A novel machine learning model for predicting late supplier deliveries of low-volume-high-variety products with application in a German machinery industry
Supply Chain Analytics(2023)
Abstract
Although Machine Learning (ML) in supply chain management (SCM) has become a popular topic, predictive uses of ML in SCM remain an understudied area. A specific area that needs further attention is the prediction of late deliveries by suppliers. Recent approaches showed promising results but remained limited in their use of classification algorithms and struggled with the curse of dimensionality, making them less applicable to low-volume-high-variety production settings. In this paper, we show that a prediction model using a regression algorithm is capable to predict the severity of late deliveries of suppliers in a representative case study of a low-volume-high-variety machinery manufacturer. Here, a detailed understanding of the manufacturer’s procurement process is built, relevant features are identified, and different ML algorithms are compared. In detail, our approach provides three key contributions: First, we develop an ML-based regression model predicting the severity of late deliveries by suppliers. Second, we demonstrate that prediction within the earlier phases of the purchasing process is possible. Third, we show that there is no need to reduce the dimensionality of high-dimensional input features. Nevertheless, our approach has scope for improvement. The inclusion of information such as component identifiers may improve the prediction quality.
MoreTranslated text
Key words
Supply chain management,Prediction methods,Machine learning,Supervised Learning,Regression analysis,Data analysis
AI Read Science
Must-Reading Tree
Example
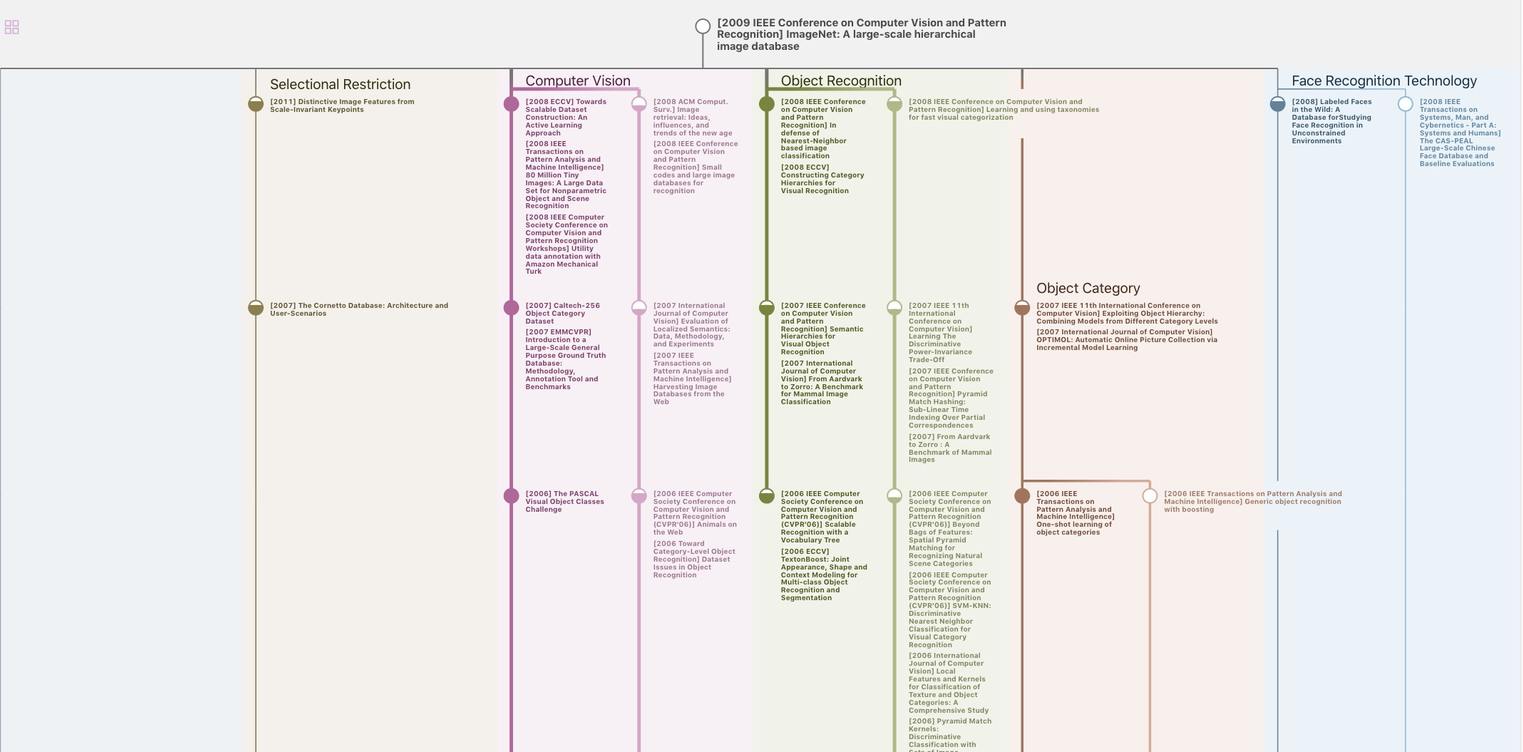
Generate MRT to find the research sequence of this paper
Chat Paper
Summary is being generated by the instructions you defined