Perceptual awareness of natural scenes is limited by higher-level visual features: Evidence from deep neural networks.
Journal of Vision(2022)
摘要
When glancing upon a scene, how much information are observers are aware of? Which particular features and elements of a scene are accessed by conscious awareness? To answer these questions, we used an inattentional blindness paradigm to measure how often observers would notice unexpected alterations to the periphery of natural scenes (i.e., scrambling the periphery so much that no object can be identified, putting the periphery through a low-pass filter, etc.). Observers from Amazon’s Mechanical Turk (N=1,260) were shown flashing images of different scenes (i.e., 288 ms/item) when unbeknownst to them, the final scene was altered in one of 21 ways. Across these 21 conditions, there were drastic differences in how often observers noticed these alterations (e.g., observers often noticed when the periphery was phase scrambled but rarely noticed when the periphery was rotated 90°). Interpreting this behavioral data on its own is challenging since the alterations made to the periphery varied along so many different dimensions. To gain insight into which features are critical for perceptual awareness of natural scenes, we screened a wide range of convolutional neural network architectures (e.g., VGG-16, ResNet-50, etc.) and asked which particular layers of each network best predict the behavioral data. For each network, we computed a direct linear mapping between each layer and our behavioral measurements. We then tested the accuracy of this model at predicting behavioral responses to held-out data (i.e., cross-validated). Across 7 network architectures, we found that mid- to late- layers could predict how frequently observers noticed different alterations to an image with extremely high accuracy: r=0.73 with a behavioral noise ceiling of r=0.78. These results suggest that higher-level visual features play a crucial role in determining the bandwidth of perception and highlight the ways in which deep learning can be used to understand the limits of perceptual awareness.
更多查看译文
关键词
perceptual awareness,natural scenes,visual features,higher-level
AI 理解论文
溯源树
样例
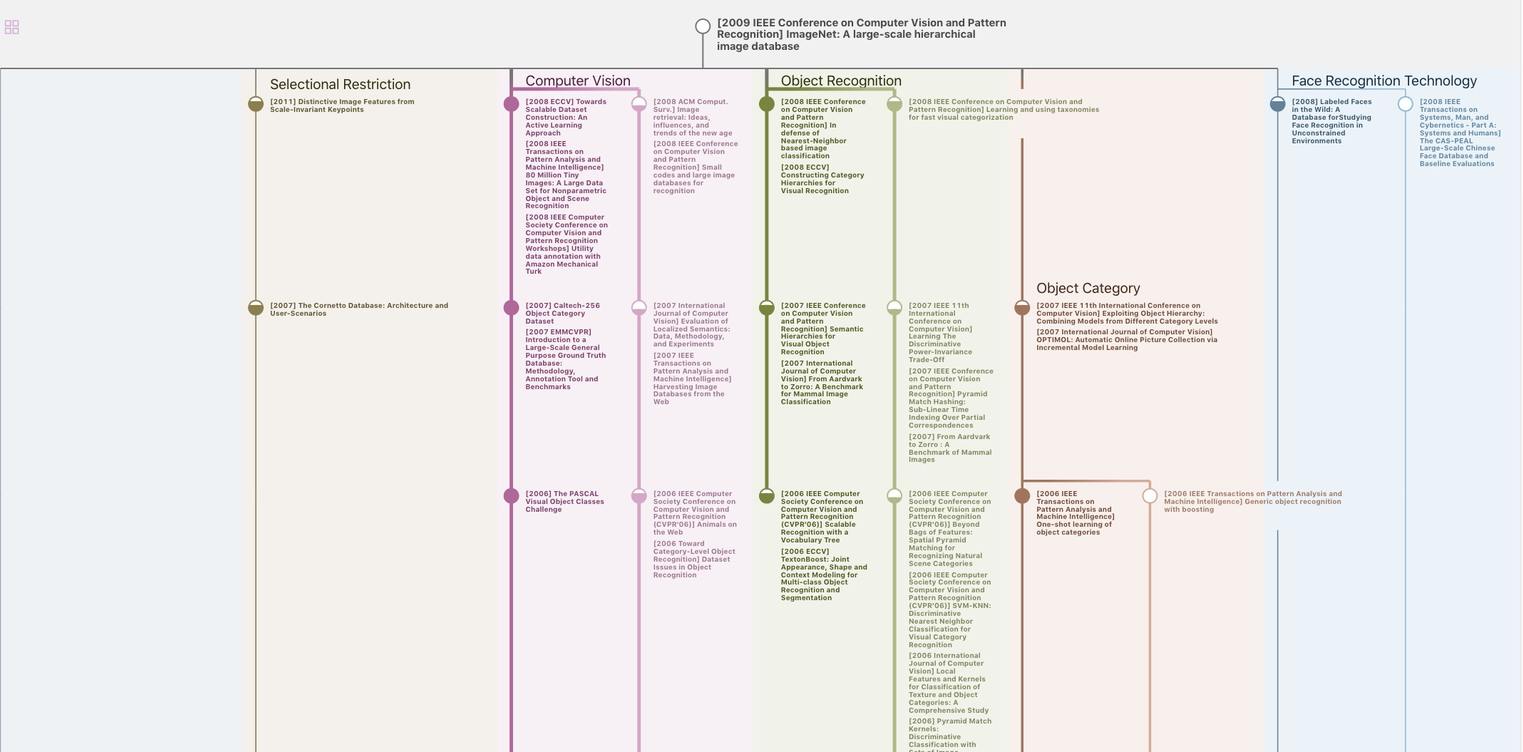
生成溯源树,研究论文发展脉络
Chat Paper
正在生成论文摘要