The nonparametric Box-Cox model for high-dimensional regression analysis
JOURNAL OF ECONOMETRICS(2024)
摘要
The mainstream theory for high -dimensional regression assumes that the underlying true model is a low-dimensional linear regression model. On the other hand, a standard technique in regression analysis, even in the traditional low-dimensional setting, is to employ the Box-Cox transformation for reducing anomalies such as non-additivity and heteroscedasticity in linear regression. In this paper, we propose a new high -dimensional regression method based on a nonparametric Box-Cox model with an unspecified monotone transformation function. Model fitting and computation become much more challenging than the usual penalized regression method, and a two-step method is proposed for the estimation of this model in high -dimensional settings. First, we propose a novel technique called composite probit regression (CPR) and use the folded concave penalized CPR for estimating the regression parameters. The strong oracle property of the estimator is established without knowing the nonparametric transformation function. Next, the nonparametric function is estimated by conducting univariate monotone regression. The computation is done efficiently by using a coordinate-majorization-descent algorithm. Extensive simulation studies show that the proposed method performs well in various settings. Our analysis of the supermarket data demonstrates the superior performance of the proposed method over the standard high -dimensional regression method. (c) 2023 Elsevier B.V. All rights reserved.
更多查看译文
关键词
Box-Cox model,Composite estimation,High-dimensional regression,Nonparametric transformation,Strong oracle property
AI 理解论文
溯源树
样例
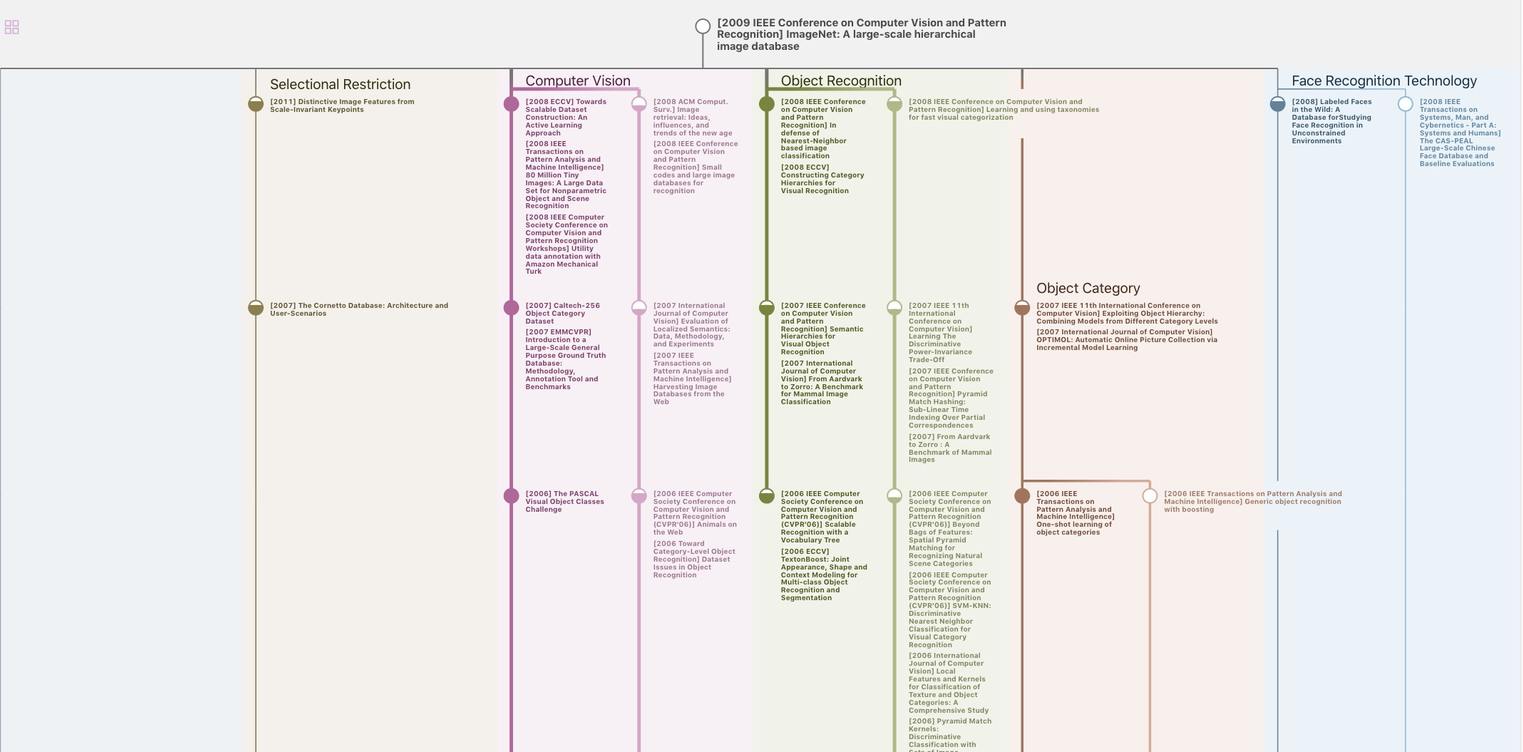
生成溯源树,研究论文发展脉络
Chat Paper
正在生成论文摘要