Big Data Mining on Trace Element Geochemistry of Sphalerite
SSRN Electronic Journal(2023)
摘要
The determination of ore genesis is a main challenge in ore deposit research. Advanced and rapid analytical techniques have given rise to the accumulation of massive amounts of geoscientific data. Therefore, it is imperative to conduct data mining on ore geochemical data to efficiently extract useful metallogenic information. In this contribution, 4095 sets of sphalerite trace element data from 86 deposits of different origins (VMS, MVT, porphyry, epithermal, SEDEX, and skarn) were compiled and analyzed. Factor analysis revealed the effects of physicochemical conditions on sphalerite trace element compositions. Specifically, the high Mn-Fe-Cu-Co-In but low As-Ga-Ge-Sb-Pb concentration of sphalerite is commonly related to decreasing pH or increasing temperature; high sulfur fugacity favors the entry of Fe and Co (but not Ga or Sn) into the sphalerite lattice; and high salinity causes enrichment in Cd-Ga-Ge-Mn, but depletion in Ag-Cu-In-Sb. Multivariate statistical analysis reveals that the Mn-Ge-Sn contents in sphalerite have good potential to differentiate among ore genetic types, the MnGe-In are used to determine between magmatic-hydrothermal and non-magmatic-hydrothermal deposits. Furthermore, machine learning models demonstrate high accuracy of sphalerite trace element data in distinguishing ore types, i.e., 93.02 % (random forest) and 92.82 % (gradient boosting), and its reliability was validated by receiver operating characteristics. Additionally, the blind tests by machine learning on sphalerite trace elements indicate that the Qingshuitang deposit (in the Qin-Hang metallogenic belt, South China), have been an MVT deposit, which is also supported by its low-temperature and high-salinity fluids, wallrock alterations (esp. bariteization), and the obvious age distinction between mineralization and local magmatism. This study highlights that machine learning and multivariate statistical analysis on sphalerite trace-element data can differentiate metallogenic origin and conditions.
更多查看译文
关键词
Big data,Sphalerite,Trace element,Machine learning,Multivariate statistical analysis
AI 理解论文
溯源树
样例
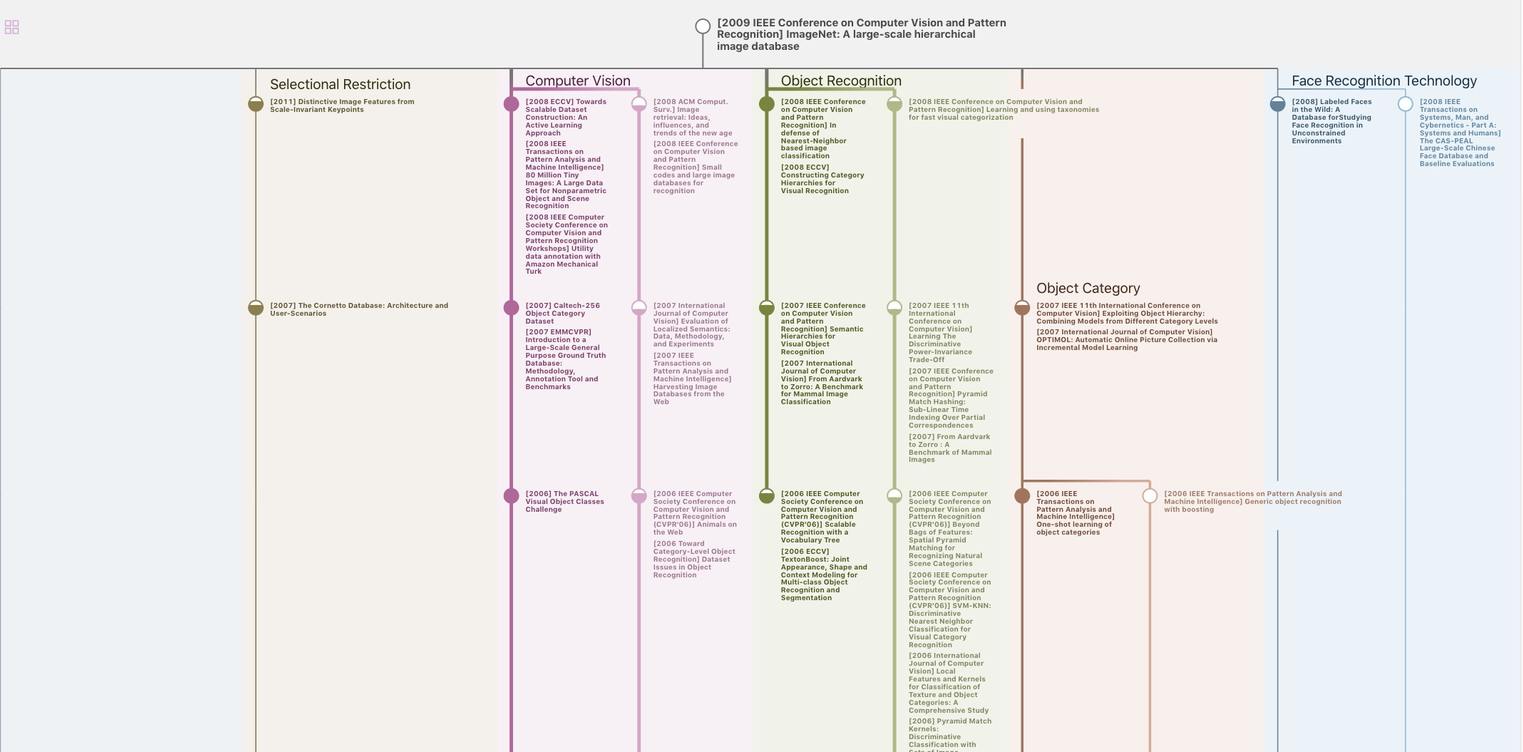
生成溯源树,研究论文发展脉络
Chat Paper
正在生成论文摘要