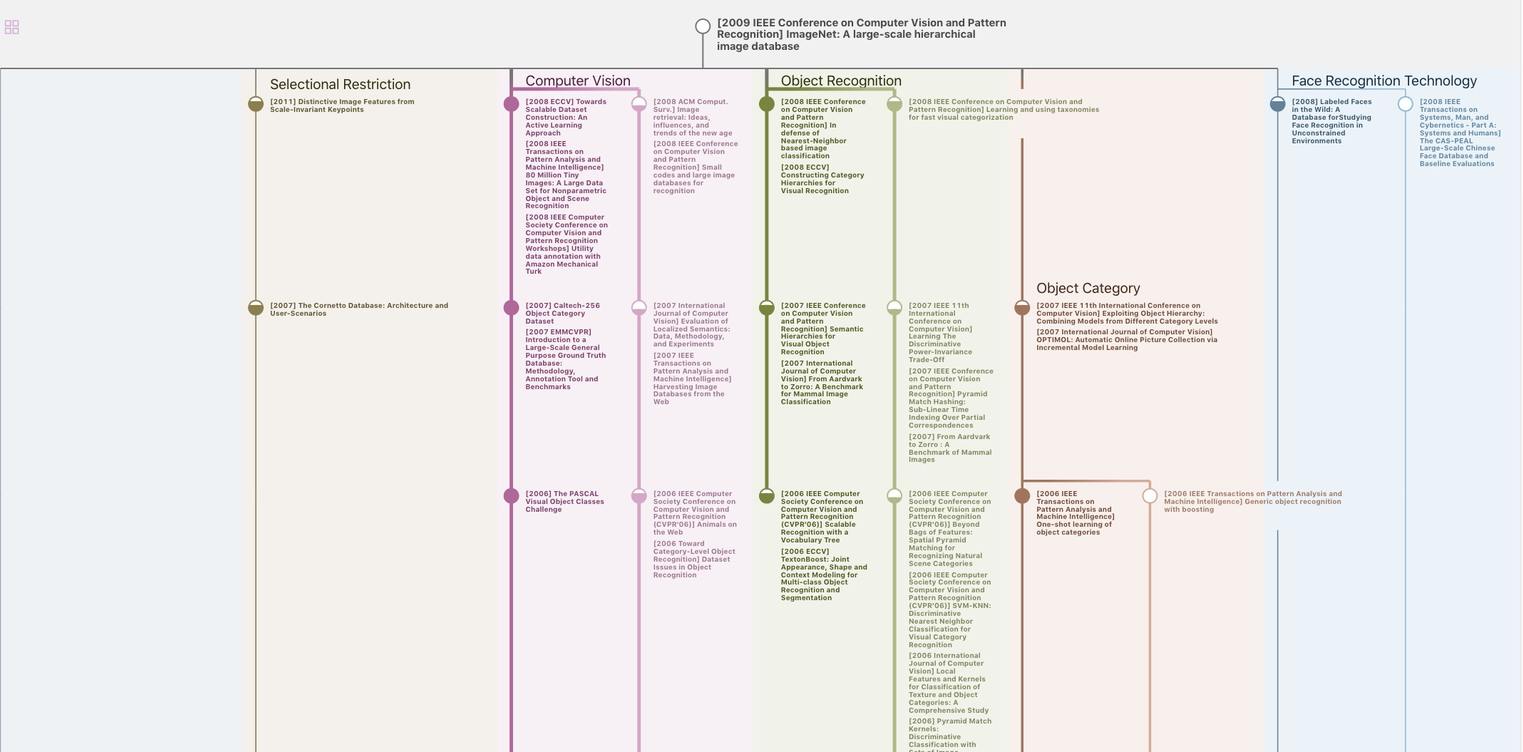
Generate MRT to find the research sequence of this paper
Chat Paper
Summary is being generated by the instructions you defined