Separate but interacting sources drive serial dependencies in temporal motor and perception tasks
Journal of Vision(2022)
Abstract
We can flawlessly navigate in and interact with our dynamic environment, despite our noise-tainted perception and motor action executions. One way to optimize perception and behavior is to learn from previous encounters, known as serial dependencies. In the time domain, serial dependencies have been found for perception (the previous percept affects the current percept) and for interval reproductions (the previous reproduction affects the current reproduction). Perceived durations and timed actions are generally studied as different processes stemming from different sources, and the importance of motor actions for the perception of duration has only recently moved into focus of timing research. However, it remains unclear whether temporal processing for perception and action rely on a shared resource or on separate modules that calibrate each other. In the current study, we looked at serial dependencies within and between a motor interval reproduction task and a visual duration discrimination task. Tasks alternate on a trial-by-trial basis, such that a motor-trial is followed by a perception trial (M-P/P-M), or a motor/perception trial is followed by a trial of the same task (M-M and P-P). If the known serial dependencies within tasks stem from separate sources, we would not expect any effects of a previous motor (perceptual) trial on the current perceptual (motor) trial. If, however, serial dependencies stem from the same source, we would expect serial dependencies between tasks comparable to those within task. Motor reproductions were affected by previous perceptual judgements and vice versa (M-P/P-M), but not as strongly as in the within-task conditions (M-M, P-P). In conclusion, our data provide evidence for separate but interacting systems processing durations for perception and for action.
MoreTranslated text
Key words
temporal motor,serial dependencies,perception
AI Read Science
Must-Reading Tree
Example
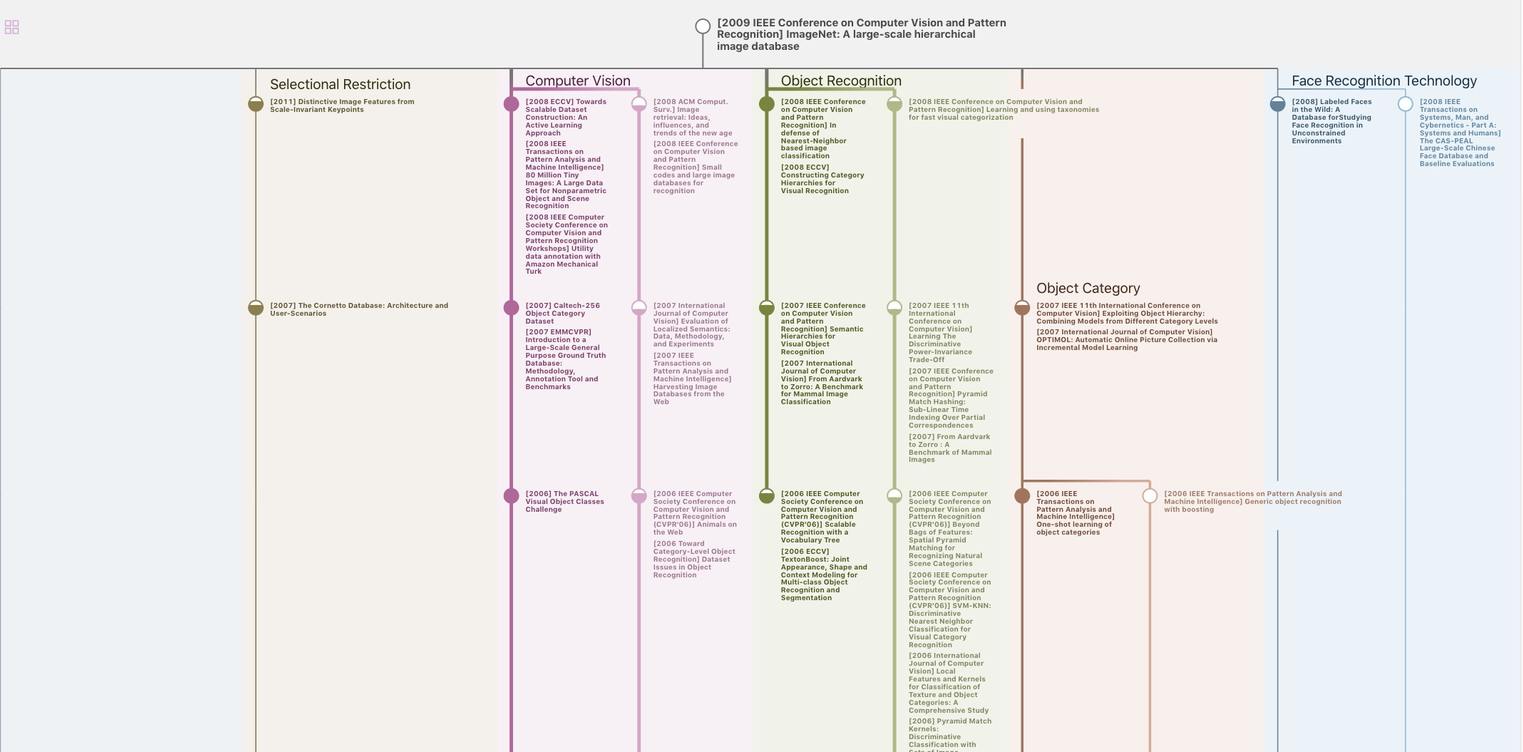
Generate MRT to find the research sequence of this paper
Chat Paper
Summary is being generated by the instructions you defined