Non-Invasive Bio-Signal Data Classification Of Psychiatric Mood Disorders Using Modified CNN and VGG16
Uluslararası Muhendislik Arastirma ve Gelistirme Dergisi(2023)
摘要
In this study, the aim is to develop an ensemble machine learning (ML) based deep learning (DL) model classifiers to detect and compare one type of major psychiatric disorders of mood disorders (Depressive and Bipolar disorders) using Electroencephalography (EEG). The diverse and multiple non-invasive biosignals were collected retrospectively according to the granted ethical permission. The experimental part is consisted from three main parts. First part is the data collection&development, the second part is data transformation and augmentation via Spectrogram image conversion process and online Keras data augmentation part, respectively. The third and final part is to fed these image dataset into modified Convolutional Neural Network (CNN) and VGG16 models for training and testing parts to detect, compare and discriminate mood disorders types in detail with a specific healthy group. As the performance evaluation background of the mood disorder classification models, confusion matrices and receiver operating characteristics (ROC) curves were used and finally, the accuracy achieved by CNN model was 88% and VGG16 model was %90, which is an improvement of 10% compared to the previous studies in literature. Therefore, our system can help clinicians and researchers to manage, diagnose and prognosis of the mental health of people.
更多查看译文
关键词
psychiatric mood disorders,modified cnn,non-invasive,bio-signal
AI 理解论文
溯源树
样例
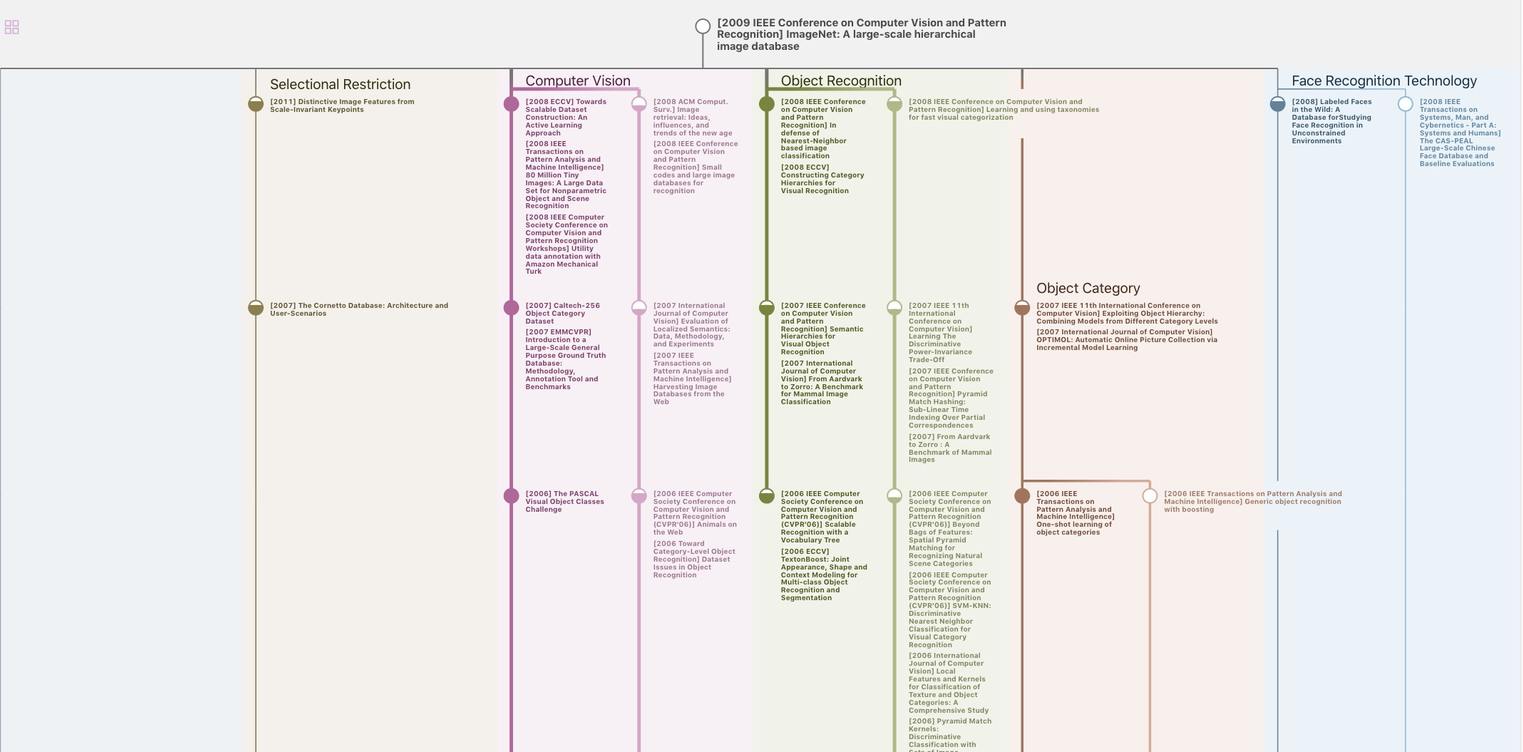
生成溯源树,研究论文发展脉络
Chat Paper
正在生成论文摘要