Data-Driven, Physics-Based, or Both: Fatigue Prediction of Structural Adhesive Joints by Artificial Intelligence
APPLIED MECHANICS(2023)
摘要
Here, a comparative investigation of data-driven, physics-based, and hybrid models for the fatigue lifetime prediction of structural adhesive joints in terms of complexity of implementation, sensitivity to data size, and prediction accuracy is presented. Four data-driven models (DDM) are constructed using extremely randomized trees (ERT), eXtreme gradient boosting (XGB), LightGBM (LGBM) and histogram-based gradient boosting (HGB). The physics-based model (PBM) relies on the Findley's critical plane approach. Two hybrid models (HM) were developed by combining data-driven and physics-based approaches obtained from invariant stresses (HM-I) and Findley's stress (HM-F). A fatigue dataset of 979 data points of four structural adhesives is employed. To assess the sensitivity to data size, the dataset is split into three train/test ratios, namely 70%/30%, 50%/50%, and 30%/70%. Results revealed that DDMs are more accurate, but more sensitive to dataset size compared to the PBM. Among different regressors, the LGBM presented the best performance in terms of accuracy and generalization power. HMs increased the accuracy of predictions, whilst reducing the sensitivity to data size. The HM-I demonstrated that datasets from different sources can be utilized to improve predictions (especially with small datasets). Finally, the HM-I showed the highest accuracy with an improved sensitivity to data size.
更多查看译文
关键词
adhesives,fatigue prediction,artificial intelligence,data-driven models,physics-based model,invariant stress,multiaxial fatigue
AI 理解论文
溯源树
样例
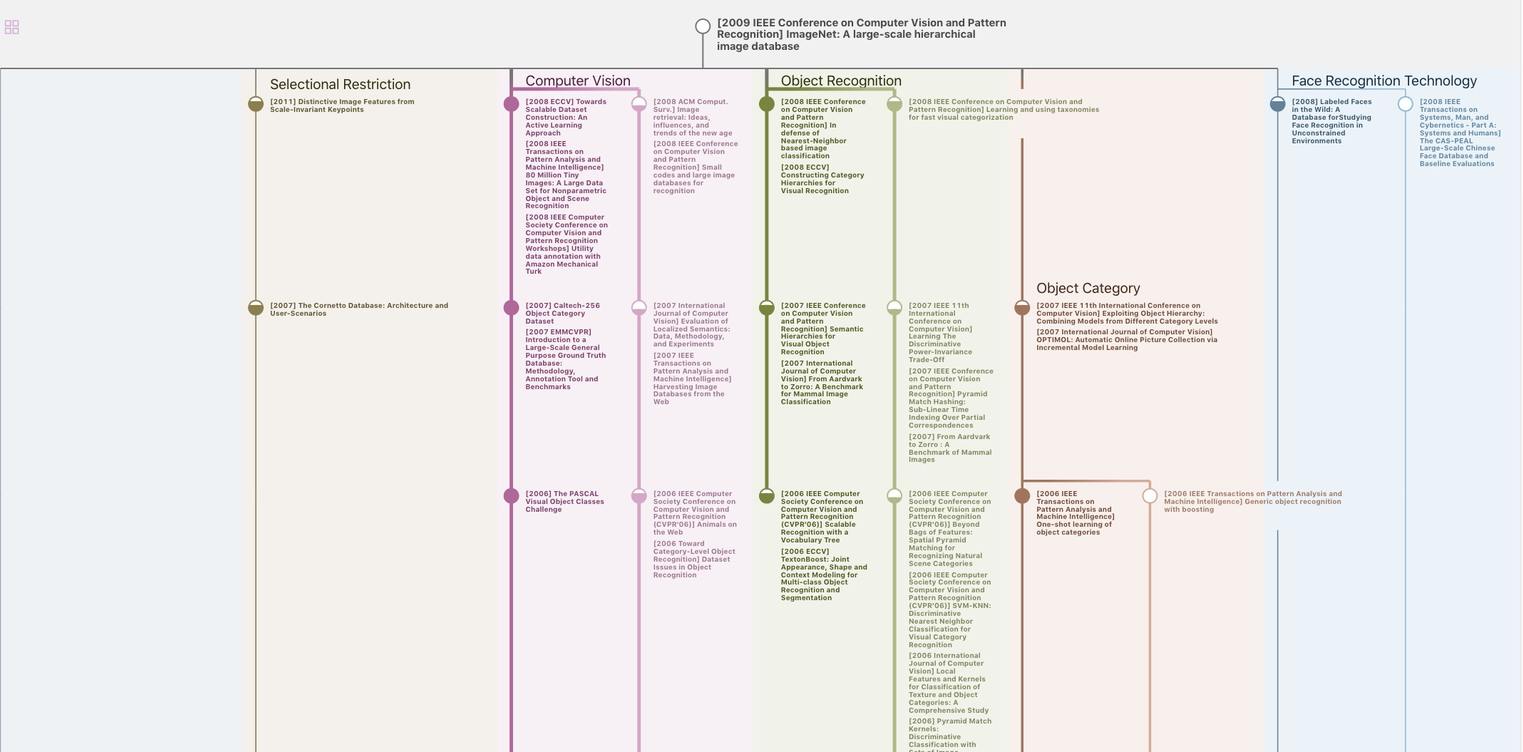
生成溯源树,研究论文发展脉络
Chat Paper
正在生成论文摘要