Adaptive multi-channel Bayesian graph attention network for IoT transaction security
Digital Communications and Networks(2022)
摘要
With the rapid advancement of 5G technology, the Internet of Things (IoT) has entered a new phase of application and is rapidly becoming a significant force in promoting economic development. Due to the vast amounts of data created by numerous 5G IoT devices, the Ethereum platform has become a tool for the storage and sharing of IoT device data, thanks to its open and tamper-resistant characteristics. So, Ethereum account security is necessary for the Internet of Things to grow quickly and improve people's lives. By modeling Ethereum transaction records as a transaction network, the account types are well identified by the Ethereum account classification system established based on Graph Neural Networks (GNNs). This work first investigates the Ethereum transaction network, Surprisingly, experimental metrics reveal that the Ethereum transaction network is neither optimal nor even satisfactory in terms of accurately representing transactions per account. This flaw may significantly impede the classification capability of GNNs, which is mostly governed by their attributes. This work proposes an Adaptive Multi-channel Bayesian Graph Attention Network (AMBGAT) for Ethereum account classification to address this difficulty. AMBGAT uses attention to enhance node features, estimate graph topology structure that conforms to the ground truth, and efficiently extract node features pertinent to downstream tasks. An extensive experiment with actual Ethereum transaction data demonstrates that AMBGAT obtains competitive performance in the classification of Ethereum accounts while accurately anticipating the graph's topology.
更多查看译文
关键词
Internet of things,Graph representation learning,Node classification,Security mechanism
AI 理解论文
溯源树
样例
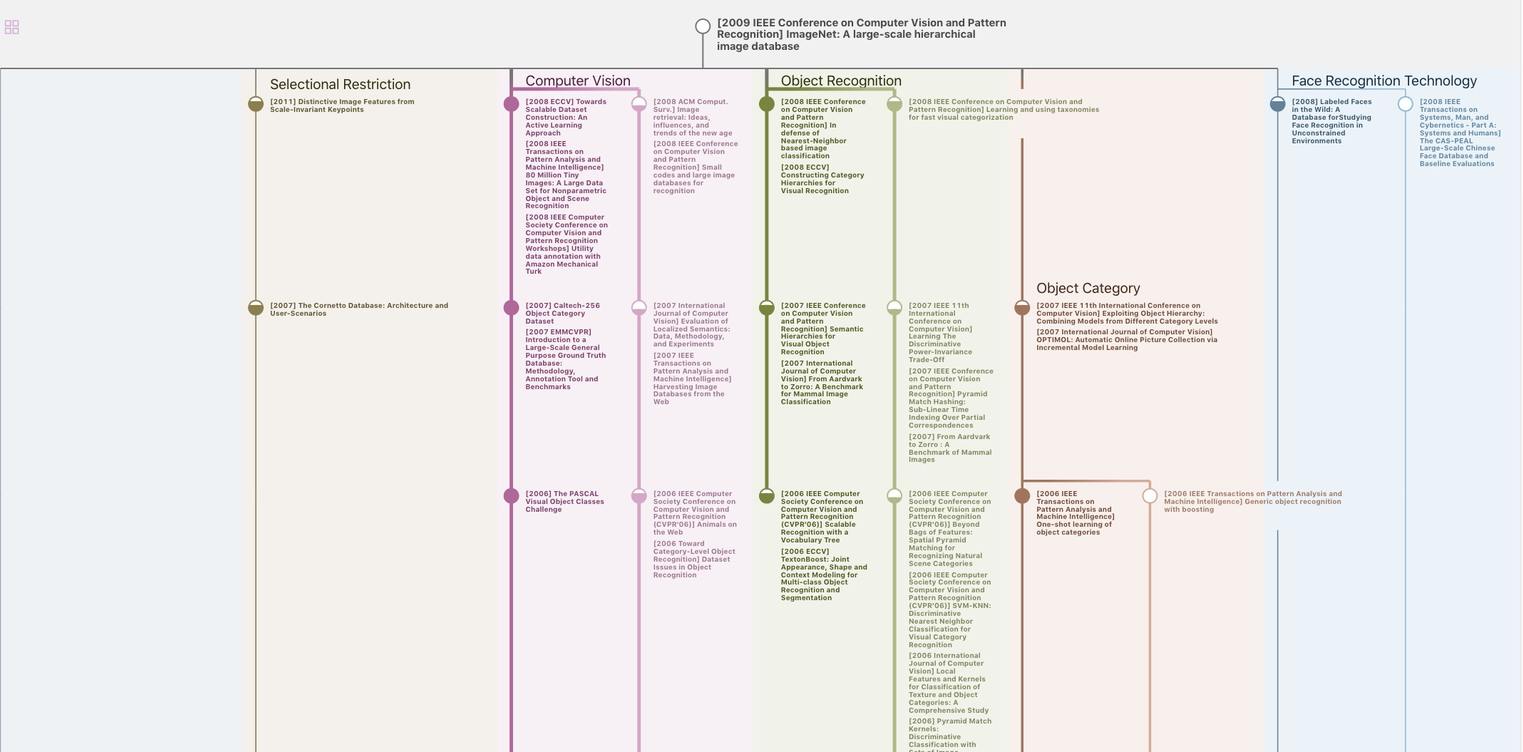
生成溯源树,研究论文发展脉络
Chat Paper
正在生成论文摘要