Water body segmentation in remote sensing images based on multi-scale fusion attention module improved UNet
Chinese Journal of Liquid Crystals and Displays(2023)
Abstract
A multi-scale fusion attention module improved UNet network is proposed for the water body segmentation task of remote sensing images, A-MSFAM-UNet, which achieves end-to-end high-resolution remote sensing images in the GF-2 remote sensing image water body segmentation task. Firstly, aiming at insensitivity of local information caused by global pooling operation of previous attention module, a multi -scale fusion attention module (MSFAM) is designed, which uses point convolution to fuse channel global information and depthwise separable convolution. The loss of information caused by global pooling is made up. MSFAM is adopted to redistribute the weights of feature points after UNet skip connection to improve the efficiency of feature fusion and enhance network ability to obtain information at different scales. Secondly, the atrous convolution is applied to VGG16 backbone network to expand receptive field and aggregate global information without loss of resolution. The results show that A-MSFAM-UNet outperforms other channel attention (SENet, ECANet) improved UNet, and achieves mean intersection over union(MIoU)?mean pixel accruary(MPA) and accuracy(Acc) of 96. 02%, 97. 98% and 99. 26% on the GF-2 water body segmentation dataset.
MoreTranslated text
Key words
remote sensing image, attention module, depthwise separable convolution, feature fusion, atrous convolution
AI Read Science
Must-Reading Tree
Example
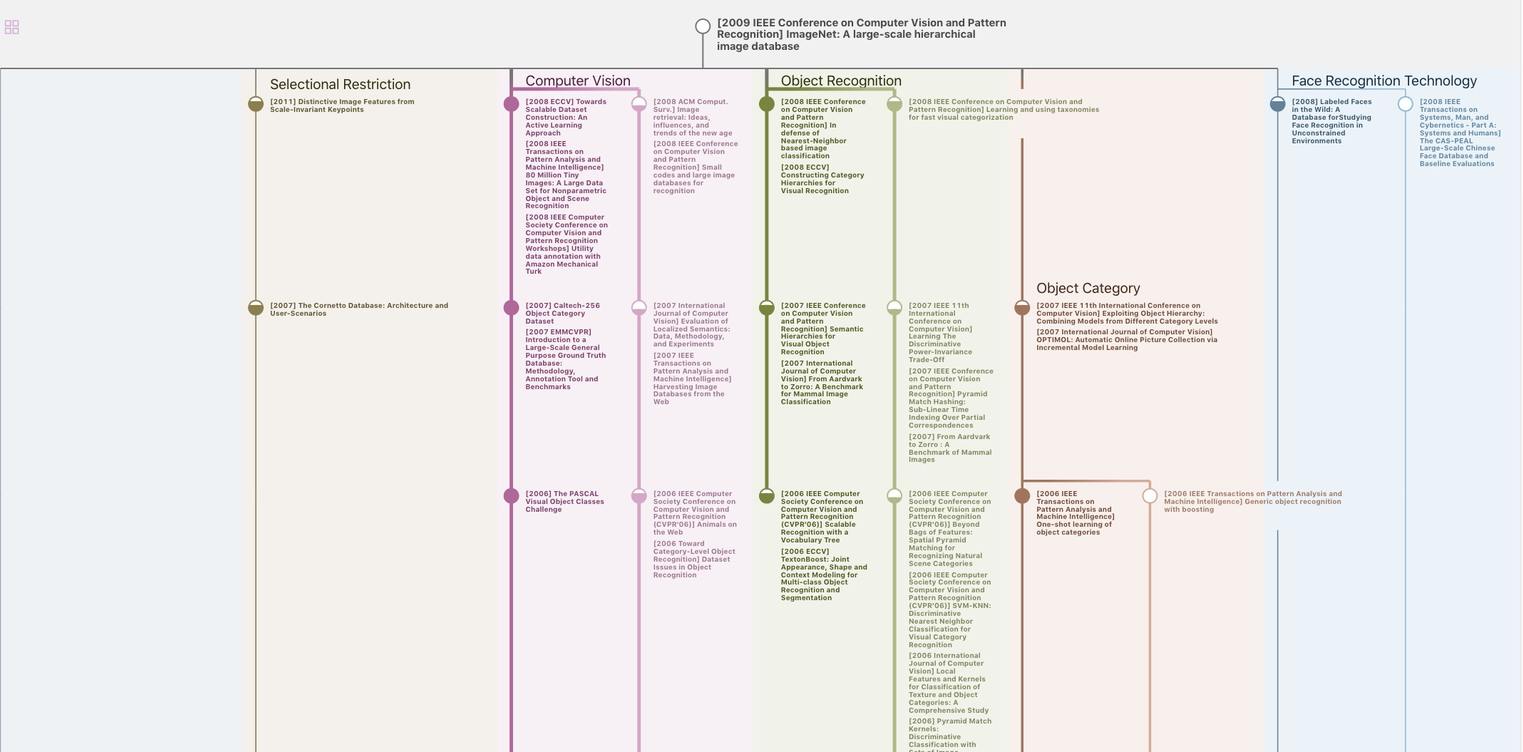
Generate MRT to find the research sequence of this paper
Chat Paper
Summary is being generated by the instructions you defined