Secure Federated Learning in Quantum Autonomous Vehicular Networks
IEEE Network(2023)
摘要
With rapid developments of artificial intelligence (AI) and wireless communication technologies, autonomous vehicular networks (AVNs) have emerged to provide convenient, comfortable, and safe driving services. Especially, due to the provable and unconditional security, quantum communication technology is increasingly being applied to promote the security of data delivery in AVNs, which forms the promising quantum AVNs (QAVNs). Federated learning (FL) is essential in QAVNs to implement intelligent and autonomous driving, where extensive distributed data is utilized to cooperatively train AI models, preserve user privacy, and reduce resource consumption. However, the FL unavoidably faces several possible privacy and security problems as a result of the intricate interconnections between several autonomous vehicles or infrastructures. In this article, we investigate the security and privacy of FL in QAVNs. Specifically, we first establish the system architecture, including quantum key distribution protocol, space-air-ground integrated network (SAGIN)-enabled QAVNs model, and FL framework in QAVNs. Furthermore, we describe the privacy and security threats to FL in QAVNs, i.e., inference attack, eavesdropping attack, and sybil attack. Following that, we give a case study for designing a secure FL scheme using the local differential privacy mechanism and the homomorphic encryption mechanism. Finally, we suggest several potential future research directions in QAVNs.
更多查看译文
关键词
quantum autonomous vehicular networks,federated learning
AI 理解论文
溯源树
样例
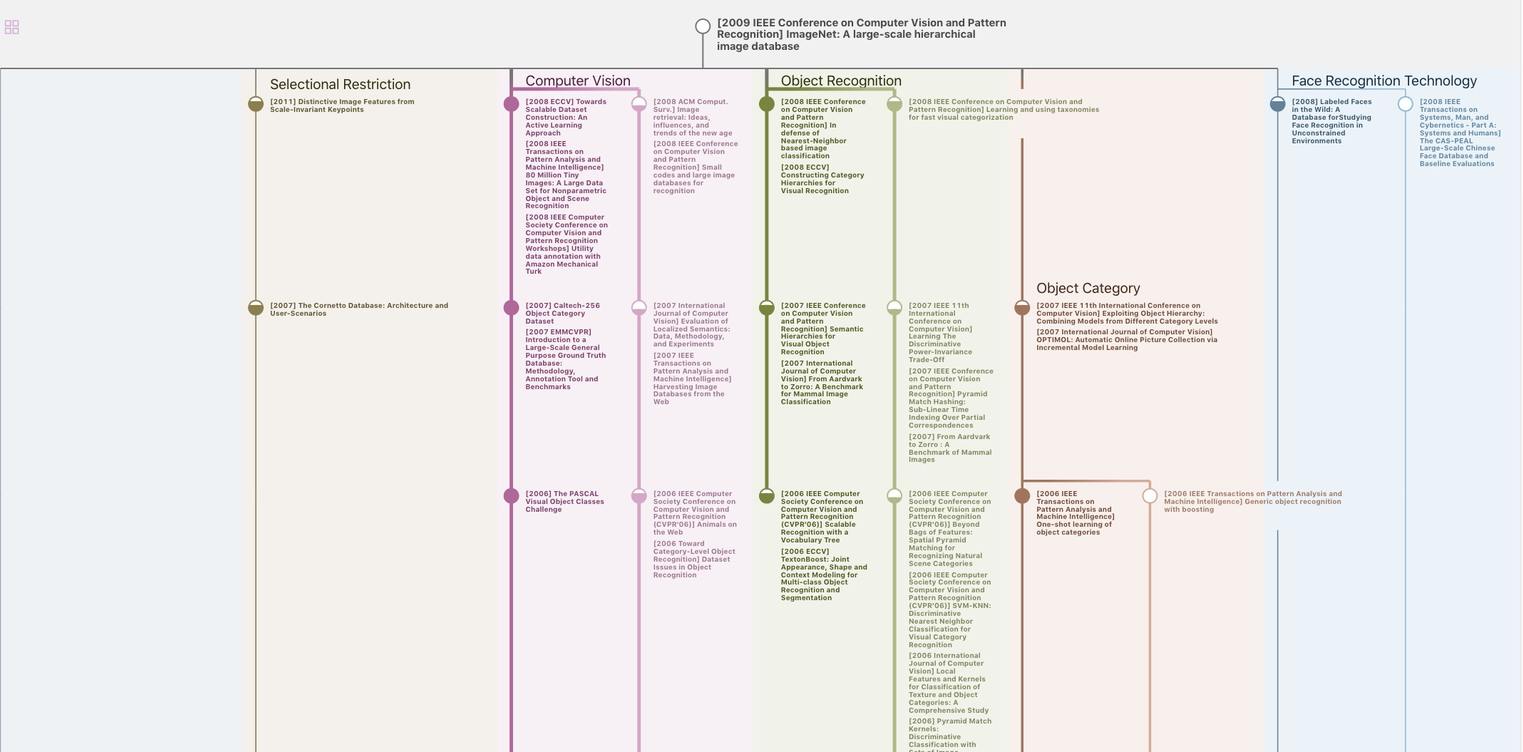
生成溯源树,研究论文发展脉络
Chat Paper
正在生成论文摘要