Training Approach in Kernel-based Fuzzy Machine Learning for Sugarcane Crop Type Mapping
crossref(2022)
Machine learning algorithms using statistical information from training data are not able to handle high levels of heterogeneity within the sugarcane ratoon/plant fields due to gradual differences in their germination process. To handle heterogeneity within a class, novel individual samples as mean with kernel-based fuzzy Modified Possibilistic c-means approach was proposed, to map ratoon. plant and ratoon diseased fields. The results show that the KMOD kernel outperformed with 0.013 mean membership difference and 0.00007 variance for ratoon and 0.044 mean membership difference and 0.00136 variance for plant with MSAVI2 temporal indices database. The ISM-based training approach provided better results than the mean training approach having a variance of 0.00136 with MSAVI2 temporal indices database. The study shows that the MSAVI2 yields better results in the case of Ratoon mapping, but for mapping sugarcane Plants CBSI-MSAVI2 performed best.
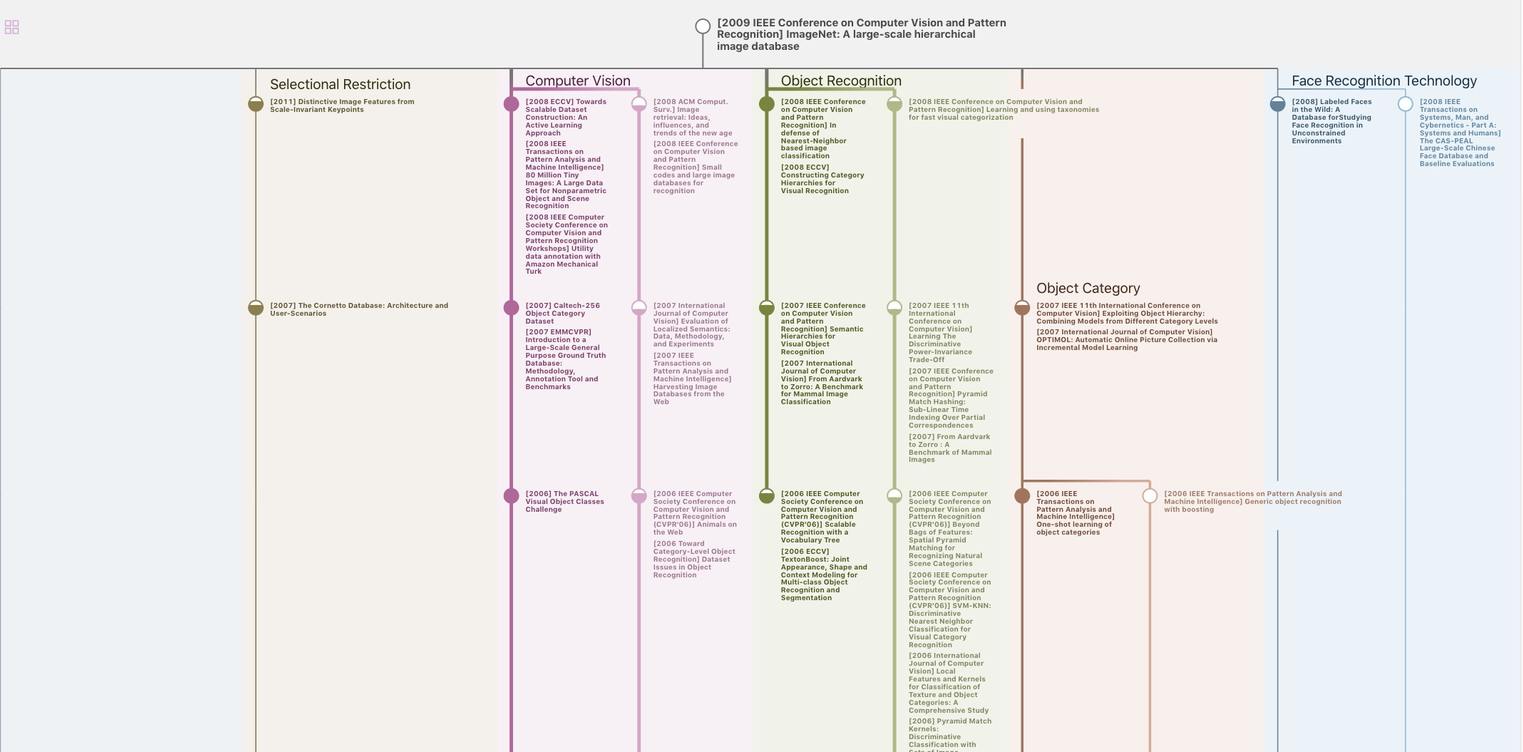