527. Antimicrobial stewardship for empirical treatment of bloodstream infection using machine learning clinical decision support
Open Forum Infectious Diseases(2022)
Abstract
Abstract Background Undertreatment of sepsis is associated with increased mortality, but only 12 to 18% of people require broad-spectrum Gram negative or positive coverage. Unnecessary use of broad-spectrum treatments represents a modifiable driver for antimicrobial resistance (AMR). Here, we test the hypothesis that a recommendation algorithm built on ML models trained on electronic health record data can safely reduce the spectrum of activity for empiric antibiotic treatments relative to clinicians for patients with community-onset sepsis. Methods We included 29,508 people with a community-onset Adult Sepsis Event in the Mass General Brigham health system. We split the data into a training and test set by time, June 2016 to June 2020 and July 2020 to February 2021, respectively. We extracted 1,200 predictors and windowed time-varying features into non-overlapping windows. We used logistic regression, random forest and XGBoost to predict pathogen, and then predict AMR to treatments relevant to the most likely pathogen. We used dynamic thresholding to convert probabilities into AMR phenotypes and then chose the antibiotic of the narrowest spectrum among those susceptible. Outcomes were the percentage of decisions that were inappropriately narrow versus broad spectrum. We compared model performance to that of clinicians. Figure 1:Analytic pipeline Schematic of machine learning architecture, thresholding algorithm and calculation of outcomes. Results The highest performing models for pathogen prediction were XGBoost, with area under the receiver operator curve (AUROC) statistics ranging from 0.60 for Staphylococcus spp. to 0.68 for Proteus spp. Random forests performed best for AMR prediction, with AUROCs ranging from 0.59 for ampicillin-sulbactam to 0.74 for penicillin. The model utilized broad-spectrum therapy in 92.4% (92.2% - 92.7%) of patients compared to 89.3% (88.9% - 89.6%) for clinicians, a relative increase of 3.5%, but chose inappropriately narrow treatments in 7.3% (7.1% - 7.6%) of patients, compared to 9.4% (9.1% - 9.6%) for providers, a 29% reduction. Figure 2:Model performance for pathogen prediction AUROCs for prediction of pathogen groupings for patients with community-onset sepsis. All models are XGBoost. Figure 3:Model performance for AMR prediction AUROCs for prediction of antimicrobial resistance based on most likely predicted pathogens for patients with community-onset sepsis. All models are random forests. Figure 4:Post-hoc outcome analysis Comparison of rates of inappropriately narrow antibiotic treatment (IAT) and use of broad spectrum therapy for the machine learning decision algorithm versus clinicians for patients with community onset sepsis. Conclusion An ML decision support tool for antibiotic prescription in community-onset sepsis did not result in decreased broad spectrum use but was able to reduce inappropriately narrow treatment. Work is underway to refine feature sets, improve the thresholding algorithm and better account for time-varying confounders. Disclosures Sanjat Kanjilal, MD, MPH, GlaxoSmithKline: Advisor/Consultant|Roche Diagnostics: Honoraria David Sontag, PhD, Adobe: Grant/Research Support|ASAPP: Advisor/Consultant|Cureai Health: Stocks/Bonds|Facebook: Grant/Research Support|Google: Grant/Research Support|IBM: Grant/Research Support|SAP: Grant/Research Support|Takeda: Grant/Research Support.
MoreTranslated text
Key words
antimicrobial stewardship,clinical decision support,bloodstream infection
AI Read Science
Must-Reading Tree
Example
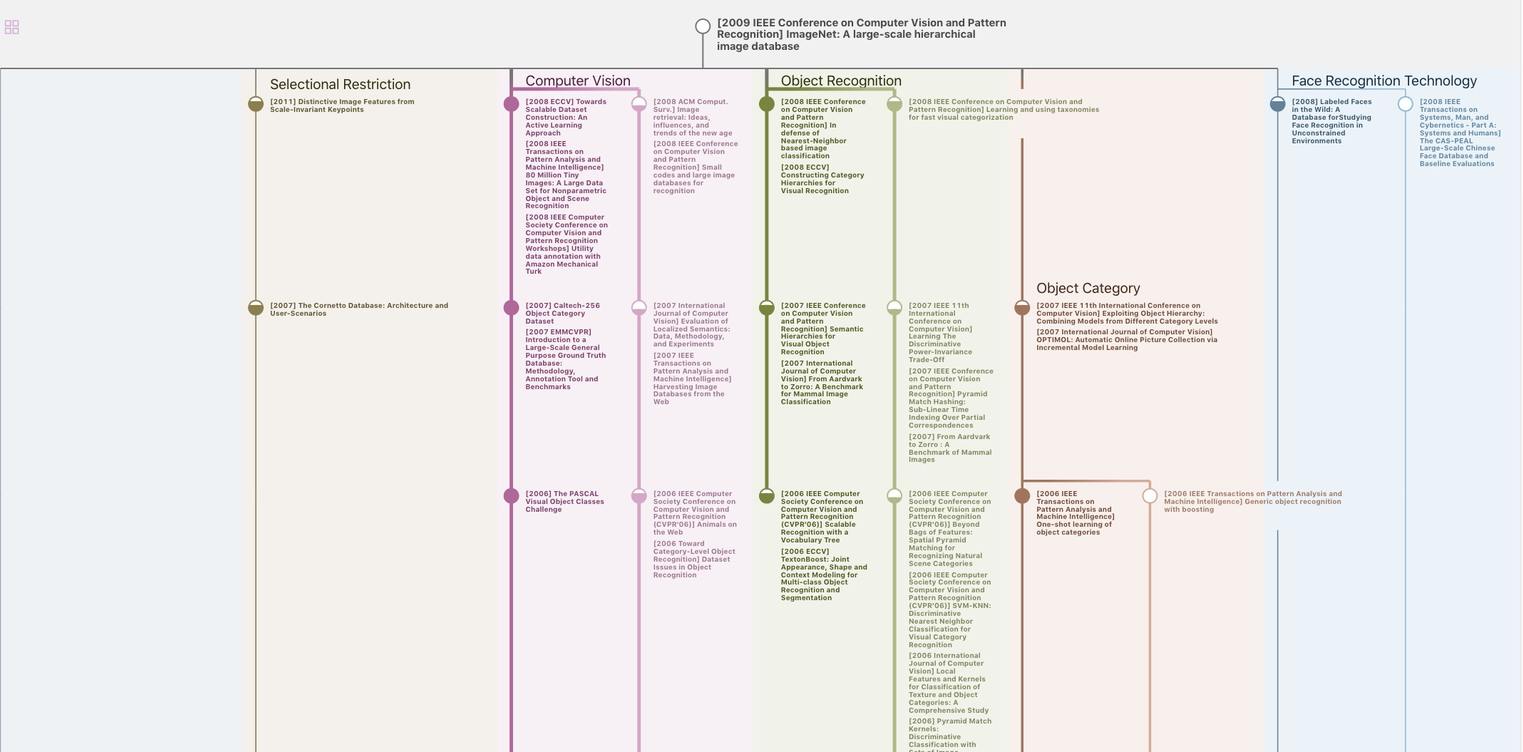
Generate MRT to find the research sequence of this paper
Chat Paper
Summary is being generated by the instructions you defined