Hierarchical Bayesian modeling of training accuracy and feedback interaction in perceptual learning in a between-subject design
Journal of Vision(2022)
摘要
Perceptual learning in Gabor orientation identification occurred in low accuracy training only with feedback (Liu et al., 2010) or in combination with high accuracy training (Liu et al., 2012). In this study, we developed a single hierarchical Bayesian model (HBM) to model the trial-by-trial learning curves in all six conditions (training at high, low, and mixed high-low accuracies with and without feedback) in both studies. The four-level between-subject design HBM consisted of parameters and hyperparameters of the learning curves as well as their covariances at the population, condition, subject and test levels. The learning curves were modeled as exponential functions with three parameters: initial contrast threshold, time constant (TC), and asymptotic contrast threshold. We computed the distributions of the learned threshold reduction (M), effect size (d'=M/SD), and TC in each condition based on the hyperparameter distributions at the condition level. Based on the 95% confidence interval of the d' distributions, we found significant learning in the high accuracy with (M: 0.21±0.02 log10 units; d': 3.9±1.06; TC: 407±53 trials) and without feedback (M: 0.22±0.05 log10 units; d': 1.6±0.52; TC: 511±59 trials), mixed high-low accuracies with (M: 0.17±0.04 log10 units; d': 2.4±0.82; TC: 417±74 trials) and without feedback (M: 0.22±0.04 log10 units; d': 2.5±0.84; TC: 444±73 trials), and low accuracy with feedback (M: 0.18±0.03 log10 units; d': 2.3±0.75; TC: 437±66 trials) conditions, but no significant learning in the low accuracy without feedback (M: 0.04±0.04 log10 units; d': 0.4±0.39) condition. In addition, the learned threshold reduction and time constants were not significantly different among the five conditions with significant learning. The HBM modeled all the trial-by-trial data in both datasets in one unified model, characterizing the general properties of the learning curves across levels simultaneously. The posterior distributions of the hyperparameters can also be used as priors for future experiments.
更多查看译文
关键词
hierarchical bayesian modeling,perceptual learning,feedback interaction,between-subject
AI 理解论文
溯源树
样例
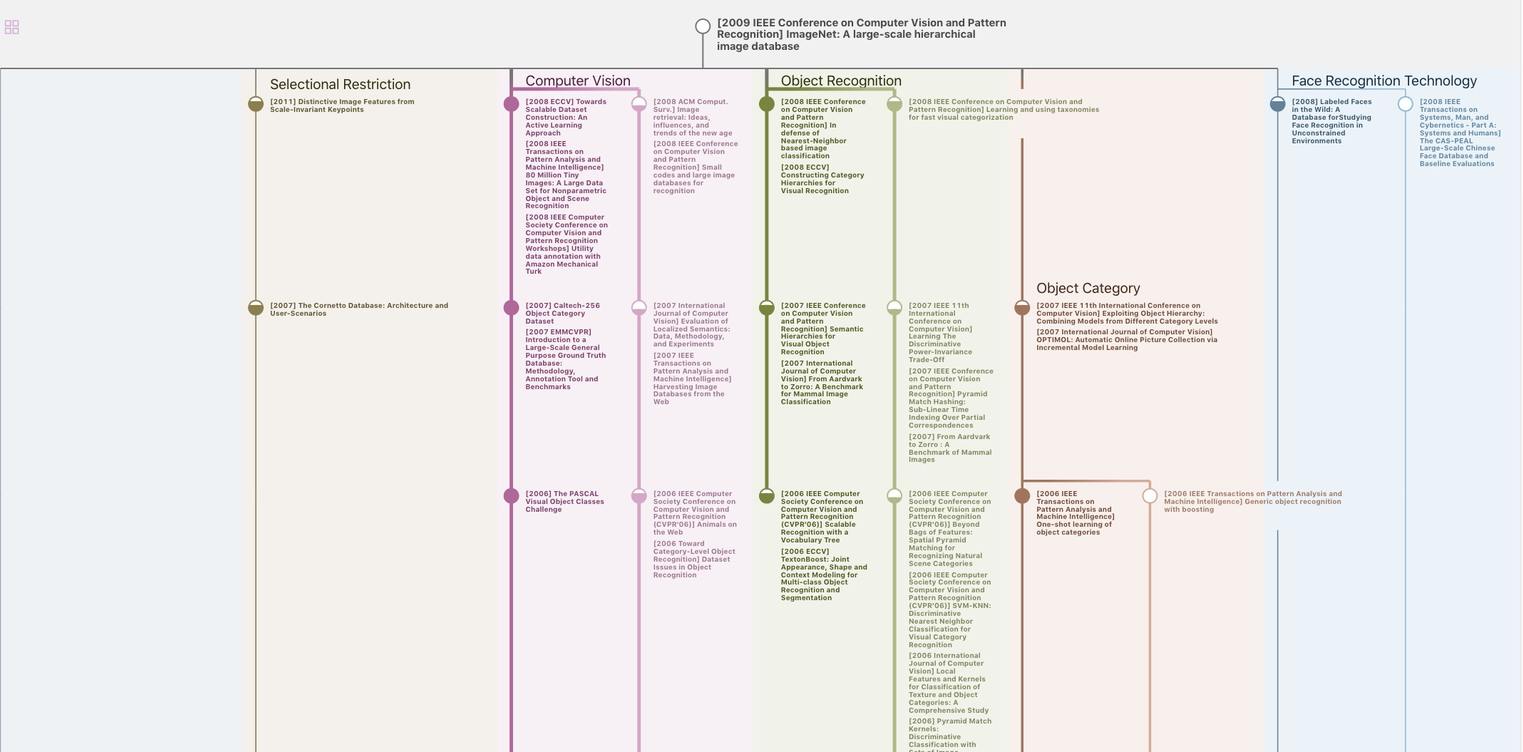
生成溯源树,研究论文发展脉络
Chat Paper
正在生成论文摘要