A Deep Learning Approach of Blood Glucose Predictive Monitoring for Women with Gestational Diabetes
Research Square (Research Square)(2023)
摘要
Abstract Gestational diabetes is a subtype of diabetes that develops during pregnancy.Managing blood glucose (BG) within the healthy physiological range can reduce clinical complications for women with gestational diabetes. In this paper, we developed a Stacked Long and Short-Term Memory (Stacked-LSTM) model to predict before- and after-meal BG levels for women with gestational diabetes. A total of 190396 BG readings from 1110 patients were used for model development, validation and testing under three different prediction schemes: 7 days of BG readings to predict the next 7 or 14 days, and 14 days to predict 14 days. Our results show that the optimized BG schedule uses a 7-day observational window to predict the BG of the next 14 days, achieved accuracies of RMSE = 0.958±0.007, 0.876±0.003, 0.898±0.003, 0.622±0.003, 0.814±0.009, and 0.845±0.005 for the after-breakfast, after-lunch, after-dinner, before-breakfast, before-lunch and before-dinner predictions, respectively. The performance of our models is on par withthe prediction accuracies (RMSE) in benchmark BG prediction models using continuous glucose monitoring (CGM) readings. In conclusion, the Stacked-LSTM model is a promising approach for capturing the patterns in time series data, resulting in accurate predictions of BG levels. Using a deep learning model with routine fingerstick glucose collection is a promising, predictable and low-cost solution for BG monitoring for women with gestational diabetes.
更多查看译文
关键词
blood glucose predictive monitoring,gestational diabetes,deep learning approach
AI 理解论文
溯源树
样例
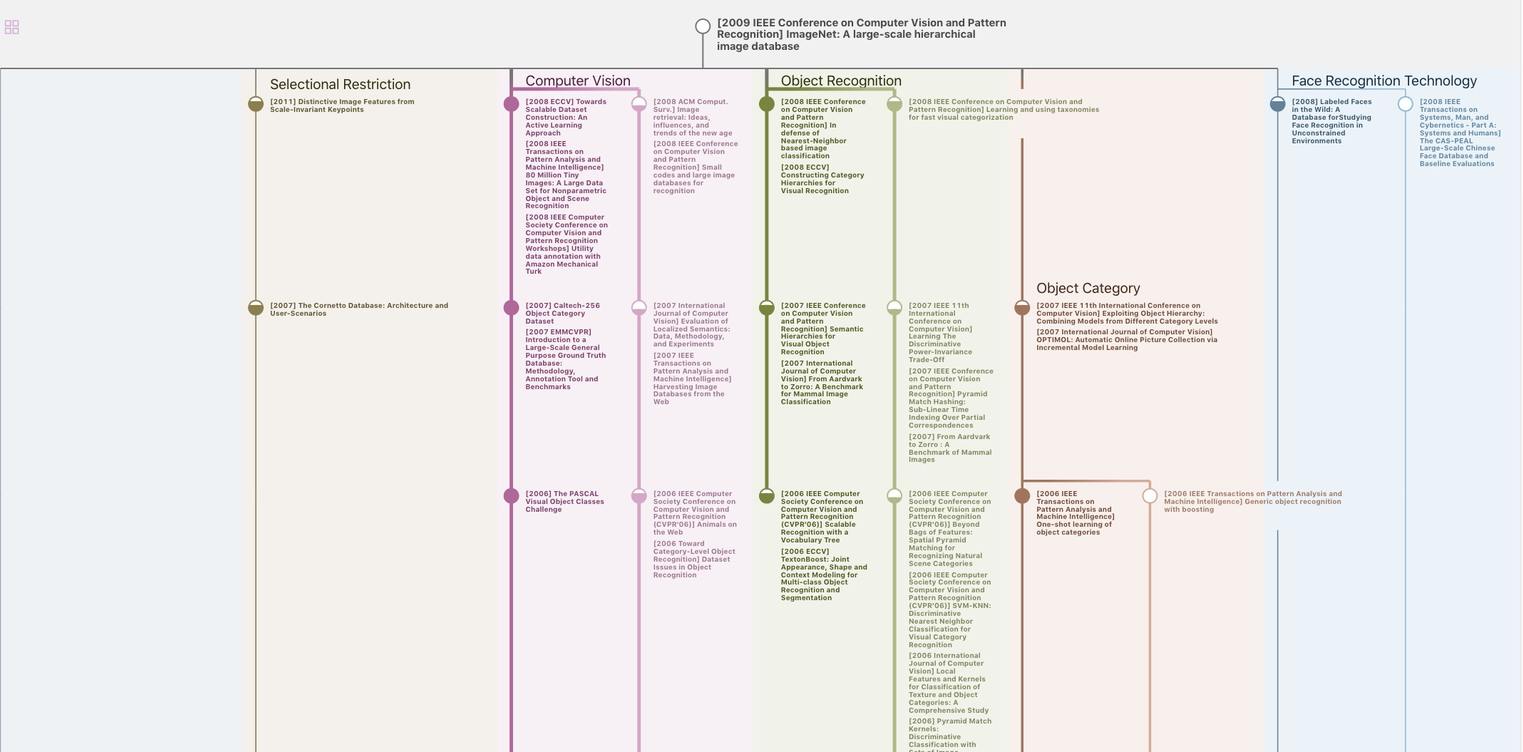
生成溯源树,研究论文发展脉络
Chat Paper
正在生成论文摘要